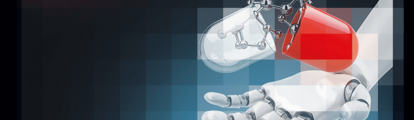
Medicina Ex Machina
The sci-fi inspired concern that artificial intelligence will one day rule the world is (mostly) unfounded, but it certainly could have a huge impact on drug discovery.
Traditionally, pharma companies have been skeptical about the promise of artificial intelligence (AI). But with its potential to bring powerful new tools to the often slow and expensive drug discovery process – and its ability to predict which drugs are most likely to successfully run the clinical trial gauntlet, pharma companies are waking up to the fact that it really could be the future.
In 2017, a collaboration began between Insilico Medicine, an AI company specializing in deep learning for drug discovery, and Juvenescence – a company focused on investing in treatments for age-related diseases and longevity. The aim of the partnership? To find out if AI techniques could enhance drug discovery in the tricky area of age-related diseases, including dementia, diabetes, and cancer, as well as the aging process itself.
In 2018, the companies announced that a handful of promising molecules generated by Insilico’s deep-learned drug discovery engines would be heading into preclinical and clinical development, headed by Juvenescence AI, a joint venture between the two companies. Juvenescence AI is also seeking to independently develop its own AI engine focused on accelerating the clinical development of novel drugs. Here, we speak with Gregory Bailey and Alex Zhavoronkov – CEOs of Juvenescence and Insilico Medicine, respectively – to find out more about the collaboration, and the potential of AI.
What drew you to AI?
Gregory Bailey: Juvenescence is a relatively new company – but we are fortunate to have attracted a team of seasoned drug developers from big pharma and biotech. Having seen millions of compounds sent through high-throughput screening to generate a single lead, our team was captivated by the idea of replacing a time-consuming and wasteful process with AI-driven compound development. The potential to generate lead compounds without high-throughput screening was enough of a draw for us to want to partner with Insilico, but we were even more excited by the prospect of using AI and machine learning techniques to parse through existing genetic and transcriptomic data to identify exciting new targets for drug development.
Alex Zhavoronkov: There is a lot of hype around AI, and most of it is because of recent advances in deep learning and deep reinforcement learning. AI techniques started outperforming humans in many tasks starting in 2014 and 2015 – examples include superhuman image recognition, autonomous driving, and the famous defeat of the human champion of the ancient Chinese board game, Go.
Pharma is perhaps one of the most inefficient industries, with about 90 percent of new drugs failing in clinical trials. The industry spends over $150 billion annually on research and only 40–50 drugs are approved every year. However, pharma companies also generate enormous amounts of data that could be used to train AI systems. We were very impressed by the results of deep learning in images; specifically, Andrej Karpathy’s work on computer vision back in 2014 – and now powering the Tesla’s Autopilot project. He demonstrated how deep neural networks could describe pictures in natural language. Some of these descriptions were better than mine. And when he showed these results at the NVIDIA GTC2015 conference, where Insilico also presented, I decided to invest everything we had into deep learning for drug discovery. I co-founded Insilco Medicine with the ultimate aim of harnessing available data to minimize the need for animal testing and clinical trials by using AI and deep learning to identify new therapeutic compounds and predict biological activity. Back then (this was around 2014), nobody in pharma was using deep learning and it was extremely difficult to explain what we were doing to big companies!
In 2015, I was invited to give a talk in Boston at a closed-door immune-oncology (IO) event organized by a big pharma company, which wanted to understand why it missed the IO revolution and how it should move forward. All of the research executives were there, and for the first time I presented our work in deep learning. No one was interested except for the executive editor of Molecular Pharmaceutics, Carston Wagner, who invited us to submit a paper. It is now an extremely popular paper with an Altmetric score over 750. But the big pharma company did not follow up on the meeting, so I guess they missed the boat in deep learning just like they missed it in IO. Ironically, we do a lot of work in IO with deep learning nowadays!
How does AI work in drug discovery?
