AI: Hype or High Performance?
Generative chemistry and other exciting applications of artificial intelligence in small molecule drug discovery
Michael Parker | | 4 min read | Technology
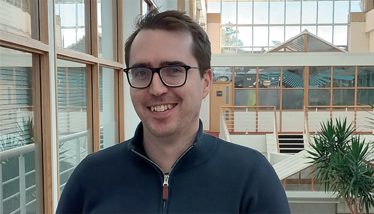
Depending on who you speak to, AI will either save or destroy the world. Rhetoric around AI making jobs obsolete remains, but perceptions are also now shifting towards embracing the usefulness of AI. Exciting tools, such as DeepMind’s AlphaFold protein structure prediction software, regularly make headline news, and there is a growing realization of the potential time, money, and resource savings that could be achieved by adopting AI in drug discovery. Reducing the number of required experiments, screening larger databases than ever before, streamlining workflows, idea generation, and synthesis predictions are just a few examples of the benefits of AI.
When it comes to synthesis prediction, AI has made great strides due to the sheer volume of published literature now available for it to trawl through. However, the intricacies of identifying a feasible synthetic route can be tricky for current AIs to predict. Reagents, reaction parameters, and multi-step syntheses lead to a complex matrix of factors to consider. In recent years, however, new retrosynthesis software has developed to allow for more accurate synthesis planning.
Sparking ideas through generative chemistry
Exploring the vastness of chemical space to find active compounds with suitable pharmacokinetic properties is challenging. Early attempts at generative chemistry software tended to provide poor or mixed quality suggestions of unstable, synthetically complex, or inaccessible molecules. These previous classical models focused on iteratively applying medicinal chemistry transformations to eventually get to a new, better molecule.
The dawn of AI in this field saw auto-encoders as a popular machine learning approach to improve the span of chemical space and the quality of suggestions provided by the software. However, more recently the community has started turning towards transformer models. These AI models are the foundations of large language models (LLMs), such as ChatGPT. They are faster, more powerful, and cheaper to train than other model types – and they work with bigger data sets. Harnessing transformers will enable drug discovery scientists to explore more chemical space. Taking a forward-looking perspective, it seems clear to me that these types of models will become more prevalent, supporting drug discovery scientists to ideate a wider variety of chemical structures, with better confidence in their synthetic accessibility.
Whilst considering compound ideas, scientists also need to identify those with sensible property profiles that fit their specific project. Multi-parameter optimization across complex absorption, distribution, metabolism, excretion, and toxicity properties can be difficult, with most AI platforms struggling to provide suggestions for previously unseen compounds or across the necessary numbers of endpoints. This area has huge potential. Gaining pharmacokinetic data experimentally can be very time-consuming and resource intensive. Often, this experimental data is “noisy,” with errors and outliers, and “sparse,” because of the difficulty in collecting data for all the areas a scientist may be interested in for every compound.
Cutting-edge deep learning algorithms can be used to impute missing data alongside their uncertainties, to highlight compounds with the highest chance of success and best potential property profiles. This approach has huge potential for streamlining innovation, reducing the number of necessary experiments, and guiding experiment prioritization to increase the efficiency of the drug discovery pipeline.
Bringing it all together
Individually, all these areas will undoubtedly progress in the coming years. What is incredibly exciting is the possibility of AI carrying out full design-make-test cycles using in-built reasoning processes. Indeed, the first very simple examples have recently been shared on ArXiv (an open access repository of articles) by a group of researchers from the Laboratory of Artificial Chemical Intelligence, National Centre of Competence in Research Catalysis, and the University of Rochester, UK, using an LLM to plan and carry out the synthesis of some simple small molecules. Giving LLMs access to the relevant tools for tasks such as simple data analysis may free up time for scientists to carry out more detailed, in-depth, or creative tasks.
There is certainly a great deal of promise for AI in drug discovery, but there are also areas that need to be improved. Data standardization can enable us to build larger interoperable data sets for AI to train on, improving models and helping build the necessary supporting computing infrastructures. For example, a general trend towards cloud-computing architectures will enable resource scalability.
Increasing trust in the reliability of AI tools will only come with time and evidence. Open science and an increasing number of publications will support this (as will educating potential users on how these methods work) and provide clarity on the strengths and weaknesses of different AI methods in particular scenarios.
Increasing ease-of-use by embedding AI tools within intuitive workflows and visual interfaces will also support adoption. Allowing users to access AI as part of an integrated in silico development pipeline containing all the tools and data they need could have significant impacts on efficiency. Each update, coupled with the continuous improvements in AI methodologies themselves, could have a revolutionary impact on the efficiency of drug discovery pipelines.
Michael Parker is Principal AI Scientist at Optibrium. He obtained his PhD in Astrophysics from the University of Cambridge, researching X-ray emission from accreting black holes. Prior to Optibrium, he held previous postdoctoral positions at the University of Cambridge, UK, and a research fellowship at the European Space Agency, all focused on the use and development of data science tools to analyze complex noisy datasets.