Meet an AI Model that Predicts Teratogenicity
How complex data analysis paired with artificial intelligence can help reveal additional causes of birth defects
Jamie Irvine | | 3 min read | News
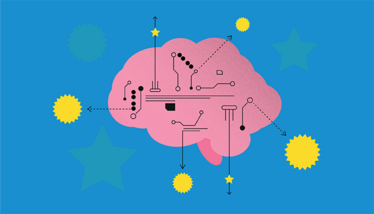
Functional and structural birth defects affect almost one in 33 births in the US. They are often caused by genetic factors, but can also be triggered by external variables, such as drugs, cosmetics, food, and pollutants that women are exposed to during pregnancy. In many cases, however, the exact cause of the birth defect is unknown.
When it comes to pharmaceuticals, predicting teratogenicity is challenging. In some cases, we can know that certain drug classes are likely to affect DNA and cell division. In others, the potential risks to the fetus are unknown. And though drugs can be tested in animal models to understand if they cross the placental barrier, the findings may or may not translate to humans.
Focusing on the impact of pharmaceuticals, scientists from the Icahn School of Medicine at Mount Sinai in New York have created an artificial intelligence (AI) model that can predict which existing medicines – not currently classified as harmful – could lead to congenital disabilities.
First described in the Nature Journal Communications Medicine, the model – or “knowledge graph” – also has the potential to predict the involvement of pre-clinical compounds that may harm a developing fetus.
“Knowledge graphs are networks of connected entities used to organize and combine data in a way that emphasizes – and naturally captures – the relationships between different types of objects,” says Avi Ma’ayan, Professor of Pharmacological Sciences and Director of the Mount Sinai Center for Bioinformatics at Icahn Mount Sinai, and senior author of the paper. “Knowledge graph databases are more efficient in conducting complex searches, and their network representation is highly useful for imputing knowledge with machine learning.”
To train their AI system (ReproTox-KG), the researchers collated knowledge across several datasets with birth-defect associations in published work, including genetic associations, drug-induced gene expression changes in cell lines, known drug targets, genetic burden scores for human genes, and the ability of small molecule drugs to cross the placenta. They then demonstrated how integrating data from these resources can lead to discoveries. AI ranked more than 30,000 preclinical small molecules for their potential to cause birth defects, as well as identified over 500 different “cliques” in the knowledge that connect birth defects, genes, and drugs potentially explaining molecular mechanisms.
“Although identifying the underlying causes is a complicated task, we offer hope that, through complex data analysis like this that integrates evidence from multiple sources, we will be able, in some cases, to better predict, regulate, and protect against the significant harm that congenital disabilities could cause,” says Ma’ayan.
There is potential to expand the model even further, Ma’ayan adds: “For example, we can consider the tissues and cell types that are most affected, the period of time in development that is most relevant, add additional information about the drugs, include information about viral and bacterial infections, and conduct the predictions directly on the knowledge graph using graph-based learning algorithms. Importantly, we started working with some collaborators who are interested in testing some of the predictions in animal models”.
The team concluded that drug developers should profile their compounds in cell lines to produce a signature of the genes that the new drug induces and represses. Such signatures could then be compared with other drugs to assess mechanisms of action, potential for side effects and adverse events, as well as uses/indications. This approach presents an opportunity to collaboratively build a gene expression drug response database to assess toxicity of preclinical drugs
This work originated from a Common Fund Data Ecosystem (CFDE) partnership. The CFDE is a new NIH Common Fund program that aims to enhance the accessibility of datasets produced by Common Fund programs and combine this data for synergistic discoveries.
The Common Fund program Kids First tackles the many birth defects that cannot be explained using genetics. At the same time, the Common Fund program LINCS collected rich information about the effect of approved drugs and an additional set of 30,000 compounds on human cells. The study combines data from these two programs and other sources
Associate Editor, The Medicine Maker