Embracing the Digital Era
From LIMS to AI, connected technologies are becoming a strategic initiative for an increasing number of smart companies
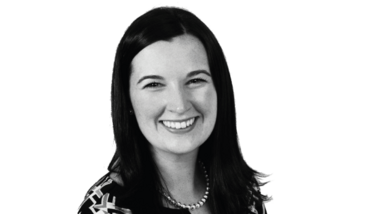
Substantial evidence of pharma’s shift towards digitization can be seen by the increasing number of “chief digital officer” appointments or similar across big pharmaceutical companies as they look to strategically boost digital capabilities. Advanced digital technologies, such as laboratory information management systems (LIMS), the Internet of Things, artificial intelligence, and machine learning, with its subset, deep learning, are all becoming hot topics in the industry.
One easy win: using connected technologies to improve data management. The colossal volumes of data generated during drug discovery and development are often siloed, hindering the progress of pharmaceutical innovation. For example, most raw data is typically stored in databases or shared network folders, remaining accessible to only one part of the organization. This inaccessibility creates inefficiencies for data retrieval and analysis, limits company transparency, and slows collaborative efforts — and the problem is only amplified by data volume and complexity. The root of this problem can be tracked back to laboratory instruments and software systems that are not connected. Storing data from a range of instrumentation in one central LIMS location and then integrating the LIMS with other connected systems is a far more efficient approach to data management. LIMS can be augmented by machine or deep learning capabilities, reducing the time spent extracting, aligning, and analyzing the data. In turn, drug developers should be able to answer complex questions faster, accelerating innovation.
However, digital technologies are also being used in more advanced ways – with some drug companies built entirely on the basis of AI approaches to drug discovery. For example, Cyclica uses AI in their polypharmacology approach that designs and evaluates drug candidates based on all potential interactions – rather than the typical single-target focus approach to screening (1). Meanwhile, Exscientia’s AI algorithm has enabled the identification of a promising molecule targeting a novel pathway to treat chronic obstructive pulmonary disease (2). A mere 12 months was needed to reach this milestone – an incredible achievement. AI could potentially drastically shorten the drug discovery phase and identify more promising drug candidates earlier, thereby reducing failures and costs.
Machine learning and deep learning are also being used to mine large amounts of literature and databases, such as drug screening data, to identify patterns. Whereas humans are prone to bias and preconceived ideas, machine learning classifiers can take a more global view. Post-approval studies can benefit from the same approach. The timely detection of adverse events and potential patient safety concerns is paramount, and relies on pharmacovigilance experts and labor-intensive processes. Digital ecosystems and deep learning analyses are being developed to facilitate better data mining to highlight safety insights that might not be observed otherwise.
Advanced digital systems have the potential to transform the pharma industry in many ways. Once considered “nice-to-have,” digital technologies are now becoming a strategic imperative for companies that want to remain competitive.
- DH Friedman, “Hunting for New Drugs with AI,” Nature, 576, s49-s53 (2019).
- BenchSci Blog, “43 Pharma Companies Using Artificial Intelligence in Drug Discovery,” (2017). Available at https://bit.ly/3njgVNQ.