Harnessing AI for Pharma's Next Leap
Discussing the finer points of AI as a catalyst for pharmaceutical innovation
Tero Laulajainen | | 3 min read | Opinion
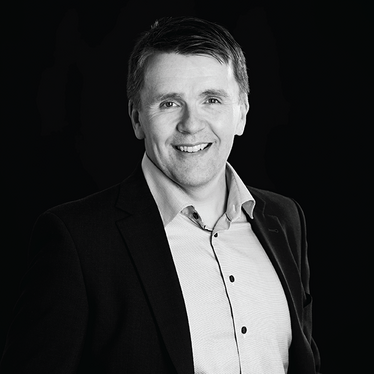
Credit: Author supplied
I’ve seen firsthand how AI can act as a powerful innovation catalyst in many aspects of drug discovery and development. It can be trained to predict trends and outcomes in pharmaceutical research and market behaviors, including forecasting drug demand, understanding disease outbreaks, and anticipating market changes. These predictions can form part of the decision making process regarding which drugs to develop, as well as the amounts to be manufactured.
Many potential drugs fail in clinical trials, but we will soon be at the point when AI will be able to analyze extensive datasets and identify potential drug candidates (whether new chemical compounds or existing drugs with the potential to be repurposed). Likely outcomes for drug development success, as well as the ability to streamline discovery and reduce costs, will also be accelerated by AI. In an ideal world, we could use AI to predict side effects and thereby reduce the chances of unforeseen adverse reactions.
When it comes to trials and effective trial protocols, real-time data analysis will identify trends or issues earlier than ever, allowing for quicker, more flexible adjustments and improved safety and efficacy.
Beyond the lab, the positive impacts of AI on manufacturing and supply chain management include optimized production schedules, anticipated maintenance needs, ensured quality control, improved transparency, traceability, and the real-time monitoring of shipments. Direct-to-patient delivery in remote clinical trial settings would therefore become more efficient and limit the risk of patients deviating from treatment schedules.
Looking to the future, I see an expanding role for AI in pharma. Beyond enhancing drug discovery and development, AI will become integral to patient monitoring and gathering real-world evidence. Perhaps this will lead to the integration of trials into clinical care, which are typically “standalones" in a patient's treatment history. After gaining patient consent, the treatment journey of the clinical trial could be followed as an integrated part of their healthcare journey through direct access to their electronic healthcare records. This novel way to collect data would provide a comprehensive, real-time understanding of the safety and effectiveness of emerging treatments. It also brings substantial value in post-marketing surveillance by enabling closer monitoring of recently approved therapies with little disruption to patients and healthcare providers.
I envision a future where AI and human expertise blend to create a more efficient, personalized, and patient-focused pharmaceutical industry, supercharging R&D, and allowing innovation to thrive. However, I strongly believe that pharma cannot solve the world’s health problems on its own. Collaboration is the way forward, and advanced technology companies that can introduce scientists to new AI tools will be the gatekeepers.
About the author
My background is in life sciences, having studied biochemistry at the University of Helsinki. After graduating, I began my career as a scientist working in research for a pharmaceutical company. Following this, I moved into clinical operations where I discovered my passion for ensuring robust global clinical trial design. I joined UCB in 2017, as Vice President - Head of Global Clinical Science and Operations. I lead the operational design, execution, and reporting of global clinical trials across all therapeutic areas and phases. I am passionate about driving digital transformation in the clinical operations ecosystem and continual process improvement.
Vice President – Head of Global Clinical Science and Operations, UCB