A New Computational Approach to Complex Molecule Synthesis
Early work from Scripps researchers uses a virtual library to synthesize plant-based metabolites
Stephanie Vine | | 8 min read

It was recently reported that researchers from Scripps had used computer modelling to synthesize the picrotoxanes – a class of complex plant metabolite that interacts with the mammalian nervous system. The relevance of their approach to machine learning and artificial intelligence has caught the attention of molecule makers.
Ryan Shenvi’s lab at Scripps Research focuses on fundamental organic chemistry. It’s a basic science approach that leads from method development to total synthesis to in vitro assay to pre-clinical animal studies. Such was the case with Shenvi’s collaboratory work with Professor Laura Bohn on Galbulimima opioids and salvinorins. However, complex molecule synthesis remains slow, circuitous and largely guided by trial-and-error.
The latest study, due to be published in Nature by Shenvi and graduate student Chunyu Li, illustrated a different approach. Here, computational tools were used to define the synthetic route. The authors used both DFT (density functional theory) and statistical modelling to predict which intermediates would react as desired, and which would fail (DFT calculates the electronic structure of molecules, as opposed to using time-consuming quantum mechanical equations for every electron; statistical modelling refers to a broad set of tools that can be used to correlate molecular parameters with reaction outcomes).
We spoke with Shenvi to learn more.
Give us an introduction to picrotoxanes…
The picrotoxanes are a diverse class of plant metabolites that are primarily known for their antagonism of GABAA receptors. These are major neurotransmitter-gated ion channels in the mammalian nervous system, which are also the targets of drug classes such as benzodiazepines and barbiturates.
As the name implies, picrotoxanes tend to be toxic and are named by analogy to picrotoxin, the bitter and toxic principle of Anamirta cocculus. It’s a complicated history; picrotoxin was, at first, thought to be one pure substance, but turned out to be a 1:1 mixture of two compounds, picrotoxinin and picrotin. Picrotoxin, the mixture, was used in early 20th century medicine as an excitant and treatment for barbiturate intoxication. It even found its way into beer as an adulterant to increase “giddiness,” as documented in a two-penny weekly magazine edited by Charles Dickens.
We were interested in exploring picrotoxinin for two reasons: the potential to avoid mammalian GABAA receptors and selectively target their invertebrate homologues; and the potential to selectively modulate GABAergic signaling in certain regions of the mammalian brain – a high risk, high reward goal.
Why are they difficult to synthesize?
The simplest and most superficial answer is that picrotoxanes are structurally complex. The members we synthesized have nine stereocenters, 3-5 rings, polyoxygenation, etc. These are typical metrics used to communicate (or sell the idea) that a molecule is challenging, but it is a superficial analysis. Some molecules are complex but will self-assemble from simple building blocks. Some molecules are complex but synthetically obvious/well-precedented, or closely related to cheap, bulk materials such as carbohydrates or steroids. The more nuanced and truthful answer is that the synthetic burden of a molecule can be hard to determine at the outset – and that is the real story of the paper.
I should also point out that we benefited from existing reconnaissance from the groups of Corey, Trost (with Mike Krische), Yamada and Yoshikishi. This past research helped us anticipate and avoid many pitfalls.
What’s the story behind your virtual library?
We synthesized picrotoxinin in 2020 as a first foray into a potent, well-characterized GABAAR antagonist. We had just synthesized and assigned other plant metabolites as GABAAR antagonists (jiadefenolide, O-debenzoyl tashironin), and also explored known antagonists, such as bilobalide.
However, picrotoxinin gave us the opportunity to investigate a compound with decades of use in cellular assays, tissue samples and whole organisms – even humans. Our 2020 synthesis allowed picrotoxinin to be stabilized against hydrolysis and led postdoc Guanghu Tong to create a small library tuned towards preferential binding of invertebrate GABA (RDL) receptors (a fun collaboration with Zack Buchan and other folks at Corteva Agriscience). We also recharacterized how picrotoxinin underwent hydrolysis in buffer and explained why other congeners (such as tutin, another picrotoxane) showed greater stability.
However, when we tried to adapt our existing synthesis to tutin, it failed. This was surprising because the synthesis intermediates that failed were so similar to the successful intermediate in our 2020 synthesis.
This scenario is familiar to synthetic chemists – not because chemistry is unpredictable, but because there are too many variables to evaluate simultaneously, even for single elementary steps. Typically, our lab and others have solved this problem with screening, using hundreds of semi-random or empirically-guided experiments that explore changes to reagents or reactant.
This screening approach is tried-and-true. However, it is also wasteful and sometimes intellectually lazy. In our case, the synthetic intermediates were hard to access and the effects leading to failure were non-obvious. However, we benefited from the simplicity of the failed reaction; an attempted 1,5-HAT reaction that instead led to β-scission. It was an ideal scenario to explore a general approach to virtual screening.
