Model Manufacturing
Our ‘dynamic flowsheet model’ links API purification and processing with downstream tablet manufacturing to provide better control of the process variables and, ultimately, the critical quality attributes of the final pharmaceutical product. Here’s how.
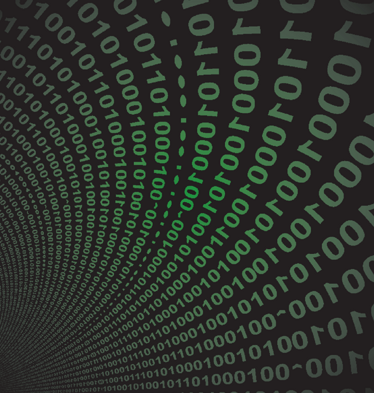
The advantages of continuous processing schemes have been discussed by many in the industry (1-3), but the US FDA’s stringent regulatory guidelines have somewhat hindered implementation. Despite this, progress is being made and the pharma industry is slowly moving from batch to continuous processing. As a result, it is necessary to have a clear understanding of the process dynamics and to develop suitable tools to help with the process design analysis. We believe that modeling and simulation can aid the transition.
A well developed, tuned, calibrated and validated model can be an effective tool in order to study the process dynamics or perform design, optimization and control studies. For instance, mathematical models can be developed that capture the dynamics of active pharmaceutical ingredient (API) powder processing operations, allowing for virtual experimentation and validation of new methodologies prior to implementation in the real plant. Design or optimization based studies require multiple trials; using a model instead of conducting several experiments will help to save resources.
In this article, we describe the development of our dynamic flowsheet model, which connects API purification and processing with downstream tablet manufacturing. The starting point for our work was the observation that the physical properties of API crystals have a wide-ranging influence on the critical quality attributes of the final dosage form. So, if we can establish a connection between API purification, processing and tablet manufacturing steps then it should be easy to control the process variables and obtain a final dosage form with the right critical quality attributes. The powder flow phenomenon is difficult to predict because of its inherent variability, so real-time feedback control and monitoring techniques are also important, and emphasized in the FDA‘s process analytical technology (PAT) framework (4, 5).
Our model, the first of its kind, incorporates a multi-scale framework that stores information from different levels or scales. For example, in the case of powder flow, the particles are considered as discrete entities that can interact with each other and with the equipment boundaries in different ways. A discrete element modeling technique can simulate the flow pattern of each and every particle, and provide particle-level information that will be used in the process model to obtain important process variables. The advantage of using a multi-scale framework is that it helps to capture process dynamics more efficiently. In addition, we’ve developed a robust control strategy for the process. This model will help to clarify the effect of upstream process parameters on the critical quality attributes of the downstream product.
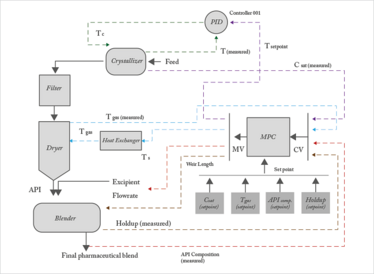
Figure 1. Closed-loop integrated flowsheet model. API: active pharmaceutical ingredient; MPC: model predictive control

Figure 2. Multi-scale framework. API: active pharmaceutical ingredient; CSD: crystal size distribution; RSD: relative standard distribution; MPC: model predictive control; PID: proportional-integral-derivative.
Essential integration
The integrated process model links crystallization, filtration, drying and mixing in a continuous manner. Figure 1 presents the schematic of the flowsheet including the control loops, which we will discuss in the next section. We developed process models of these unit operations from first principles and then established a continuous connection between them.
The crystallization model is that of a cooling crystallization – as the temperature falls, the solute precipitates out in the form of nuclei, which grow to form crystals of different sizes. As the crystals are formed, the liquid solvent forms a thin layer on the crystal surface and often gets lodged within the crystal pores. The crystal size distribution (CSD) is a function of the nucleation and growth rate kinetics, which in turn are controlled by the cooling profile (the pattern in which the temperature is reduced over time), so changing this profile also changes the CSD.
CSD can have a considerable impact on mixing efficiency, so we used the CSD obtained from the crystallization process as an input in a discrete element method, which predicts the behavior of large numbers of small particles, for the continuous mixing model. The discrete element method simulations give the average particle velocity at specific locations inside the mixer as an output, which is then fed into the mixing process model. Our crystallization and mixing process models were both developed on the basis of a population-balance model methodology. Figure 2 shows the schematic of the multi-scale framework.
The output of the crystallizer, a slurry of API crystals in the mother liquor, is connected to the input of the filter and the crystals are removed from the solvent. The crystals form a solid cake on the filter medium and, when dislodged, enter the dryer. The stream of crystals entering the dryer are of a particular size and contain considerable liquid content, as determined during the crystallization step. Although there is some size change associated with the drying process, it is quite minimal, so we can ignore it for the purposes of our model.
Once the API crystals are separated, purified and dried, they are sent to the mixer where they are mixed with an excipient to obtain the final pharmaceutical blend. The output variables from the mixing model are the variability of the product, expressed as relative standard deviation, and API composition of the blend. You can read more about the mathematical equations and multi-scale flowsheet model in our previous articles (6, 7).
