AI Versus AMR
MIT scientists studying AI computer programs’ ability to identify drug mechanisms believe the future is bright for antibiotics discovery, but more work needs to be done
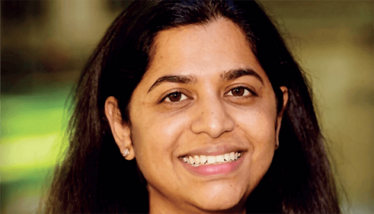

Known for being expensive and not particularly efficient, current methods for screening drug mechanisms of action have not performed well when it comes to the search for new antibiotics. Felix Wong and Aarti Krishnan, postdoctoral fellows in the Jim Collins lab at MIT and members of the Broad Institute of MIT and Harvard, hope to address that with a new study focusing on computer models’ ability to identify drug mechanisms of action. Here, Wong and Krishnan discuss AlphaFold, a promising AI program they have used to accurately predict the behavior of bacterial proteins in interaction with antibacterial compounds. But is AlphaFold ready for the big leagues?
Why is AMR still such a key topic?
Global deaths due to drug-resistant bacterial infections are projected to reach 10 million per year by 2050 (1), almost twice the reported number of global COVID-19 deaths to date. The increased prevalence of AMR also means that there will be increased morbidity for even routine procedures such as surgeries and hospital care.
The lack of new antibiotics has been a longstanding challenge. How pressing is the need for innovation in this area?
It took 38 years for us to introduce a new class of antibiotics to the clinic – the oxazolidinones in 2000 – after the 1962 introduction of quinolones (2). There has been no shortage of innovation in antibiotic discovery, but finding clinically relevant antibiotics is hard. The major classes we discovered in the middle of the 20th century (which are still in use today) came from empirical screens of natural products, notably from soil bacteria. Now, this pipeline has dried up and many of our efforts to invent new approaches have yielded molecules that are toxic to humans or for which resistance could evolve easily. One way forward may be to vastly augment the chemical libraries we are exploring to better sample more aspects of chemical space (3).
Why aren’t many companies developing new antibiotics?
A major reason that companies hesitate to invest in new antibiotics is that bringing these drugs to market is generally not profitable. There is no financial incentive for a money-losing business. Governments, academia, and industry must take immediate action so that we can discover novel antibiotics against killer superbugs. A typical R&D cost for an antibiotic can be US$1.5 billion, but the revenue it generates is only about $46 million per year (4). This situation is partly due to the possibility that not many people might need that specific antibiotic and partly to the fact that the price one can charge for treatment is usually limited by government regulations.
Is the development of new antibiotics considered scientifically difficult?
Definitely. Finding chemical compounds that kill bacteria is not hard, but finding those that kill bacteria without being toxic to humans – and have enough favorable medicinal chemistry properties to inspire further testing – is rare. On top of this, bacteria might quickly evolve resistance to the compounds of interest and render them useless.
It wasn’t always this difficult. The “golden age” of antibiotic discovery in the mid-20th century saw many chemical screens yield new, selective, and effective antibiotics. The problem now is that we’ve picked much of this low-hanging fruit. In the meantime, bacteria have evolved resistance. Our current chemical screens don’t yield nearly enough lead compounds, which may be due to the fact that we can only explore so much chemical space. Developing new computational screening pipelines is one approach that could help us navigate chemical space and discover new lead compounds.
How are in silico drug discovery tools helping?
We now have computational approaches for virtual screening, so we can quickly and cheaply predict antibiotic activity from the chemical spaces of billions of compounds. We can go through these in weeks and use our models to prioritize which molecules we procure and test in the lab. The Collins lab pioneered this type of approach and it resulted in the discovery of a new antibiotic candidate, halicin, two years ago (5).
Predicting antibiotic activity is a coarse-grained approach, though. Toxic compounds often have antibiotic activity (which the model would recognize), but they don’t make very good drugs. In our study, we wanted to go a step further and predict drug binding targets. This means that we could, in principle, predict how exactly an antibacterial compound works and whether or not its mechanism of action might have toxic liabilities. If our in silicoapproaches could successfully do this, we could more easily pick out real antibiotics from large chemical spaces, characterize how they select against bacteria, and maybe even design antibiotics de novo.
How does AlphaFold help – and what are its limitations?
AlphaFold is an AI system developed by DeepMind that uses the amino acid sequence from a protein to give us a three-dimensional structure. It can provide excellent predictions of the 3D structures of many proteins; those structures are freely available to the scientific community and can be used for molecular docking simulations – an in silicoapproach similar to assembling a jigsaw puzzle. This allows us to predict how a compound targets bacteria by simulating whether or not it binds to a specific protein of interest. Many antibiotics work in this way; for instance, quinolones bind specifically to bacterial DNA gyrase and topoisomerase, whereas β-lactams bind specifically to bacterial penicillin-binding proteins.
