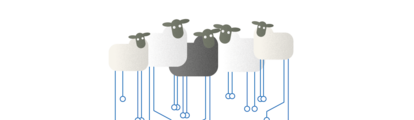
Does AI Dream of Electric Patents?
To reap the rewards of artificial intelligence and machine learning, biotech companies must overcome the legal, regulatory, and commercial hurdles
Amy Nick | | Longer Read
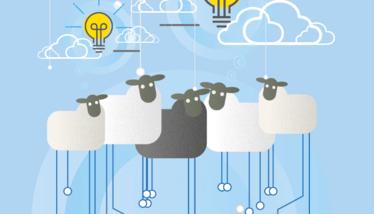
Developments in artificial intelligence (AI) and machine learning (ML) are playing an increasingly influential role in the pharma sector. FDA approvals of AI algorithms have increased exponentially over the past few years (1), and the AI healthcare market is predicted to reach US$6.6 billion by 2021 (2). A 2019 survey of pharmaceutical and biotech professionals by ICON suggested that 80 percent of survey respondents were using, or planning to use, AI technologies (3). The trend has driven the formation of new partnerships between the tech and healthcare industries; for example, AI startup Concerto HealthAI is currently working with BMS, Pfizer, and Astellas, to support precision oncology initiatives, while Roche’s acquisition of Flatiron Health and Foundation Medicine provided proof-of-concept that clinically meaningful insights can be generated through large-scale analysis of genomic and clinical data (4). Meanwhile, major tech players, such as Google, IBM and Microsoft, have all taken steps into the biotech space; among other developments, 2019 saw the announcement of several new healthcare-related collaborations by Alphabet-owned Verily (5), and a partnership between Microsoft and Novartis aimed at integrating AI across clinical development and commercialization (6).
AI and drug design
The use of AI in drug design is considered speculative right now. At the time of writing, no AI-designed drugs have been approved and very few have reached clinical trials. UK-based startup Exscienta was the first company to put an AI-designed drug into clinical trials (7). In collaboration with the Japanese pharmaceutical firm Sumitomo Dainippon, Exscienta succeeded in reducing the development time of its OCD drug to just twelve months. The drug is currently undergoing phase I trials.
The COVID-19 outbreak has created a new sense of urgency as researchers race to develop treatments. There is greater interest than ever before in accelerating the drug development process. With the spread of COVID-19 outpacing the capacity of global healthcare systems, alliances between the pharmaceutical and tech sectors have become more influential than ever in combating the spread of the disease. Though there has been great optimism about AI’s potential to assist in drug development, the COVID-19 crisis may reveal which approaches can truly deliver.
Several companies are already employing AI-mediated approaches to combat the pandemic. BenevolentAI, for example, has applied its proprietary AI platform to the prediction of COVID-19 drug candidates (8). The software highlighted members of the numb-associated kinase (NAK) family as potential targets for treatment, and identified baricitinib, currently used to treat rheumatoid arthritis, as a potential therapeutic agent based on its antiviral and anti-inflammatory properties, and safety profile. Meanwhile, South Korean company Deargen’s deep learning technology has identified the antiretroviral atazanavir, used for the treatment of HIV, as another possible candidate (9).
US-based biotech company Insilico Medicine has taken a different approach. Rather than attempting to identify commercially available drugs that could be repurposed for the treatment of COVID-19, the company employed AI to accelerate the synthesis and validation of new drug candidates. Their platform has identified six new small molecules, predicted to target a key viral protease, which they suggest could be synthesized and tested for efficacy. Meanwhile, Moderna, the first company to bring a COVID-19 vaccine into Phase 1 trials, suggested that its $100 million investment in digital technologies (including AI) was a key factor in its ability to push products rapidly through the development cycle. Indeed, the speed at which Moderna responded to the emergence of the novel coronavirus is considered unprecedented. Phase III trials have already begun for the company’s mRNA vaccine.
AI meets IP
When it comes to using AI in the drug development process, companies need to consider how they create and protect their intellectual property (IP) – especially with the trend towards personalized medicine. With some products being applicable to just a handful of patients, there is likely to be a greater emphasis on patents that capture the potential value across all stages of the clinical development process – not only the final product. In particular, patents will need to protect novel strategies for accelerating drug discovery, improving patient selection, and enabling treatment optimization, as well as methods of data capture and the analytics underpinning them.
Obtaining such protection will not be without its challenges. In Europe, for example, the approach of the European Patent Office (EPO) to patentability in this area is still evolving. In 2018, the EPO updated its Guidelines for Examination to include, for the first time, specific guidance on how the patentability requirements for algorithms and computer programs should be understood in the context of AI and ML. Meanwhile, in decision T 0694/16, the EPO’s Technical Board of Appeal acknowledged that a claim directed to the use of a known drug in a purposively selected patient subgroup could be considered novel, even where the identified subgroup overlapped with the previously treated patient group (10). This decision paves the way for patentability of existing drugs that have been identified by AI and ML platforms, such as those used by BenevolentAI and Deargen, as potential candidates for repurposing.
In addition, broader questions arising from the use of AI are likely to impact approaches to IP in biotech. Standards for inventiveness may need to be revised, as AI interprets and processes information in an entirely different way to a human inventor. Under current law, to obtain a patent, the invention must not be obvious to a person of skill in the relevant field, on the basis of publicly available information. Yet questions will arise as to how this standard should be applied in the context of AI-generated predictions. While it could still be argued that Insilico’s novel protease candidates are within the scope of what could be achieved by a skilled synthetic chemist, for example, this type of algorithm could conceivably identify drug candidates that are entirely non-obvious to a human expert, but nevertheless an obvious outcome of the application of AI. The more commonplace these methods become, the more difficult it may be to determine the inventiveness exclusively by reference to the perspective of a human inventor.