AZ: The most difficult part of the application of deep learning to drug discovery is the pace of progress. In deep learning, there are several papers published every week with ideas that can be incorporated into the drug discovery pipeline, and our team is coming up with new ideas on a daily basis. If we were to turn our pipelines into a software product, in a month it would be obsolete. That’s why we keep all stages of our pipeline in a flexible “Lego-like” mode and run the entire pipeline only when we absolutely need to churn out new targets and molecules for partnering and licensing.
The current pipeline starts with deep learned and pathway scoring algorithms applied to massive “omics” databases for target identification. We use generative adversarial networks (GANs) to reconstruct the missing or erroneous values or to generate diverse data sets that we may be lacking. We heavily rely on the predictors of disease state, or in the case of aging, age of the patient, where we extract the most relevant targets and pathways of the disease or aging. We then engage a second part of our pipeline, where we look for the molecules that may effectively inhibit or modulate some of these targets or pathways.
We do this in three ways:
- Screen the existing molecular libraries for molecules that are likely to bind to a specific target.
- Screen for molecules that can effectively reverse a disease pathway signature.
- Generate completely novel molecules that can bind to a specific target using our GANs. GANs are trained on multiple data sets to “imagine” molecular structures that have the characteristics of a good drug. We also introduce a reinforcement learning (RL) component, which helps build the molecules with a specific objective. We have a large zoo of these GAN-RL models and we are building new ones almost every week.
The most promising leads are then scored using a predictor of clinical trial outcome. For disease areas with sufficient data available, we created predictors of success or failure using the “omics” data and the structure of the molecule. The highest-scoring molecules are then synthesized and tested experimentally.
How did this collaboration come about?
AZ: We met the Juvenescence team when Jim Mellon, one of the core founders of Juvenescence, was doing research for his book “Juvenescence” – which discusses the science of longevity. This is where we connected, because we were also interested in developing biomarkers of aging and linking the targets implicated in aging with disease. Jim is often referred to as the “British Warren Buffett” (even though he may not like it!) because he makes some bets very early on, and these bets often turn into multibillion-dollar businesses. Gregory Bailey has a similar track record in the biopharmaceutical industry, so it’s a good combination. We really liked the team and worked very hard to establish this collaboration. Juvenescence invested in Insilico and now has the right to license up to five of the best molecules discovered using our deep learning engine annually.
GB: Jim Mellon introduced Alex to the rest of the Juvenescence team, and we were fascinated by the potential of Insilico’s drug discovery engine to shorten the time between target identification and lead candidate selection. We decided to make an investment into the company and to create a joint venture to develop five drug families per year, playing to our strength in drug development – and Insilico’s strength in drug discovery. This led to the creation of Juvenescence AI, a subsidiary of Juvenescence Limited dedicated to combining advances in AI with our classical techniques, to develop compounds sourced from Insilico Medicine’s drug discovery pipeline and take them through to clinical proof-of-concept.
The team behind our company have an extensive track record in drug development and biotechnology investment, and by using AI to massively reduce the long and expensive drug discovery process we hope to eventually develop drugs to treat both aging itself and diseases of aging including diabetes, dementia, cancer, and respiratory disease.
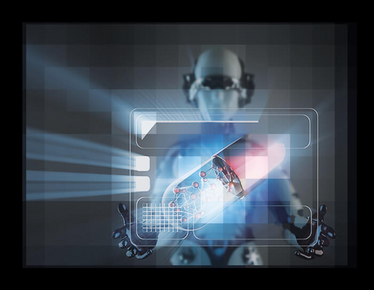
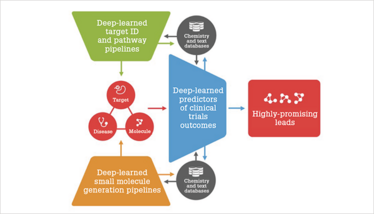
Figure 1. Integrated top-down and bottom-up target ID and drug discovery pipelines.
What advantages does an AI approach offer?
GB: AI approaches to drug discovery offer three major benefits: enabling drug discovery programs against targets for which no high-throughput assay is available, reducing time to lead candidate selection, and identifying new targets of interest. How we apply AI depends on the task; in some situations, it is used to identify a target of interest that can be explored using known biological tools, and in some situations it can generate compounds that are predicted to interact with a given target. Our goal is to build a tight feedback loop between Insilico’s AI engine and the biological experiments conducted based on its output.
