The AI and Data Problem in R&D
And how it can be explained with a parable of three farmers…
Quin Wills | | 6 min read | Future
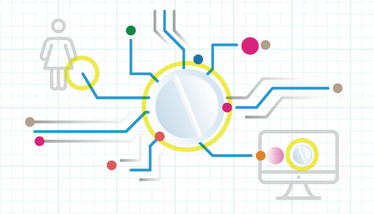
Most of us in drug research look forward to a time where life-changing medicines don’t take 12–15 years or cost $2.5 billion (on average) to reach patients in need (1, 2). I’ve spent almost two decades in liver disease research, so I’m painfully aware of the fact that chronic diseases are responsible for over 41 million global fatalities annually and contribute to an annual healthcare expenditure of $3.79 trillion in the US alone (3, 4).
A question dominating conversations around the future of drug development is whether artificial intelligence (AI) will prove itself to be a catalyst for better, cheaper, and faster R&D. Or will it remain in “hype and hot air” territory (5,6)? In a June 2023 report, authored by BCG and Wellcome, 84 percent of AI users and 70 percent of non-users believed that AI will exert a “significant impact” on drug discovery in the coming half-decade (7).
The optimist in me agrees with this statement, albeit with a cautionary note that AI's near-term success hinges as much on data advances as it does algorithms. AI needs to be fuelled by good data, just as humans function best when fuelled with nutrient-rich food. And whilst large language model chatbots might have cheap data (the internet) in abundance, good biological data rarely comes cheaply or easily.
Many people believe we have so much data that the biggest challenge is making sense of it all (8); however, this notion has fueled a recent bias towards investment in algorithms, which, in some cases, is doing more harm than good because it is diverting funds away from data. In biomedicine, we are at the very start of a journey towards generating human data that is both causal and contextual, clearly mapping (gene) targets to cells, tissues, organs, and disease states. We need to double down on investment in three specific areas of data innovation, which I often explain with reference to the following parable of three farmers.
A tale of data production
There once was a village renowned for growing the best vegetables in the land. Unlike other places, this village had three farmers. The first farmer had an unwavering belief in the power of fertile ground. She spent her days enriching the soil, ensuring it was teeming with nutrients and life. The second farmer dedicated her time to tending the weeds. She understood that without proper care, weeds could choke the life out of their valuable crops. This left the third farmer to focus on planting better vegetables, using carefully selected seeds from the most robust and flavorful vegetables in the region. With hard work, the farmers found that the harmonious combination of their efforts led to the most abundant and delightful harvests year after year.
At Ochre Bio, a liver RNA therapeutics company that uses AI extensively, we celebrate all three farmers. Or, in our case, the three types of scientists who produce and innovate with data. We encourage those, who like the first farmer, place their faith in the evolving genomic and imaging technologies as fertile ground from which bigger and better data will emerge. New technologies allow us to ask better questions, but they are of little use if the data are not sufficiently clean. We also support our unsung heroes, the statisticians and bioinformaticians who, akin to the second farmer, obsess about what it takes to clear out the weeds. Finally, we make a very special place for those who take on the tough task of producing their data from the best possible sources. It’s this third farming endeavor that we’ll focus on for the remainder of this article.
The third farmer: generating quality data from quality sources
In a 2023 article, Aaron Daugherty, Vice President of Discovery at Aria Pharmaceuticals, offered some candid insight. He pointed out that, though AI excels in streamlining processes and accelerating timelines, it doesn't solve the “real” problem: the complexity of human disease (5). How then do we generate causal human data of sufficient complexity? How do we increase the use of humans as the model?
Human genetics has been heralded as a beacon of success, linking DNA variation to population health (9). However, genetic data falls short of answering crucial biological questions, such as what cell type a gene is active in, and at what point it is relevant in a chronic disease’s trajectory. It also doesn’t guarantee reversibility – the extent to which the effects or progression of a disease can be halted, diminished, or completely undone, through therapeutic targeting of a gene. To address these shortfalls in studying human liver disease, we have set up liver labs in Europe, Asia, and the US.
