Predictive AI in Drug Discovery: Five Steps to Success
The use of AI in small molecules drug discovery is driving the sector forwards in big ways – but there are big challenges too. Here are five steps to success
Mirit Eldor | | 5 min read | Opinion
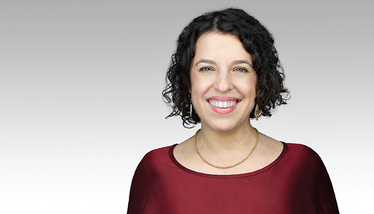
The preclinical phase of drug discovery is the most time intensive stage of the R&D lifecycle – taking up to six years and accounting for more than 40 percent of total drug development costs. To reduce the billions spent on preclinical drug development, faster, more efficient R&D workflows must be a priority across the industry. So it’s no surprise that pharmaceutical and biotechnology companies are looking to use machine learning (ML) to revolutionize R&D and AI to generate and validate small molecule drug discovery pipelines.
Research organizations that successfully deploy AI are already gaining a competitive edge. There is emerging evidence that these organizations get through preclinical stages quicker and cheaper than the traditional approach, with savings of around 30 percent of time and cost. The approach is already gaining traction; one study by the Boston Consulting Group found that biotech companies that have adopted an AI-first approach, “…have more than 150 small molecule drugs in discovery and more than 15 already in clinical trials.”
Predictive AI is one AI approach that many pharmaceutical and biotech companies are exploring today. Here are five steps that research leaders should follow to realize success.
1. Identify the right use cases
Before investing in predictive AI, research leaders must define the problems, or use cases, that they want to tackle. Typically, the best applications for predictive AI are discrete tasks and processes where measurable, tangible gains can be achieved. In early drug discovery, examples of predictive AI use cases include predicting the 3D structure of a protein, relationships between molecules based on their chemical structure, and drug-target interactions.
In small molecule discovery, predictive retrosynthesis combines high-quality reaction data with AI to find structural or chemical patterns that correlate with specific compound properties and accelerate synthesis planning of novel molecular entities. The potential benefits of predictive retrosynthesis over traditional approaches are significant; routes can be generated for novel compounds in minutes rather than weeks.
2. Source accurate and high-quality data
The nuance of research questions in drug discovery demands a level of precision that requires high-quality, verified training data. Without accurate and high-quality data, researchers will lack confidence in predictive AI outcomes. For predictive models to work, researchers will want to include data from multiple sources in addition to their internal data. This will typically include data from scientific literature, plus other databases containing patent data, regulatory data, clinical trials data, safety data, and data from patient records.
For example, a predictive AI chemistry model requires a breadth of chemistry inputs that includes not only proprietary data and data on failed reactions, but also published literature. A predictive model that is fine tuned using incomplete data will produce inferior results whose shortcomings may not be immediately identified, leading to expensive incorrect decisions.
3. Prepare and structure the data
Once data is acquired it must be structured to power predictive AI successfully. Much of the data R&D organizations source are not AI-ready; datasets are siloed and stored in myriad formats with insufficient metadata, making it difficult to retrieve and use in predictive AI models. Standardizing and structuring datasets via the application of ontologies is a critical step.
Ontologies are human-generated, machine-readable descriptions of categories. They standardize data against an agreed vocabulary, providing a shared language across an organization. Vocabularies can include terms specific to an organization – such as product names – alongside industry recognized concepts and terms. Ontologies define semantic relationships to other classes and capture synonyms, which is essential where there are multiple ways to describe the same entity in scientific literature and other datasets. For example, the gene PSEN1 can also be referred to as PSNL1 or Presenilin-1.
4. Semantic enrichment
To extract insights, datasets must be enriched and annotated. Semantic enrichment is a key step that unlocks the full potential of data in structured and unstructured, public and proprietary, datasets. It transforms text into clean, contextualized data, free from ambiguities and synonyms, through annotation, tagging and adding metadata. It works by employing text analytics to extract key words, concepts, and terms for predictive models, and harmonizes synonymous terms for better accuracy.
Data harmonization is especially important when using databases from multiple sources as technical terms or abbreviations are often used. For example, sophisticated semantic enrichment software can identify and extract relevant terms or patterns in text and harmonize synonyms, such as “heart attack” and “myocardial infarction”, so they are identified as the same entity by a predictive model. This eliminates “noise” and ensures predictive AI models are underpinned by high-quality, enriched data.
5. Domain specificity
Structuring data for predictive AI through ontologies and applying semantic enrichment methods is highly specialized work that requires expert understanding of the domain under investigation. General purpose AI models developed by technology companies have utility in broad areas such as marketing and operations, but scientific research represents a set of niche challenges that necessitates domain expertise.
Few biopharma companies today will have the right mix of skills needed for tasks such as creating ontologies in-house. And though they are experts in their scientific field, researchers lack the technological capabilities required. Best positioned to solve this challenge are data scientists who can couple technology skills with scientific domain expertise. Such data scientists can bring an understanding of the context of questions asked in relation to the data available. They can further ensure ontologies and vocabularies are built so that predictive AI models return relevant results, and no essential data is missed.
The world is in agreement: AI will be a game-changer for every industry. For those working in preclinical drug discovery, the opportunity is huge – but so is the challenge. To accelerate drug discovery to meet the medical needs of patients around the world, pharma and biotech organizations need to bring together data, technology, and expertise. When these elements converge, AI can serve as a valuable support tool for researchers to usher in a new era of drug discovery.
Managing Director, Life Sciences Solutions, Elsevier