What were the biggest challenges?
By far the greatest hurdle was our lab’s lack of experience with DFT calculations. We were starting from square one. Luckily, Chunyu Li picked it up very quickly by way of a Scripps DFT class directed by Professor Keary Engle. We benefited from seminal DFT studies of HAT reactions by Houk and others. One of our lab’s postdocs, Dr. Stephen Ting, a wonderful scientist from Professor Abby Doyle’s lab, also used his experience with coding to develop a tool called autoDFT, which made job submissions much easier for novices.
However, I should also point out that quantum mechanical calculation has recently become more widespread and accessible for beginners. There is a great company, Rowan Scientific, that even offers web-based computing.
But calculations tell you nothing if the output is physically meaningless, or if the questions are unclear. It took us some time to select the right basis sets and tunneling approximations so that our calculations could be benchmarked. That is, we could compare the predicted relative rates from DFT with real ratios of products derived from 1,5-HAT versus β-scission. We needed very little data – just three novel experiments and two from the literature – but assuring fidelity to reality was crucial. Many calculations that appear in the literature lack rigorous benchmarking, or lack any predictions that are probed experimentally. It’s hard to put any actionable confidence in such calculations, even if they make pretty figures.
We modeled 30 virtual synthetic intermediates and triaged them by preference for 1,5-HAT. Finally, we extracted parameters from the DFT library that might predict success via linear regression analysis. This statistical modeling also proved successful without the need for massive experimental data. We needed just the 5 real data points to benchmark the DFT model, plus the 30 virtual experiments. Furthermore, the predictive parameters helped us understand the mechanism for how reactivity was relayed from one site in the molecule to another, which was a nice bonus.
What problems could this approach potentially solve?
I see this approach as part of a larger effort by the community to better integrate computational tools into the chaotic, empirically-driven world of organic synthesis. Established groups such as those of Jacobsen, Sigman, Doyle and Denmark are training legions of talented students in this area. At the same time, young labs led by people like Tim Cernak, Ana Bahamonde, Jolene Reid and many others are doing great work. It’s an exciting time to work at the interface of synthesis and computation.
In some ways, the paper served as a critique (a constructive critique, I hope!), of retrosynthesis tools, which are driven by pattern recognition that is too coarse to be predictive (unless the targets and intermediates cleave very close to literature precedent). These tools might aid the design of overall strategy, but questions of feasibility remain unanswered. Feasibility, of course, determines strategy, so there must be crosstalk between the two.
Our paper demonstrates one way to create this crosstalk computationally. We did not use AI, which is far from being applicable to this sort of problem. But the statistical modeling we did use (linear regression analysis) and the way we used it could be incorporated into machine learning for total synthesis.
It’s an interesting thought experiment that is far away from automated deployment, but this paper establishes the general idea and proof-of-concept.
What are the next stages?
We have a few ongoing projects that have benefited from this approach already. For example, we used computation to triage a virtual library of linkers for tethered glycosylation. This approach led to the cotylenins, a series of glycosylated 14-3-3 protein/client molecular glues. We have ongoing work on antifungal and neuroactive compounds that also rely on DFT analysis.
I think the biggest hurdle will be generalizing this tool using existing literature data – not because it is impossible, but because of the person hours involved. I can imagine building benchmarked DFT models that are then parameterized to be predictive across diverse substrates, but it would take a tremendous, community-wide effort and centralized organization like CAS. For the time being, we’re doing this in-house on a project-by-project basis.
It’s early stage for the work, but potentially how could it be applied to drug discovery and development?
I’ve fielded a couple meetings to find out.
Industrial discovery and process development for many years have been working on computational route design, route derisking, reaction modeling and route triage. We are new-comers to this arena, but there are great opportunities in working together.
This paper is a proof-of-principle and I’m proud of its rigor, but it relies on extensive computational work by others in the community. In many ways, this paper is merely a representative of a field on the verge of explosive growth. I’m very happy to be part of it, but we owe so much to so many.
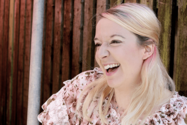
Making great scientific magazines isn’t just about delivering knowledge and high quality content; it’s also about packaging these in the right words to ensure that someone is truly inspired by a topic. My passion is ensuring that our authors’ expertise is presented as a seamless and enjoyable reading experience, whether in print, in digital or on social media. I’ve spent fourteen years writing and editing features for scientific and manufacturing publications, and in making this content engaging and accessible without sacrificing its scientific integrity. There is nothing better than a magazine with great content that feels great to read.