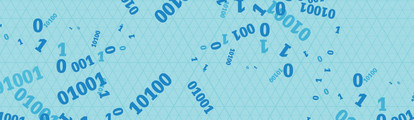
Giving Data the Gift of Meaning
Ever-growing datasets are a constant challenge when trying to develop efficient clinical trials. As more conventional approaches fail to give companies the competitive edge they need, can the insight provided by predictive analytical tools pick up the slack?
Gen Li, Jonathan Peachey | | Longer Read
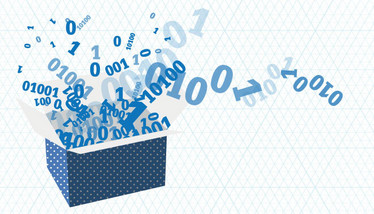
An underpinning value of the pharmaceutical industry is its desire to provide solutions where there seem to be none – and yet each year the pharma machine continues to churn out drugs, which, rather than being first-of-kind treatments to combat areas of unmet need, are often alternative formulations of existing products. Despite the millions of dollars annually spent on R&D, true scientific breakthroughs seem few and far between.
Big data has become an industry buzzword – and could be particularly useful for improving clinical trials. The industry knows that big data has huge potential but pharma companies are inundated with data from various sources, which is often found in different formats and grows at an incredible velocity. Selecting data that will significantly impact the quality of clinical trials and result in breakthrough medicines is more than a trying task.
While trials are intended to be high quality, transparent, and discoverable, companies are often at a loss as to how to handle the vast amounts of data available to them. The large volume of structured and unstructured information companies encounter can significantly reduce the visibility and discoverability of pertinent data as making true comparisons between trials can become a virtually impossible task when handled manually. And the problem is only exacerbated by the rapid rate at which datasets grow.
Clinical trials are also plagued by other issues:
- Poor recruitment. Investigators often overestimate their ability to recruit suitable patients to trials. In some therapy areas, there may be smaller populations of patients for certain indications and public awareness can also be poor, resulting in fewer people engaging with the recruitment process. Strict exclusion criteria can also hinder recruitment.
- Misinformed site selection. Trial sites should have sufficient resources and experiences to effectively manage a trial. A proven history of performance in similar studies is important, but does not guarantee that each site will perform well in every trial. The lack of detailed guidelines and lack of due diligence done to inform site selection can result in trials set up with the wrong number of sites, in the wrong geographies. The systematic reviews required to ensure that the best sites are chosen can often fail to take place.
- Patient burden. Clinical trials can be an inconvenience to patients. Patients must commit themselves to commuting to research sites and dealing with imposed obligations, such as regular medical tests. When the relationships fostered with patients are poor, their adherence to the terms of these trials may dwindle.
Ineffective trial design. An example of this is the deliberate targeting of older patients (aged 70 or above) with an ECOG performance status of 2 (meaning that they are ambulatory and capable of all selfcare, but unable to carry out any work activities and are up and about more than 50 percent of waking hours) for an indication such as lung cancer. In doing this, many companies will fail to create impactful trials as only a small percentage of the patient population has been targeted. However, pharma companies often default to this type of trial due to pressure from regulators. The FDA, for example, requires that medicines be trialled in special populations – one of which is the geriatric demographic. While they cannot be blamed for this, predictive tools would allow them to realize that this type of trial would most likely fail before it has even taken place.
Predicting a data revolution
When clinical trial outcomes fail to translate into benefits for patients, the consequences are dire. Money, time and resources are wasted, and little value is derived. Clinical trials are overdue for an overhaul – AI and machine platforms are the way forward. Some have said that around 80 percent of the data we have today has been created in the last ten years. There has been a data explosion in the last decade. How can individual people cope by manually inputting data, identifying the relevant data and interpreting it?
Predictive analytics is arguably the best tool at any company’s disposal when trying to find the needles in big data haystacks and design better trials, with the right sites and the right patients. Predictive analytics provide flexibility and dynamism in ways that more conventional approaches fail to. The rich types of data companies acquire and license cannot be thoroughly analyzed using resources which provide unrefined, basic information about individual trials. Predictive analytic tools are AI-driven platforms that can trawl through large datasets and identify the data most relevant to companies. They can be designed to accommodate ever-growing data sets and have algorithms that allow them to handle data from any source, in format, and allow them to be compared to create recommendations. In our database, for example, we source data from over 70,000 data records. This number grows by around 6,000 records a day. This is an incredible amount of data and with the right tools you can pull effective insight that can help with matters such as finding the right type, location and number of investigator sites with the right capacity and protocol design in a matter of days, rather than the enormous timelines associated with manual research. Imagine the benefits of being able to use a platform that can analyze “what if” scenarios. What if I was to include X patients in my protocol versus Y patients? Does epidemiology data tell me that there are specific kinds of patients who suffer from this illness?