Such applications of AI also raise issues around the nature of inventorship. Currently, inventorship is generally considered to reside with the person who developed the AI. Yet this situation is likely to become increasingly complex as the capabilities of AI develop and the role of human supervision becomes less prominent. There are currently no specific legal provisions addressing the notion of AI as an inventor. And most jurisdictions require the named inventor to be a natural person (11). Both the UK Intellectual Property Office (UKIPO) and the EPO recently rejected applications because the named inventor was an AI named DABUS, despite acknowledging that the criteria for patentability were met, and the UKIPO has now updated its Manual of Patent Practice to explicitly exclude the AI being named as an inventor.
But this is unlikely to be the end of the issue. As technologies developed by unsupervised learning algorithms become more prominent, we’re probably going to see more cases where the extent of the developer’s oversight is increasingly insufficient to justify human inventorship – bringing the issue back to the fore.
As more companies switch to AI- and ML-driven approaches, the case law will necessarily develop to take account of such issues and ensure that AI-driven biotech inventions do not risk slipping through the gaps in current IP law. Drug development is a notoriously costly process, and the chance of not being able to obtain a return on investment is likely to significantly disincentivize innovation. The field also needs a balance between ensuring companies can protect the value of their investment and making sure that the monopolies do not unduly limit the potential for progress. Ensuring that a consistent approach to patenting AI and ML inventions will also be important here. Patents require public disclosure; without robust systems for protecting IP, companies may increasingly choose to protect novel AI and ML processes as trade secrets – depriving the research community of the opportunity to build on their progress.
These are fundamental issues and navigating them will be complex, requiring careful consideration and close collaboration with stakeholders across the pharmaceutical and tech industries. Addressing these uncertainties surrounding the role of AI within the biotech field will be essential to move towards an era where the industry can truly embrace technology.
The Risk of Error
So far, few drug development predictions made by AI have been validated, and the extent to which many of these technological solutions can be implemented in the real world remains to be seen. Critics have also alleged that, although AI may be faster than medicinal chemists at identifying novel drug candidates, the development process for these drugs does not necessarily lead to better outcomes. Nevertheless, the risk of failure is an unavoidable part of drug development and achieving the same outcomes at an accelerated rate now, more than ever, appears a goal worth pursuing. Validation of AI predictions is likely to be expensive and time-consuming, especially where they require the synthesis and trial of new compounds or large-scale clinical trials. Companies investing in this kind of research need to be convinced that the chances of success are worth the risks.
The challenges of using and validating AI can be emphasized by looking at the healthcare sector and diagnostics. Recently, an AI algorithm developed by Google Health in collaboration with Imperial College London made headlines for out-performing human radiologists in the diagnosis of breast cancer. A meta-analysis comparing the diagnostic performance of deep learning algorithms and healthcare professionals suggested that algorithms performed at least as well as human experts in diagnosing a wide range of diseases from medical imaging. However, the authors noted that very few of the studies they analyzed were carried out in conditions that realistically reflected clinical practice. And we must remember that the margin for error is low. Despite the interest in using AI to diagnose patients, the reality is that any mistake could cost lives. This risk is particularly problematic for unsupervised algorithms, which generally offer little insight into the processes underlying their final output, leaving healthcare professionals unable to determine whether anything critical may have been missed. Further work is needed to demonstrate the extent to which algorithm-based approaches to diagnostics could lead to tangible benefits for patients and healthcare systems. Even the most advanced machine learning models are limited by the quantity and quality of the datasets they are trained on, and in the healthcare sector, much of this data may still be inaccurate, incomplete, or biased towards specific populations. Furthermore, algorithms cannot yet take the full clinical picture into consideration in the way that a human doctor would, nor are they able to account for the wider context of a problem, such as its emotional or economic impact.
Although new collaborations between tech giants and biotech or healthcare companies have the potential to drive significant technological progress, they also give rise to a new set of legal, ethical and regulatory issues, which must be resolved soon if progress is to be made at the speed envisaged by the tech sector.
- Medical Futurist, “FDA Approvals For Smart Algorithms In Medicine In One Giant Infographic” (2019). Available at: https://bit.ly/3h5C3nM
- Stanford Medicine, “The Democratixation of Health Care” (2018). Available at: https://stan.md/2MRUU85
- ICON, “How digital technologies will transform R&D productivity” (2020). Available at: https://bit.ly/30murHl
- Foundation Medicine, “Foundation Medicine and Flatiron Health Publish Validation of Clinico-Genomic Database as a Platform to Advance Oncology Therapeutics Development and Personalized Cancer Care” (2019). Available at: https://bit.ly/2UmCjoC
- Biopharma Dive, “Verily lures major pharmas with promise of speedier clinical research” (2019). Available at: https://bit.ly/3h2pMQW
- Novartis, “Novartis and Microsoft announce collaboration to transform medicine with artificial intelligence” (2019). Available at: https://bit.ly/3eZYqJo
- Exscientia, “Financial Times: Read the article detailing Exscientia s work on the first AI-designed drug to enter clinical trials” (2020). Available at: https://bit.ly/2zf4U87
- The Lancet, “COVID-19: combining antiviral and anti-inflammatory treatments” (2020). Available at: https://bit.ly/3h8eeLR
- Deargen, “Deargen Predicted Potential Antivirals for The Novel Coronavirus Infection using AI” (2020). Available at: https://bit.ly/2BDfkiu
- European Patent Office, “T 0694/16 () of 15.5.2019” (2019). Available at: https://bit.ly/2Ac9B2M
- WIPO Magazine, “The Artificial Inventor Project” (2019). Available at: https://bit.ly/3cKV4IP