Embracing the Digital Enterprise
Today’s pharmaceutical manufacturing operations capture huge amounts of data. Unfortunately, in many cases the data pass by without actual context. How do we obtain the digital “Holy Grail” – a fully mapped process that facilitates decision-making using data we trust?
Bob Dvorak |
We recently visited the process development group of a large pharmaceutical company with a colleague to gather information about process analytical technology. We asked the head of the group to describe some of his challenges around data. He repeated the well-worn refrains heard in many companies: old technologies and lack of integration. Then he said something that stuck with us: “I feel like I am looking through a knothole in a fence, watching my data go by. There is no way to understand the upstream and downstream context or to interact with it.”
This is exactly the problem with most data strategies in bio/pharma manufacturing; though they typically show specific data with absolute clarity, the context and connection for the data to be truly useful is missing.
Today, the biopharmaceutical industry captures massive amounts of data about its products and the processes that allow them through its well-established automation systems, data historians, document controls and manufacturing analysis systems. Each of these is an ‘island of data’ that is integrated as and when needed to ensure execution continuity. This point-to-point integration is OK for many of these systems since it solves the short-term goal of manufacturing product, but does little to address the broader questions that a decision-maker may have:
- Where are the critical places in the process that are the least robust?
- How much is this downtime costing us?
- How much material should I make based on current demand?
- Which of my plants is currently making this product most efficiently? And why?
The lack of a central source of process context leads to data gaps about the overall manufacturing process.
When ‘something’ (a problem or unexpected event) occurs in the process, those data gaps result in an inevitable meeting request in your calendar. People who understand the process are brought together and data from multiple sources is reviewed and queried. The hope is that the individuals in the room will somehow recognize the data they are watching and be able to make sense of it in terms of the process. In fact, each expert is “looking through the knothole” at data, seeing specific pieces of information without understanding them in a broader context. Having enough eyes on the problem can make it possible to find patterns, but that means the involvement of many people every time a problem occurs. And even then you may not always be able to spot the issue. There is a better way.
Adding digital context
We have to change the way we think about data. Importantly, we must close the gaps that exist between the traditional ‘islands of data’ and instead focus on creating a richer context for our process data. This context is captured not just in a series of meetings, but in a formal model of the manufacturing process that tracks relationships, business rules, equipment and resources. This model is designed by the subject matter experts who understand the process, and their meetings should occur long before ‘something’ happens. These experts can build the process, identify the key data that needs to be collected, and visualize that process directly.
The approach also avoids unnecessary customization of the system and ad-hoc queries (which encourage rigid business processes) and instead focuses effort, data and automation in the areas that need it the most. We call such an effort the digital enterprise.
A central driver of the digital enterprise is better and faster decision-making. Whether to better embed quality into the process (Quality by Design) or enable continuous improvement, data needs context, which must be based on sound process understanding. The digital enterprise builds on the automation systems you already have in place and allows users to see how a change in one area of the facility cascades through other processes and areas via a complex set of relationships and business rules. Identifying the pattern of cause and effect up front enables the business to see the impact of a change or adverse event not just on a single manufacturing area, but on overall metrics like adherence to plan and cost per batch.
The foundation of the digital enterprise starts with capturing your process knowledge to create an overall ‘map’ of the process that provides context for data. The map gets populated with data from your manufacturing runs, with knowledge from your experts and, coupled with your historical data, provides a foundation of certainty about what you will be seeing. This approach allows you to tunnel into the unit operations in a meaningful way: not mining data, but populating process models with actual numbers.
Accuracy and logic are needed to correctly model the dynamics of the system being studied, which requires a formal methodology. For example, Schruben’s model accreditation protocol can be used to ensure that the model is an accurate reflection of plant operations. This model relies on the judgments of a team of experts who need to distinguish simulated system performance from actual system performance. The experts must then use deep process knowledge and experience of many batches or experimental runs to refine the models and create the basis for confidence in the data.
Shifting the lens
We are all familiar with the current ‘big data’ trend - analyzing massive datasets for trends that can be used to the organization’s advantage. The challenges of ‘big data’ arise when the data sets are external, uncontrolled and unstructured, and must be gathered and processed. In manufacturing, that data is created and structured by you, so an understanding of the decisions you want to make should drive your data strategy.
In the old model of data analytics, the ‘lens’ of analysis is only typically applied when a decision needs to be made (Figure 1).
