Failing Safely: Why Ending Some Programmes Early May Be a Recipe for Successes Later
With so much time and money invested into pre-clinical research and early phase clinical trials, why are compounds still failing at the final hurdle?
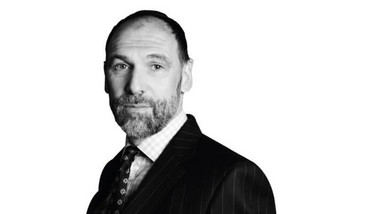
It was Thomas Huxley who wrote, “The great tragedy of science [is] the slaying of a beautiful hypothesis by an ugly fact.” Pharma’s recent history is filled with examples where unexpected proofs regarding lack of efficacy have emerged to kill promising products late on in their development cycle.
Late-stage candidate failure can heavily impact a company’s stock and projected earnings. One of the best-known examples in recent years is Novavax’s ResVax. ResVax is a respiratory syncytial virus (RSV) vaccine targeting the post-fusion F-protein. Despite promising results at phase 2, late-stage trials failed to meet their primary endpoints. Subsequently, Novavax’s share price fell by 96 percent over a five-year period.
In another example, a lack of efficacy observed for Regeneron’s anti-RSV antibody suptavumab led to losses of over $270 million in 2017. This humanized-monoclonal, also failed to meet its primary endpoints at phase 3, despite being the subject of “accelerated development” aligned to encouraging early phase data. In the same year, Aviragen’s BTA585 drug failed show significant reductions in viral load in a controlled human infection trial. This additional reverse brought total financial losses in RSV therapy investments alone for just one year to over $1 billion, inclusive of share price adjustments.
The industry recognizes that the cost, as measured both in time and money, of bringing a drug to market is increasing – currently, estimates are in the region of $2 billion, spread over a period of up to 12 years (based on a final success rate of approximately 10 percent). Given the enormous sums involved, why do so many drugs fail so late in the process? Prior to 2000, the main reason for candidate failure in late phase studies was safety. Improvements in PK/PD modelling reversed this trend, but other variables emerged to fill the gap. Efficacy has now leap-frogged safety to become the primary reason for late-phase failure, with commercial pressures such as price or pipeline rationalization also contributing significantly to withdrawals or late-stage project termination.
Given the structure and principles of drug development, a lack of proven efficacy should be a minority reason for failure. Prognostic correlates (for example, correlates of protection) and other objective measures should be evidenced during pre-clinical and early clinical studies, primarily during phase II, before a product progresses to a large field trial. Allied to the value or strength of prognostic markers, relevant powering (which is to say, recruitment of subjects to achieve “n”, the number required to demonstrate that observed events are unlikely to be related to the product’s effects and not to chance), is essential if outcomes are to be considered valid. Study centers with a track record of success are historically more likely to meet enrolment targets – often because they have proven recruitment strategies (for example, rare-disease databases, investigator engagement and, most importantly, enthusiasm). These, in addition to such measures as time from ethics approval to first enrolment and the allocation of a dedicated clinical trial coordinator, all make for good predictors of success or failure to enrol. Adequate funding of all of the above may also impact significantly on success rates, with around 22 percent of small/medium companies being unable to conclude trials for financial reasons alone.
The norm for return on investment (ROI) for pharma is 25 percent or less, based on drugs already well into the clinical development cycle. Many of the reasons for poor ROI remain intractable, such as candidate failure due to low incidence of disease and undesirable side effects (adverse events). Late-stage findings regarding lack of efficacy remain irregular as the discovery process is predicated on finding effective ways of altering actions and reactions in a predictable manner for a given set of indices. Where such indices or endpoints are intractable (e.g., symptom resolution in hospitalised patients) or poorly prognostic, trials have a greatly increased chance of failure.
Candidate failures will never be wholly avoidable, as research by its very nature incorporates elements of the unknown, but there are steps that can be taken early in the research cycle to reduce the number of late stage failures. Some of the issues with efficacy could perhaps be resolved by better interpretation of pre-clinical data and less optimistic analysis and interpretation of data. One factor above all others that has been proven to safeguard and accelerate development is the availability of a strong correlate of efficacy. Many studies must rely on observational or subjective measurements, such as symptoms, to infer that a treatment improves a patient’s condition or welfare – often due to the lack of objective markers of disease. Strong correlates can considerably shorten the time to licensure for drugs and vaccines but, equally, the incorrect use or interpretation of correlates can confuse rather than clarify the response of an individual to a given intervention. For example, random, ordinal rankings of disease severity, such as “1-25”, “A to D,” “mild to severe” may be poorly transferrable or comparable between studies. Correlates may, upon investigation, fail to show correlation to effect, being non-functional covariates with little or no direct relation to mechanisms of interest. We should, therefore, be careful in the invocation of correlates to prove or disprove cause and effect. It’s also worth noting that out of over 150,000 published biomarkers in 2011, only some 100 are regularly used in clinics today.
To estimate the real-world predictive value of healthy volunteer studies, we often have to look backwards; using data from similar late phase studies and the prognostic value of animal and early clinical efficacy data in that indication. Additional in silico or in vivo prognostic modelling prior to testing novel compounds in large field studies may also decrease risk. Increasingly, in my own view and those of many clinical trials professionals, controlled human infection modelling (CHIM) can add value as the “next-step” from preclinical work in animals and provide strong bridging data to humans. Employing the correct human model to substantiate pre-clinical findings can validate decisions regarding both candidate selection and dose selection. Recent studies that I have been involved with have seen the CHIM model provide solid evidence of efficacy and offer additional immunological data to assist in vaccine design and delivery programs.
Getting pivotal phase II studies to be predictive of field behavior is the key to safeguarding investment in phase III. There is no crystal ball to reliably predict low-incidence safety signals, but efficacy should be a given by the time the sponsor invests large sums into a large field trial.
Adrian Wildfire has worked as an infectious disease specialist for over 30 years, having trained and worked within the fields of bacteriology, virology, parasitology and mycology after obtaining his Fellowship in Medical Microbiology in 1990, and a Masters in Parasitology in 1998. He has specialised in Human Challenge Models for nearly 10 years and is currently leading a multidisciplinary team manufacturing challenge agents for use in clinical trials. He is the author of numerous published papers and articles relating to HIV, ethics and viral challenge amongst others.