Humans and Machines: Exploring the Reaches of Chemical Space
Machine learning has significant promise, but we should never overlook the importance of the human factor.
Ryan Walsh, Ying Zhang | | 6 min read | Technology
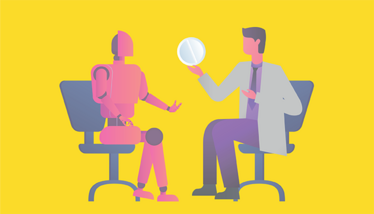
Machine learning (ML) approaches are enhancing the efficiency and success rate of chemical space exploration in drug discovery, but from our perspective, the most successful programs will also play to human strengths.
Although not a stand-alone drug discovery approach, because it relies on experimentally generated data, ML can augment existing drug discovery approaches, such as high-throughput screening, virtual screening, DNA-encoded chemical libraries and fragment- and structure-based methods. When it is implemented strategically, the results are clear. For example, Verge Genomics’ VRG50635 is in phase I clinical trials for the treatment of amyotrophic lateral sclerosis (ALS), and Insilico Medicine’s INS018_055 is in Phase II clinical trials for the treatment of idiopathic pulmonary fibrosis. Both were uncovered by AI-driven drug discovery.
But with all the recent excitement around ML, it is important to emphasize that the human factor should not be eliminated from the iterative design of compounds, data curation or benchmarking. A chemist should always have the final say in the prioritization of ML-generated compounds, so that the focus on progressing high-quality chemical matter can be maintained as our computational capabilities evolve. We remain responsible for supplying high-quality data and exercising scientific rigour when drawing conclusions from our experiments.
One inherent advantage of human expertise is our capacity to think critically and consider problems in a broad context. The best ML models might pass a Turing test, but will generally be less accurate in extrapolating beyond the corpus of data on which they were trained. On the other hand, ML models are better suited to comprehend and leverage complex relationships within large datasets holistically than a human brain.
The need for general awareness regarding the distinct advantages humans and machines offer is important, but so is the need for robust processes for both parties to exchange information with each other. The responsibility falls to us in both cases. We must tailor our descriptions of molecules to the ML models to incorporate as much context as possible. We are also responsible for querying the ML models and predictions for relevant information and interpreting it sensibly in our decision making.
The impact of diversity
With the abundance of data at medicinal chemists’ fingertips, rational design decisions have become more difficult. The breadth of chemical space we can access is expanding exponentially faster than we can design, make, test, and analyze chemical libraries, so our intuition regarding the opportunity cost of each new library is now less clear than before.
We can mitigate this by leveraging domain knowledge and computational tools, including ML, to inform and prioritize focused library designs according to an objective (e.g. maximizing diversity relative to an existing library deck, or modulating a particular biological target class).
“Diversity” itself is not a well-defined term and, therefore, difficult to measure, even in a relative sense. The underlying motivation behind a focus on diverse library designs is to cast a wide net into chemical space and then delve into promising chemotypes, which inherently assumes the similarity principle. But as with many rules, there are exceptions (activity cliffs are one example). Furthermore, each chemical representation and similarity score has its own merits and limitations, but none are universally reliable in the measurement of similar bioactivity. So, by extension, none are universally reliable in measuring library diversity in a relevant context, either.
The development of molecular representations which localize bioactive compounds in chemical space and similarity metrics which are consistent with medicinal chemists’ intuition are expected to have a positive impact in addressing these concerns.
Predictions and promises
Using ML, predictions about the safety and efficacy of promising chemical matter can be made at the “design” stage rather than the “analyze” stage. The predictive power of the current state of the art is continuously improving, so the value offered by these early-stage predictions continues to increase. Substructure filters can be particularly helpful to weed out molecules that can potentially cause in vivo toxicity, instability, assay interference or synthesis challenges.
It is also important to remember that ML does not replace experimental measurement of endpoints related to safety and efficacy. It could be used to provide estimates beforehand, but the measurements themselves will (and should) always be part of the approval process. If ML is deployed complementarily to other drug discovery approaches, it can discover chemical matter that would not have been found by those other approaches, so we expect it to have a positive impact on the safety and efficacy of future drug candidates overall.
Looking toward a collaborative future
Current innovations are likely to have a profound impact on the discovery of future therapeutics. For example, improved assessment of the synthesizability of ML-generated compounds can enable drug-likeness estimates, retrosynthesis prediction and generative compound design. The latter is not yet as mature as predictive modeling approaches, but we anticipate that its impact will grow over time.
We can also leverage explainable AI approaches to query the ML models for which information they found to be most influential in making their predictions. Peering into the “black box” helps us understand the model’s perspective on the underlying chemistry, which can reveal new insights, such as the putative pharmacophore within each molecule. Confidence estimates are also helpful in assessing how “sure” the model is about each prediction, so we know which ones to take with bigger proverbial grains of salt.
We would like to emphasize a common sentiment in the ML community: the practice of sharing datasets and code for any public communications describing nonproprietary advances is not only encouraged, it is of the utmost importance. The ability to reproduce results is critical to the scientific method, and the transparency and accountability that stems from that facilitates our advancement as a community.
Data validity should also be checked and communicated consistently. Unfortunately, many datasets in the public domain, such as patents, are of low quality. To avoid propagating that pattern, dataset preparation and any processing steps should be documented wherever possible.
The standardization of benchmark datasets for common ML use cases remains crucial as well. These already exist for certain use cases, such as the Therapeutic Data Commons (TDC) for absorption, distribution, metabolism, excretion, and toxicity (ADMET) prediction, and the Open Reaction Database (ORD) for reactivity prediction. We hope that this trend continues.
Overall, there is a great deal of hype in the scientific community around AI and ML, which should only be perpetuated with appropriate care and diligence. It’s an exciting time for the community, and we should celebrate advances where we can. Equally, we should not lose focus on the concrete value offered by AI and ML in the broader context of drug discovery, which is driven by, and for, people.
The task at hand for drug researchers is striking the right balance between AI/ML and human intervention. The most successful drug discovery programs will play to the strengths of both, for the benefit of all.
Senior Research Scientist, X-Chem, Waltham, Massachusetts
Vice President, Chemistry, X-Chem, Waltham, Massachusetts