AZ: When you really delve into AI and begin to understand how to combine AI tools with advanced machine learning and bioinformatics, you should be able to look at complex diseases and narrow them down to a set of actionable and druggable targets. It can also help us to understand the population-level response and generate new molecules with a specific set of characteristics for the individual targets using GANs and RL. Such generation was not possible just two years ago, as GANs are a very new concept – we were the first to publish and validate the applications of GANs in both medicinal chemistry and biology (1)(2).
I really believe that our AI pipelines are among the most comprehensive in the industry. AI can be used for a top-down approach and can work with multiple omics data types to identify targets for individual diseases, and a bottom-up approach to identify or generate the best molecules for individual or multiple targets. We can then use AI to assess the probability of passing the clinical trials for some of the molecules (see Figure 1).
Why focus on aging?
GB: I think that finding therapeutics for aging and age-related diseases presents us with the opportunity to have the most dramatic effect on the largest population – potentially 7.8 billion people. We are also interested in investments that use AI technology to solve other problems in the pharmaceutical industry, particularly in drug development. AI is a very exciting new tool that gives us the ability to do work that is too time consuming or even impossible for a human. It can also assist us with many of the issues we confront in drug development, such as viable drugs that fail due to a spuriously high placebo effect, or improper patient selection for clinical trials. AI will help drug developers sort out these problems, and therefore have an effect in many different areas.
AZ: I personally have always been fascinated by the idea of extending healthy longevity, but aging is an extremely complex and multifactorial process. As a result, we have taken a wide view of the field, with a variety of projects and collaborators. Aging is also a very important component in many diseases and it is extremely important to study it in both biomarker development and drug discovery, as people of different biological age simply do not respond to therapies in the same way.
We intend to publish many “firsts” this year in multiple areas, ranging from cancer immunotherapy to novel molecular structures generated using AI – and we’ve already started. For example, we were the first to publish a deep-learned predictor of human biological age using simple and inexpensive blood test results. So far we have three papers describing this methodology, and we use it internally on other data types like gene expression and genomic data (3). We were also one of the first to publish on the applications of the adversarial autoencoder (AAE), a form of GAN, in a peer-reviewed journal (2). We submitted in June 2016 and published in December 2016, as it took two months to find the relevant reviewers. In the meantime, another group, led by Alan Aspuru-Guzik from Harvard published the application of the variational autoencoder (VAE) in October 2016. So we can say that we were first to submit, but they were the first to publish. The Aspuru-Guzik group is our main competitor in this area – and a pretty formidable one at that! We have a lot of respect for their team.
What successes have you seen so far?
GB: In terms of drug development, our collaboration has only just begun, and we are in the process of learning about the strengths and limitations of the AI engine. Similarly, Insilico is learning how to interact with the culture of biotech drug development. We have selected a family of geroprotective compounds for development as our first license, and we are also seriously exploring the biology behind four other potential programs.
AZ: So far, we have had many proofs-of-concept, some of which are published. We were also involved in another collaboration in which we applied our AI methods to the development of nutraceuticals, which helped us learn how to work with large population data. We have also had multiple pilots with large pharma companies, and some of these will be published in the near future. But I would consider our real success the partnership with Juvenescence – the successful licensing of our compounds is a major milestone for us! But in pharma, the only outcome that you can truly call a success is the completion of a phase III clinical trial for a blockbuster drug – and we’re still quite far away from that!
What’s next?
GB: Our goal for Juvenescence AI is to build a continually-renewed pipeline of therapeutic candidates. Acknowledging the odds of drug development, we would be delighted if two compounds achieved clinical proof-of-concept in an aging-related disease every year!
AZ: We plan to provide Juvenescence with safe and effective molecules at the early preclinical level that can potentially turn into new drugs targeting both age-related disease and aging itself. Together, we aim to become the core of what will be called the longevity biotechnology industry. The current pharmaceutical model is focused on treatment and, initially, we will need to play by this rule. But in the future, we hope to prevent diseases from occurring, and to help people live longer in a healthier and more youthful state.
How do you think AI will alter the pharma industry as it advances?