In our research, we aimed to perform this sort of prediction at scale. We looked at the interactions between 296 proteins from Escherichia coli and 218 antibacterial compounds, or ligands (6). By simulating each of the 64,310 pairwise protein-ligand interactions using molecular docking on the corresponding AlphaFold-predicted protein structures, we could predict which binding interactions were likely and which were unlikely. We then performed experiments at the bench for 12 different proteins, empirically testing them for binding activity with respect to each of the 218 antibacterial compounds. After comparing our model predictions with our experimental results, we found that the model performed no better than chance; it correctly predicted a real interaction only about half of the time. Thus, one of the main takeaways of our study is that molecular docking needs to be improved so that we can correctly predict binding interactions and better leverage AlphaFold for antibiotic discovery. One known limitation of AlphaFold is that it predicts only static, rigid protein structures that are “stuck” in time, but the dynamic and disordered properties of these structures could be important for drug binding.
How can this issue be overcome?
It’s likely that we need more accurate ways of simulating the interactions between proteins and ligands. Indeed, when we used machine learning-based models trained on additional binding information to complement the baseline docking approach, we found that the predictive accuracy increased. It’s also likely that improvements in protein structures might enable us to better predict drug binding.
We think AlphaFold will be a useful resource for the drug discovery community. However, as Derek Lowe recently wrote, “It is very, very rare for knowledge of a protein’s structure to be any sort of rate-limiting step in a drug discovery project” (7). Although AlphaFold can be improved to provide protein structure predictions that could better inform drug discovery, we think that some of the most needed improvements are in molecular docking.
Molecular docking is intrinsically a difficult problem because it aims to predict just one binding conformation (the most energetically favorable one) out of the billions of potential conformations a protein and a ligand might have. Dynamics and other biologically relevant factors aside, this involves simulating many-body interactions between large numbers of atoms. This task is computationally intense, time-consuming, and not easily solved. Supercomputing resources, along with new ways of performing molecular docking that can leverage more information (for instance, from ground truth protein-ligand interaction datasets), might help with this challenge.
To be clear, we think in silicodrug discovery has great potential. Computational platforms, including the one our lab previously used to identify halicin, have often helped the field find interesting lead compounds with limited resources. Our study focuses on showing the limitations of one such computational approach, especially in regard to the challenging task of predicting drug targets.
What’s next for your research?
As part of the Antibiotics-AI Project, we’ve been interested in applying other deep learning approaches to the de novodesign of compounds that could have strong selectivity against bacteria and favorable medicinal chemistry properties (8). We think our study should help guide the drug and antibiotics discovery fields and provide empirical benchmarking data that can be useful in future efforts to predict protein-ligand interactions. We’ve also been exploring whether we could build on our study to develop molecular docking approaches that are better at identifying drug-target interactions or otherwise see if we could further study the proteins for which the accuracy of this approach is encouraging. If so, we could start to more accurately identify compounds that bind to specific bacterial proteins and may have a chance of becoming useful antibiotics.
- Review on AMR, “Tackling Drug-Resistant Infections Globally: Final Report and Recommendations” (2016). Available at: https://bit.ly/3FyPdbl.
- MA Fischbach, CT Walsh, “Antiobiotics for emerging pathogens”, Science, 325, 5944 (2009). DOI: 10.1126/science.1176667.
- LL Silver, “Challenges of antibacterial discovery,” Clin Microbiol Rev, 24, 1 (2011). DOI: 10.1128/CMR.00030-10.
- B PLackett, “Why big pharma has abandoned antibiotics” (2020). Available at: https://go.nature.com/3TV8OqM.
- JM Stokes et al., “A deep learning approach to antibiotic discovery,” Cell, 180, 4 (2020). DOI: 10.1016/j.cell.2020.01.021.
- F Wong et al., “Benchmarking AlphaFold-enabled molecular docking predictions for antibiotic discovery,” Mol Syst Biol, 18, 9 (2022). DOI: 10.15252/msb.202211081.
- D Lowe, “Why AlphaFold won’t revolutionise drug discovery” (2022). Available at: https://bit.ly/3foVQma.
- J Collins, “Harnessing AI to develop the first new class of antibiotics this generation” (2020). Available at: https://bit.ly/3zxgWpo.
Following a Bachelor’s degree in English Literature and a Master’s in Creative Writing, I entered the world of publishing as a proofreader, working my way up to editor. The career so far has taken me to some amazing places, and I’m excited to see where I can go with Texere and The Medicine Maker.