Clinical Trials and Digital Doubles
Digital twins in clinical trials are changing how research is performed – but it won’t be a revolution, according to Phesi CEO Gen Li.
| 5 min read | Interview
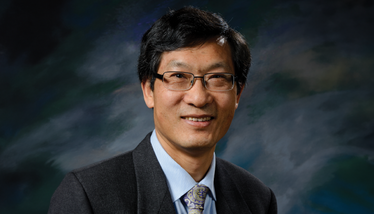
Digital twins are being brought to clinical trials. In manufacturing, digital twins are a virtual replica of a process or parts of a process that can be used by engineers and operators to examine how a process might perform, and to detect or predict potential weaknesses.
In clinical trials, the digital twin concept is being used as a virtual replica of a patient’s tissues and organs to predict treatment outcomes for the control arm of the trial. Unlike a clinical trial, the data used to construct a digital twin come not solely from patients in a healthcare setting, but also from a database to which it was collected with a clearly defined context of who, when, where, and to what end. A digital twin can be used as an external control arm and as a historical control to enhance submission packages for regulatory approval.
The concept could streamline the eligibility and recruitment processes, but it is unlikely to overhaul the proven, traditional methods altogether. Phesi CEO Gen Li tells us why…
Is this the end of the placebo in clinical trials?
The concept of a “sugar pill” placebo is already declining. Trials with active comparators in a control arm are far more common. Digital twins won’t make placebos disappear entirely in clinical development, but they will enrich the development process.
Clinical trials have a history spanning 277 years, since James Lind’s Scurvy Trial in 1747. Over the years, various elements have been added to the toolkit of designing and conducting trials. In 1863, Austin Flint coordinated a 13-person trial for rheumatism that involved a placebo for the first time. In 1946, the first randomized curative controlled clinical trial took place in the UK.
Technology breakthroughs in big data and artificial intelligence have enabled us to gather, tabulate, and interpret patient data, enabling the construction of digital twins. But the introduction of digital twins is a part of ongoing efforts to enhance the clinical trial toolkit, not to replace any of the existing tools, such as placebo arms, active comparators or standard-of-care comparators.
Digital twin technology is a natural byproduct of accumulating patient data from placebo arms, active comparator arms, and many other settings. This large volume of contextualized patient data allows us to simulate the planning, implementation, and outcomes of clinical trials.
How many lives will be improved or even saved through the use of digital twins?
The aim of digital twins is to transform the planning and implementation of clinical trials. Digital twin technology allows sponsors to “meet patients” before starting a clinical trial – eliminating costly protocol amendments through better alignment between trial design and the target patient population.
With a stronger understanding of the patient population, digital twins allow sponsors to better identify investigator sites that can recruit the right patients in the desired timeframe, resulting in higher patient enrollment rates and shorter cycle times. Reduced costs and shortened cycle times mean that more drug candidates can be evaluated, helping bring innovative medicines to patients faster. It is hard to quantify how many lives will be improved or even saved, but the use of digital twins has already been proven to improve and save lives, and reduce the patient burden.
What flaws or new variables have presented themselves so far?
To construct a digital twin in many ways resembles conducting an actual clinical trial. We need guidance from a protocol to “recruit” patients, not from investigator sites, but from a patient database. We then use data from those patients who met our eligibility criteria to construct a digital version of patient baseline characteristics. An efficacy digital twin can be constructed with data from a portion of the eligible patients where the outcome measure(s) and treatment duration(s) are available as being defined in the protocol. We can construct a safety digital twin similarly. Baseline characteristics, efficacy, and safety outcomes are three components in a complete digital twin.
This provides the context for possible flaws or new variables in digital twin construction. First, we may not have enough relevant data. Secondly, there are possibilities of data bias in data extraction and data analysis. Third, there are always possible data quality issues in source documents. We rely on data totality and inclusiveness to overcome the challenges in data bias and data quality. In the digital twins we have constructed so far, having one of two cohorts of outliers cannot impact the statistical significance of the conclusions.
The Medicine Maker Presents:
Enjoying yourself? There's plenty more where that came from! Our weekly Newsletter brings you the most popular stories as they unfold, chosen by our fantastic Editorial team!
What are the obstacles between digital twins and regulatory approval?
A digital twin allows sponsors to explore changes in a trial without the need for regulatory approval; for example, define an optimized development pathway, design a better protocol without need to amend, select better investigator sites, recruit patients faster and with better quality patient data, and interpret results from a clinical trial in early development more accurately, to name just a few.
There are not necessarily obstacles between digital twins and regulatory approvals if we want to use a digital twin to replace a placebo control arm. The FDA, for example, has made it clear that if sponsors intend to deploy a digital twin/external control arm, sponsors need to inform them early ahead of starting a human trial when an IND is filed. Regulators also need to be informed consistently and completely on various progresses and issues. In summary, sufficient groundwork must be conducted to present compelling evidence to regulatory authorities when a digital twin can be used to replace a placebo and active comparator arm. It requires collaboration among several stakeholders to materialize a digital twin/external control arm.
What in your experience is the key benefit of digital twins over a placebo control arm?
There are circumstances where a placebo control arm is not possible because of ethical and other reasons. In this case, a digital twin becomes the only choice, where there is relevant data. Increasingly, a single arm trial with a limited number of patients is allowed by regulators to proceed to phase III. However, sponsors can increase the chance of phase III trials being successful if they harness digital twin technology to understand and interpret the outcomes of phase II trials – and avoid a costly failure further down the line. A digital twin can provide a more accurate interpretation of clinical trial results when there are a small number of patients, helping inform decision making in the next steps of development.
Using a digital twin to substitute a placebo or active comparator can help get innovative therapies to patients faster and reduce development costs. A digital twin over a placebo eliminates unnecessary risk for patients in a control arm. What’s more, a digital twin can include a vast number of patients, which is impossible when using a placebo control arm, allowing sponsors to have greater geographic coverage. In addition, the scale of data over time afforded by these approaches allows for deeper analysis, allowing sponsors to identify trends in the patient population and build more accurate disease models.