GB: Although AI has the potential to open up new landscapes of targets, drugs against these targets will need to fit into the current therapeutic development paradigm. We think the key near-term structural change that AI will bring to drug discovery is a reduced need for the capital-intensive, high-throughput screening process. The collection and collation of more biological data, however, and maturation of machine learning and AI techniques will enable more profound changes to the therapeutics industry, but the structure of the pharma industry is largely a product of its regulatory environment. I hope that as AI techniques prove themselves, regulators will embrace them for drug discovery and development. We assume that for now change will be slow, and we will need to see multiple successes before regulatory processes are updated.
I firmly believe that AI has unique potential to open up new drug targets that humans would not have conceived of, which have the potential to change the therapeutic compendium. AI is an amazing tool; it is not yet a stand-alone solution to all problems in medicine and drug development but certainly it can help humans deal with the extraordinary complexity of biological systems and help us to solve some of the biggest mysteries and challenges in biology.
AZ: Many large pharma companies are still saying that AI is overhyped and we need to slow down. They claim that the AI field is overpromising and will under-deliver, and refer to similar claims made by computational biologists and chemists in the 1990s. Some older-school computational discovery scientists and even some younger academics from the top institutions, who are just entering the deep learning field and want to make a name for themselves, often refer to how easy it is to fool deep neural networks, and how difficult they are to interpret. These people often support each other at conferences and in the press claiming that AI is overhyped and that pharma companies should limit their investments in this field. After the driverless Tesla demonstrations they switched gears and started making arguments for the human-machine union, where deep learning techniques can act as useful tools, while still claiming that AI is overhyped.
My response to these people is simple – AI in drug discovery is not overhyped. In entertainment, robotics and consumer businesses, investors are pouring billions into AI, but this is not the case in the pharma space. Our company struggled to fundraise until 2017, and even after the investment we remain very lean and I do not receive a salary. Many other companies are finding it hard to fundraise as well, and pharmaceutical companies are trying to build internal expertise in this area but failing to attract the right talent. My guess is that in 2016 the total capitalization of the entire AI for drug discovery market was under $300 million with the exception of one company, which managed to get into unicorn territory.
The CEOs of the top pharma companies do not yet see AI impacting their bottom lines, at least on the discovery front. These people are busy with the products they have on the market and with what can be acquired in late-stage clinical trials. But considering the potential, the results we see in the lab and the lag between virtual and real experiments, promoting AI in the pharma space and educating executives on the differences between traditional machine learning and next-generation AI is a very logical thing to do.
I personally think that AI will transform the way we discover drugs, and diagnose and treat diseases. To quote Bill Gates, “We always overestimate the change that will occur in the next two years and underestimate the change that will occur in the next ten. Don’t let yourself be lulled into inaction.” I think we need to act now, and act fast.
Alex Zhavoronkov is CEO of Insilico Medicine, Inc, a company specializing in the development of next-generation artificial intelligence and Blockchain technologies for drug discovery, biomarker development and aging research.
Gregory Bailey is CEO of Juvenescence Limited, an investment company focused on developing therapies for anti-aging and age-related diseases, which will be using AI to augment its drug discovery and drug development programs in partnership with Insilico Medicine.
- A Kadurin et al., “druGAN: an advanced generative adversarial autoencoder model for de novo generation of new molecules with desired molecular properties in silico”, Mol Pharm, 14, 3098–3104 (2017). PMID: 28703000.
- A Kadurin et al., “The cornucopia of meaningful leads: applying deep adversarial autoencoders for new molecule development in oncology”, Oncotarget, 8, 10882–10890 (2017). PMID: 28029644.
- P Mamoshina et al., “Population specific biomarkers of human aging: a big data study using South Korean, Canadian and Eastern European patient populations”, J Gerontol A Biol Sci Med Sci, [Epub ahead of print] (2018). PMID: 29340580.
I have an extensive academic background in the life sciences, having studied forensic biology and human medical genetics in my time at Strathclyde and Glasgow Universities. My research, data presentation and bioinformatics skills plus my ‘wet lab’ experience have been a superb grounding for my role as a Deputy Editor at Texere Publishing. The job allows me to utilize my hard-learned academic skills and experience in my current position within an exciting and contemporary publishing company.