Sense and Sensitivity
How quantum mechanics and machine learning can be combined to develop new drugs
Mario Öeren | | 3 min read | Opinion
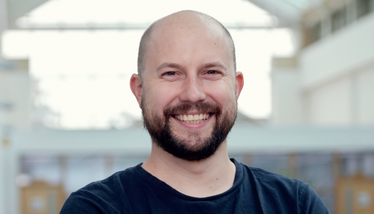
Credit: Author supplied
The make or break of getting your drug approved may very well come down to the “making” or “breaking” of your compound into its metabolites. Which metabolites form – and how fast? Are they toxic or reactive? A good grasp on your compound’s metabolism is crucial. Speaking from personal experience, early stage in silico modeling can help avoid late-stage failures caused by unexpected metabolism. But it can be difficult to balance both sensitivity and precision.
In short, we must attempt to predict a large number of potential metabolites in the hope that all experimentally observed metabolites are covered, while also forecasting a select few to filter out less probable metabolites and concentrate on the critical ones. In an ideal scenario, the models will have high sensitivity (covering all experimentally observed metabolites) and high precision (metabolites not seen in the experiment are not predicted).
Many methods suffer from vast overprediction, making it difficult to identify what the true in vivo effects of a compound will be – an issue often attributed to the rule-based machine learning methods that most models use. These methods, whilst very fast, do not take into account the fundamental reaction mechanisms of the enzymes involved in drug metabolism.
Over the last seven years, we have focused our efforts on building models based on quantum mechanics and machine learning to identify which enzyme families metabolize a given compound and which part(s) of a compound are likely to get metabolized (1, 2, 3, 4). These regioselectivity models predict likely metabolites based on reactivity and accessibility. In this approach, reactivity is shaped by the enzyme-specific reactions while accessibility considers the structure of the corresponding enzyme.
Using the reaction types catalyzed by each enzyme family, we can build simplified quantum mechanical models to predict the reactivity of each potential site of metabolism on a compound. In terms of accessibility, compound orientation within an enzyme’s active site is governed by its structure. And that means the molecular shape and functional groups near potential sites of metabolism play a decisive role in determining whether a particular area can access the active site; thus, some sites will be less vulnerable to metabolism than others. By integrating machine learning to amalgamate the effects of reactivity and accessibility for each enzyme, we are now able to create an accurate picture of regioselectivity.
In addition to the regioselectivity of each individual enzyme family and isoform, it is also important to understand which enzyme families or isoforms are most likely to metabolize your compound. Classification models can be harnessed to give a quick indication of the major metabolizing enzymes for a specific compound, both across different enzyme families (5), or for isoforms within a specific enzyme family (6).
Combining the outputs of these models can substantially increase the precision of metabolite predictions without a considerable decrease in sensitivity. This, in turn, allows us to pinpoint the most likely experimentally observed metabolites, aiding interpretation of metabolite-ID experiments. We can also assess potential toxicity and guide compound design to avoid particular metabolic liabilities.
Ultimately, our research is still ongoing, and there are many further avenues for exploration – more enzyme families to model or specific enzymes within different preclinical species to consider. I’m particularly interested in the speed of these calculations; quantum mechanical simulations tend to be computationally costly, but there are ways to improve (7). All in all, metabolism prediction is an exciting, fast-paced field. I look forward to seeing how we can make even better, faster predictions in the coming months and years.
- JD Tyzack, et al., “Predicting Regioselectivity and Lability of Cytochrome P450 Metabolism Using Quantum Mechanical Simulations,” J Chem Inf Model, 56, 11 (2016). DOI: 10.1021/acs.jcim.6b00233.
- M Öeren, et al., “Predicting Reactivity to Drug Metabolism: Beyond P450s – Modelling FMOs and UGTs,” J Comput-Aided Mol, 35, 4 (2021). DOI: 10.1007/s10822-020-00321-1.
- M Öeren et al., “Predicting Regioselectivity of AO, CYP, FMO and UGT Metabolism Using Quantum Mechanical Simulations and Machine Learning,” J Med Chem, 65, 20, (2022). DOI: 10.1021/acs.jmedchem.2c01303.
- M Öeren, et al., “Predicting Regioselectivity of Cytosolic Sulfotransferase Metabolism for Drugs”, J Chem Inf Model, 63, 11 (2023). DOI: 10.1021/acs.jcim.3c00275.
- M Öeren, et al., “Predicting Routes of Phase I and II Metabolism Based on Quantum Mechanics and Machine Learning”, J Chem Inf Model,” 63, 11, (2023). DOI: 10.1080/00498254.2023.2284251.
- PA Hunt, et al., “WhichP450: a Multi-Class Categorical Model to Predict the Major Metabolising CYP450 Isoform for a Compound,”J Comput.-Aided Mol Des, 32 (2018). DOI: 10.1007/s10822-018-0107-0.
- E Gelžinytė, et al., “Transferable Machine Learning Interatomic Potential for Bond Dissociation Energy Prediction of Drug-like Molecules,” J Chem Theory Comput, 20, 1 (2024). DOI: 10.1021/acs.jctc.3c00710.
Mario Öeren has a background in computational chemistry, with a PhD in Natural Sciences from Tallinn University of Technology, where he has also held roles as a Lecturer and Research Assistant. Since joining Optibrium in 2017, Mario has led much of the company’s research and development efforts into metabolism prediction, developing new models based on quantum mechanics and machine learning for predictive modeling.