The Three Pillars of AI
AI helps make preclinical decisions faster, but stakeholders remain cautious. We asked an expert from Elsevier for their views on the challenges.
| 4 min read | Interview
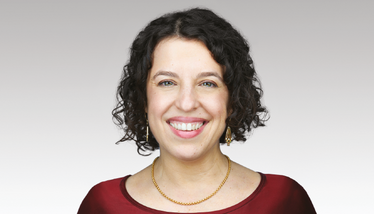
There are three dimensions to AI use in pharma, according to Mirit Eldor, Managing Director, Life Sciences Solutions, Elsevier: the data used to train AI models; the technology itself; and the expertise that goes into these. Elsevier has been working alongside the Pistoia Alliance to address AI-related challenges regarding data, such as the synergy between ontologies and taxonomies, and ensuring that AI has the right context to give the good answers. With AI technology encompassing extractive, predictive, and generative models, the technology requires readiness and maturity to be the right fit for the right problem.
Another challenge related to AI is the human in the loop. With this in mind, we spoke with Eldor about the challenges in AI and how collaborative approaches help.
Is AI deterring people from joining the industry? How will further AI integration affect talent?
Most people are excited about AI, but they are unsure how to use it. Elsevier and the Pistoia Alliance performed extensive market research to figure out where customers want AI, if they're apprehensive of AI, and how we can support them. Most people (between 95 and 97 percent of respondents in our market research) think AI can help them work faster, better, and more efficiently; they believe AI can free up time to work on higher value projects.
However, many people also had concerns. The main concerns were AI leading to misinformation and poor decisions, including critical errors. Our challenge is to help mitigate these concerns. There's no question that AI can be great, but we need to use technology better. The challenge is how to make sure technology works well enough so that we can rely on it. That’s what I see from this research: people want to ensure they can rely on AI so that when you go into a clinical trial, you can make the best decisions on what to take forward and how to design the trial.
What measures has Elsevier implemented to ensure AI applications are safe and fit for purpose?
We started using AI more than a decade ago, so we’ve had time to figure out the risks and how to mitigate them. At the time, there was no legislation, so we had to come up with a set of responsible AI principles that could be applied to every algorithm and every AI-driven product that we build. There are five main elements: the real-world impact of solutions; action to prevent the creation or reinforcement of unfair bias; being able to explain how our solutions work; creating accountability through human oversight; and respecting privacy and championing data governance. This latter includes IP protection, which is particularly important in life sciences. We make sure we have clean, validated data, the right technology, and a human in the loop.
Any data that we use to train algorithms or to give answers has to go through legal governance and checks. People know us mostly as a publisher, but we have comprehensive data sets in the life sciences. We pay royalties for it and we make sure we have the right to use it. We have stringent controls on data management and data privacy.
How do you get involved with policymakers to improve public perceptions of AI in pharma manufacturing or research?
Knowledge sharing is really important for working with other stakeholders and like minded people. Whether we work in pharma innovators, service providers, governments, or NGOs, we all face similar challenges and can learn from each other. In 2022, I was invited to give testimony to the US Chamber of Commerce, which was looking at AI governance and how to mitigate the risks. I'm always happy to work with regulators, industry bodies, and government organizations. Pistoia is a great example. It's a precompetitive collaboration of stakeholders and organizations who struggle with these questions around data, how to bring together data sets, and how to make sure we have data standards. It's a perfect community for sharing with and learning from others.
Are we in safe hands with AI, and is AI in safe hands with us?
Good question! I think we need to continue to think about how to use AI responsibly. In science, we think through the models so that there's no false information. We have to continue to ensure data integrity, and we have to continue to be mindful and cautious as we deploy AI to make critical decisions.
What could AI in the preclinical world look like 10 years from now?
AI will be used extensively to optimize preclinical processes. People currently use it for simple tasks to achieve better productivity, but AI can go much further. I’m really excited. Elsevier has been around since 1840. We have survived by being able to change and adapt over time to numerous technological disruptions that everyone was cautious about. We figured out how to use these technologies to our benefit, from the arrival of the CD-ROM and the Internet, to now with AI. It's natural to be unsure, but technology always makes life easier and more efficient. Yes, we're still figuring it out, but I'm very excited about the difference AI is going to make.