Of course, even with predictive analytics and other data mining techniques there will never be absolute certainty, but we should be able to use data more effectively and predictably to create value.
A new mindset
At one end of the spectrum, there are many passionate people and companies in the industry who are quick to embrace new technology. There are many companies that have expressed their interest in moving toward more AI-led forms of data analysis, but we find that progress is often hindered because many companies seem unsure of what options are available to them and what it really means for the performance of their valuable trials. But at the other end of the spectrum is the fact that the industry as a whole can be very conservative, preventing companies from expanding their horizons! Just look at how long it has taken the industry to move to electronic data capture.
Though risk-aversion is a problem commonly experienced in pharma, we’ve found that small to mid-size companies have been quick to trial – and in many cases – adopt these technologies. Some big pharma companies are even building their own platforms, but algorithmically analyzing data in real-time may present problems given the rapid growth of data. By 2020, 1.7 megabytes of data will be generated per person per second. It takes, on average, five years to develop functioning platforms, by which time the data records have already developed to an even more overwhelming size.
Another challenge is that the pharma industry is highly compartmentalized, resulting in the development of functional silos – all of which are key actors in the design of protocol and site selection. Predictive analytics break down the barriers that so often separate professionals whose work lies in the fields of statistics, operational design and medicine. The beauty of these tools is that they work in an integrated manner allowing for study optimization and the prompt activation of sites.
While these two aspects of clinical trial design may seem obvious to clients, this often can’t happen using the conventional CRO approach. The use of CROs has historically resulted in poor design, the overuse of underperforming sites for clinical trials and poor recruitment. The majority of trials they design do not optimize centers well or to the same degree that automated platforms can. And while this is not due to ill-intention on their part, their methods simply cannot keep up with the constant floods of data that the industry receives. Many CROs have realized this and are now focusing on data – and it’s not uncommon to see large CROs publishing media statements about data acquisitions to show they are serious about data analytics.
But it’s not about just acquiring data. Without the algorithms to extract information, data is meaningless. You could have the world's largest database but if you can’t filter, compare and contrast that data, there is no value. If you are serious about using your data effectively, don’t just buy data; you must invest in the platforms that can mine this data effectively! To address this, we just started to roll out a self-service tool that companies can license to do their own investigator site searching, filtering and selection - utilising our massive, real time database. We will be adding another integrated module to help companies with protocol planning, enabling them to identify design issues that are the root cause of many recruitment delays.
Predictive analytical tools may seem intimidating and it is common for people to worry that their jobs may be replaced. But predictive analytical tools and data algorithms have not been designed to replace or eliminate roles – they are only helping to redefine them and guide the best path forward for clinical development.
Data is a competitive lever and when taken advantage of, allows companies to reduce costs, compete more aggressively and serve their customers and patients better. It is only when the industry let’s go of the momentum of traditional thinking that the greatest successes for clinical trials will be seen.
Meet Phesi
The company?
Gen Li: Before creating Phesi, I’d been in the industry for a long time, and one of the biggest frustrations for me has always been the uncertainty of the clinical development process: uncertainty in terms of the time frame and costs. You anticipate that a trial will be short and not too expensive, but as soon as you start the picture can change quickly. The pharma industry invests huge amounts into R&D and we need some sort of structure to see through the data noise and identify patterns.
This motivated me to create Phesi and to focus on AI and data-driven products and services. And we are making good progress in terms of analyzing data and transforming that into knowledge that can be used to make important clinical trial decisions. Do we know everything? No, but we now have an effective way to look at some very important issues associated with the clinical trial process and identify the fundamental reasons behind these.
The focus?
Jonathan Peachey: We are trying to use predictive analytics to provide more certainty in an unpredictable world. It is not the pharma industry’s fault that it is struggling with internal challenges around data. What data do I need? How much data is there? What is relevant? How do I act on it?
Gen was the visionary behind Phesi. I'd been working in parallel at the time with IBM, who were thinking about IBM Watson, and smart analytics and smart planners. I met Gen and I was impressed because he demonstrated the benefits in speed and cost that could be achieved by harnessing data.
Throughout my time working with Gen, we have always encouraged our clients to adopt an integrated view when it comes to clinical trials and not to be afraid of agile deployment. To test that the platform that we provide delivers on what we say it offers. Within the space of a month, you’ll know if we can do what we said we can do.