Our lab in Oxford takes different types of human cells from donor livers, which they combine to build components of the liver from scratch. These “bottom up” models allow us to flexibly study the metabolic, cell death, inflammation, fibrosis, and neoplastic processes that occur throughout the liver disease trajectory. Our lab in Taipei is the only lab in the world that receives biopsies of diseased human livers, turning each biopsy into about 50 slices that we culture in the lab as diseased micro-livers. If testing a potential therapy on these “top down” models generates promising results, we then test directly on human livers kept alive on perfusion machines in our New York lab. We call this our “Liver ICU” where each human liver is studied over many days. We are, in effect, trying to do the clinical trial before the clinical trial.
The role of AI in human data
Bringing human data earlier into the drug R&D process isn’t a new idea. Population genetics has enjoyed considerable attention in recent years, and the FDA has also lent weight to the idea, with the recent FDA Modernization Act no longer requiring animal data to support human clinical testing (10). For the first time in modern drug research, we can get a drug to patients solely using human data.
What then is the role of AI under this new status quo, where we use humans as the model? An approach adopted by some impressive techbio companies has been to use AI to automate and enable scale. In large robotic labs, human cells and organoids are cultured in what are sometimes called “unbiased screens.” Here, unbiased refers to genome-wide perturbations to ensure that every target is tested. However, unfortunately, there’s no such thing as an unbiased screen. Choosing to be less biased in one way often means being more biased in another. The “bias” such genome-wide approaches often face is that, to achieve this brute force scale, the compromise is model simplicity. If Daugherty is correct, and human complexity is the real problem, we need to consider whether such compromises are sometimes too much.
We want the complexity of primary human cells, tissues, and organs. We also want to achieve scale with causal (perturbation) studies without wasting effort on the vast majority of targets that will have little effect. We see the role of AI as that of enabling adaptive screens, akin to adaptive clinical trials. Humans begin with testing a few hundred targets. Algorithms analyse the data, looking for explanations as to why some targets have worked and others haven’t. Algorithms then recommend the next iteration of genes to test.
At a time where there can be fear mongering about AI supplanting scientists, I see the interaction between humans and algorithms as being more collaborative: a flourishing partnership fueled by good data from our celebrated farmers.
- JP Hughes et al., “Principles of early drug discovery,” Br J Pharmacol, 162, 1239–1249 (2011). DOI: 10.1111/j.1476-5381.2010.01127.x.
- N. Fleming, “How artificial intelligence is changing drug discovery,” Nature, 557, S55–S57 (2018). DOI: 10.1038/ d41586-018-05267-x.
- WHO, “Noncommunicable diseases,” (2023). Available at: www.who.int/news-room/fact-sheets/detail/noncommunicable-diseases.
- CDC, “Health and Economic Costs of Chronic Diseases,” (2023). Available at www.cdc.gov/chronicdisease/about/costs/index.htm
- A Daugherty, “Artificial intelligence: a great crash of hype into reality,” Drug Target Review, (2023). Available at: www.drugtargetreview.com/article/108086/artificial-intelligence-ai-a-great-crash-of-hype-into-reality/
- J Montague, “AI‘s Role in Drug Discovery: Separating the Hype from the Hope,” HIT Consultant (2022). Available at: hitconsultant.net/2022/11/03/ai-drug-discovery-hype-hope/
- Wellcome Trust, “Unlocking the potential of AI in Drug Discovery – 2023,” (2023). Available at: cms.wellcome.org/sites/default/files/2023-06/unlocking-the-potential-of-AI-in-drug-discovery_report.pdf
- C Auffray et al., “Making sense of big data in health research: Towards an EU action plan,” Genome Med 8 (2016). DOI: 10.1186/s13073-016-0323-y
- R Qureshi et al., “AI in drug discovery and its clinical relevance,” Heliyon, 9 (2023). DOI: 10.1016/j.heliyon.2023.e17575
- Drug Discovery World, “What does the FDA animal testing legislation mean for drug discovery?” (2023). Available at: www.ddw-online.com/comment-what-does-the-fda-animal-testing-legislation-mean-for-drug-discovery-21746-202302/
Quin is a medical doctor with further degrees in genetics, mathematics, computational biology, and a doctorate in systems genomics, from Oxford and Cambridge Universities. He started his first drug discovery liver genomics company 17 years ago. More recently he founded and led Novo Nordisk’s Advanced Genomics Department, again focused on liver disease. Frustrated with the lack of therapeutic innovation in chronic diseases, Quin co-founded Ochre Bio, a liver RNA therapeutics company with global R&D sites in Europe, Asia, and the US.