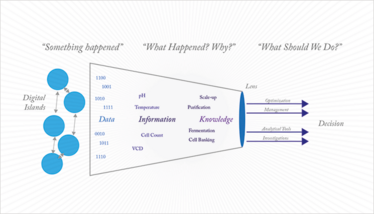
Figure 1. The old model of data analytics
Data is collected and brought together in a painstaking manual process. At each stage, individuals attempt to ‘strip down’ the quantity of information into manageable streams that can be understood. That information is interrogated to turn it into knowledge that can be used to make a decision. While this traditional approach does (eventually) get decisions made, the transformation of data into information into knowledge usually requires active work, for example, looking at reports and interpreting them.
However, since knowledge only has value to the organization when it enables actionable decisions, the next step must be to look at how decisions themselves are managed. Modern manufacturing intelligence systems should model the knowledge you have about your products, processes, and resources. Then they should gather data from the fully digitized enterprise that provides current information (Figure 2).
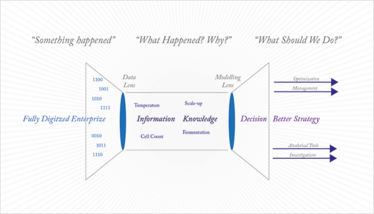
Figure 2: The fully digital enterprise
This concept transforms the way we look at data. Knowledge isn’t pulled from information: it is modeled on an understanding of the way we manufacture the product. Information isn’t extracted from data: it is the way that data is structured. Now, the lens of analysis moves right up to the data itself. It is not the ‘knothole in the fence’ through which data is observed, but the real-time measure of a well-understood process – placed in a context or map of that process. Data becomes a snapshot of performance rather than something to be translated.
The real power to your organization comes when both the operator at the terminal and the manager on the floor have a device, such as a smartphone or tablet, that provides an instant view of data in a format familiar to them. With that process visualization, events can be addressed immediately and decisions can be made with minimal delay. The ability to go beyond individual events and to instead see trends helps to make those decisions progressively smarter. The more knowledge grows, the more decisions are informed and vetted. A key element is the certainty that the decision is being based on a full picture, with the complete context well-defined.
When ‘something’ happens, it is an anticipated event.
Escaping ‘average’
Ultimately, the real power of the digital enterprise comes from managing the ordinary variations that occur in bioprocessing. The digital enterprise, based on the model of the process, allows us to escape the fallacies that arise from the law of averages. Think about a bioprocessing plant like a drunken man walking down the middle of a busy road. He staggers right and left, but on average, he is walking the median. In the theoretical model, he makes his way safely along the road and stays alive. In practice, he wanders off the median and gets hit. He is dead in practice.
Older models have to focus on the ‘average’ batch because they lack the defined process and complete data set to actually track the variations. In the digital enterprise, you can predict based on the past, react based on the present, and get smarter in the future. Systems can react to the anticipated event. People can react to the unanticipated event with immediate visualization of the data they need to make their decisions. Then those decisions become part of the overall knowledge base and that unanticipated event becomes an anticipated one.
The Digital Enterprise: Defined
- All business processes captured in digital systems.
- Data modeled on the business process.
- No gaps in the flow of data between elements of the business.
- Visualization tools that show data in real-time and in context.
- Mobile and workstation access to data in the context of the operator or supervisor viewing the data.
- Combined data views of how things should work, how they are working, and what this means for the profitability of the business.
- Fast decision-making, based on data you trust to be complete and accurate.
Total disruption of the current ‘Islands of Data’ approach is critical. Those islands must be connected together by interfaces and reports to obtain a fully mapped process that enables rapid decision-making based on data that you trust – that’s the ‘Holy Grail’!
When Indiana Jones goes through his trials to reach the ‘Holy Grail’, he has to take a leap from the lion’s head – a leap of faith. He sees the chasm in front of him and he knows he must continue. And yet there is no way he can clear the gap. He closes his eyes, takes a deep breath, and then takes a step forward and finds that he is on solid rock. No leap was required because the gap never really existed. The digital enterprise is about having your team step onto solid rock instead of making some wild leap across the chasm of data.
PhD is Principal at BioPharma Data & Manufacturing Systems Consulting, San Francisco Bay area and Rick Johnston, PhD is founder and CEO of Bioproduction Group, Berkeley, CA, USA.