MAICing a Decision
Do methods that compare newly approved drugs with those already on the market help cut healthcare costs?
Regulatory approval is seen as a major milestone in drug development, but it’s far from being the final hurdle. After approval, companies must demonstrate the clinical and economic value of their product relative to other existing drugs on the market – so healthcare payers know which drugs to pay for, and how much. A lack of direct comparisons forces analysts to compare results across different studies – often with different study designs and different patient characteristics. And the problem can be especially tricky given that access to the complete patient-level data is usually only available for one study.
With these difficulties in mind, researchers came up with a new method in 2010: matching adjusted indirect comparison (1). MAIC allows researchers to reweight observations or adjust final analyses so that the patient characteristics match the summaries of another trial. In the UK, MAIC has been used in over 20 successful drug reimbursement evaluations and included in methodological guidance for indirect comparisons issued by the National Institute of Clinical Excellence (NICE).
David Cheng, postdoctoral researcher at Harvard’s T.H. Chan School of Public Health is co-author of a new study that looks at the statistical performance of MAIC (2). Here, we speak with him to find out more.
Who came up with MAIC and how does it work?
The development of MAIC was inspired by the real-world challenges faced by decision-makers who need to compare treatments in the absence of head-to-head clinical trials. James Signorovitch, managing principal at Analysis Group, first came up with the idea with colleagues in a 2010 paper published in Pharmacoeconomics. Since then, it has been increasingly applied in health economics and outcomes research studies and health technology assessments.
The method works by making use of individual patient level data (IPD) for one study and adjusting that population to match summary baseline characteristics reported from a published study. Treatment-specific outcomes can then be compared across balanced populations. The key to MAIC is that the analyst does not need access to IPD from both trials – just from one. This is a very common situation in practice, especially right after new drugs are approved and critical reimbursement decisions need to be made by payers. During these periods of time, clinical trial data for new drugs are usually available only to the pharmaceutical company and certain academic groups, and not broadly accessible. Mathematically, the method is similar to propensity score weighting, but uses a different approach to estimate the propensity score model compared to a traditional setting where IPD are available for all patients.
What inspired you to study the statistical performance of MAIC?
The original 2010 paper focused on proposing the method and providing guidance on practical considerations in applications. Few formal evaluations of the procedures have since been reported. The increasing use of the method, in our own applied research and by others, prompted more careful study to better understand under what data scenarios the approach can be expected to deliver reliable comparisons that appropriately adjust for cross-trial differences.
What were your main findings?
Our research identifies the conditions under which MAIC is valid. In particular, it shows that MAIC provides unbiased estimates of a treatment effect when patient characteristics between trials are sufficiently similar and the probability an individual is selected into one trial versus another can be adequately modeled. It also compares bias through extensive simulations to other common approaches to indirect comparisons. Finally, it justifies some approaches for quantifying the uncertainty in the estimates.
What impact could your work have?
Our work helps decision-makers understand when MAIC results are reliable and when there are challenges in the data that would produce unreliable results, such as sample sizes that are too small. And that could, in turn, enable better decision-making and ultimately inform smarter allocation of resources to drugs that work best.
- JE Signorovitch, “Comparative effectiveness without head-to-head trials: a method for matching-adjusted indirect comparisons applied to psoriasis treatment with adalimumab or etanercept”, Pharmacoeconomics, 28, 935-45 (2010). PMID: 20831302.
- D Cheng, “The Statistical Performance of Matching-Adjusted Indirect Comparisons”, (2018). Available at: bit.ly/2KyVCU0. Accessed August 8, 2018.
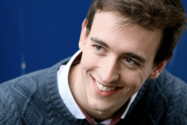
Over the course of my Biomedical Sciences degree it dawned on me that my goal of becoming a scientist didn’t quite mesh with my lack of affinity for lab work. Thinking on my decision to pursue biology rather than English at age 15 – despite an aptitude for the latter – I realized that science writing was a way to combine what I loved with what I was good at.
From there I set out to gather as much freelancing experience as I could, spending 2 years developing scientific content for International Innovation, before completing an MSc in Science Communication. After gaining invaluable experience in supporting the communications efforts of CERN and IN-PART, I joined Texere – where I am focused on producing consistently engaging, cutting-edge and innovative content for our specialist audiences around the world.