Process control
We also developed a hybrid model predictive control (MPC) – proportional-integral-derivative (PID) control scheme for our flowsheet model (8). Control loops were developed for each of the unit operations, but we used a constant pressure gradient for the filtration process (by assuming an ideal controller that can achieve the desired set point perfectly). In practice, the pressure gradient can be maintained by adjusting the flow of air or exhaust across the filter medium (9, 10).
The cooling temperature schedule is the critical process variable in crystallization and here we used a cascade control scheme (consisting of a slave and master controller). The master controller generates the set point of the temperature cooling schedule for the slave controller, and the temperature schedule is then achieved by varying the temperature of the cooling water that passes through the cooling jacket. We demonstrated the cascade loop with the aid of a hybrid MPC-PID design, where MPC is the supervisory controller, used to control the saturation concentration, and PID is the secondary controller, used to control the operating temperature. The saturation concentration of the solute is measured and fed to the MPC block, which generates the set point for the operating temperature controlled by the PID. In many instances, a cascade control loop has better performance than a single-loop control; for example, when there are long dead times; or when a disturbance affects an intermediate variable, causing a knock-on effect on the main control variable (11, 12).
For drying, the temperature of the drying medium, which in our case is air, is the critical control variable. Air at atmospheric temperature can be heated to the desired temperature by using superheated steam in a heat exchanger prior to it being sent into the dryer. For the mixer, there are two control variables of interest: fractional API composition of the final pharmaceutical blend and holdup. Mixer holdup is an important control variable as it can have a considerable effect on the product’s variability. We controlled holdup by manipulating the weir length, having previously determined the effect of the weir length on the holdup by running discrete element method simulations of the mixing operation.
As mentioned earlier, the control scheme is a hybrid - we used an MPC-PID hybrid control loop for the cooling profile of crystallization operation and the drying gas temperature; while API composition and holdup have been controlled with the help of MPC only. If you’re interested in the design strategy of the hybrid control system, you can find details in a previous article (7).
And the results?
We evaluated the control loop both in terms of set point tracking and disturbances rejection. Disturbances rejection refers to the ability of the system to bring a process variable back to a fixed value – a bit like cruise control in a car. Set point tracking is the ability to adjust a variable to a changing value – say, when the driver increases the cruising speed. A step change was applied to the set point to evaluate the set point tracking ability, and sinusoidal disturbances were introduced in the input signal to study the disturbance rejection. Using these two tests, we compared the performance of the MPC-PID control scheme with that of a PID (regulatory controller)-only scheme, with positive results. MPC-PID scheme stabilizes faster and has a high decay ratio, which is always a good thing for a controller. Looking at disturbance rejection for crystallization, again the hybrid scheme performs better. The same pattern was seen when looking at set point tracking and disturbance rejection for the drying gas temperature. Similarly, the performance has been evaluated for the mixing operation; once again the MPC scheme is better than the PID scheme.
Next steps
This integrated continuous flowsheet model, developed from first principles, connects the API purification and processing steps with a downstream tablet manufacturing operation and can be used as an effective tool to study the impact of the upstream process parameters or API properties on the efficiency of the downstream operation. The next steps will be to extend this flowsheet further by adding other operations for downstream processing (e.g., granulation, roller compaction, tablet compaction and tablet dissolution) for a complete quantitative analysis of continuous purification and downstream processing of solid dosage forms.
Maitraye Sen, Ravendra Singh and Rohit Ramachandran are from the Department of Chemical and Biochemical Engineering, Rutgers, The State University of New Jersey, Piscataway, NJ, USA.
- G. V Reklaitis, J. Khinast and F. J. Muzzio, “Pharmaceutical Engineering Science – New Approaches to Pharmaceutical Development and Manufacturing”, Chem. Eng. Sci. 65, 4 –7 (2010).
- A. Gorsek, P. Glavic, “Design of Batch Versus Continuous Processes: Part I: Single-Purpose Equipment”, Chem. Eng. Res. Des. 75, 709–717 (1997).
- H. Leuenberger, “New Trends in the Production of Pharmaceutical Granules: Batch versus Continuous Processing”, Eur. J. Pharm. Biopharm. 52, 289–296 (2001).
- Food and Drug Administration, “Guidance for Industry. PAT-A Framework for Innovative Pharmaceutical Development, Manufacturing and Quality Assurance”, www.fda.gov (2004).
- Food and Drug Administration, “Guidance for Industry. Q8 Pharmaceutical Development”, www.fda.gov (2006).
- M. Sen et al., “Multi-Scale Flowsheet Simulation of an Integrated Continuous Purification– Downstream Pharmaceutical Manufacturing Process”, Int. J. Pharm. 445, 29–38 (2013).
- M. Sen, R. Singh and R. Ramachandran, “A Hybrid MPC-PID Control System Design for the Continuous Purification and Processing of Active Pharmaceutical Ingredients”, Processes 2, 392–418 (2014).
- M. Sen et al., “Flowsheet Optimization of an Integrated Continuous Purification-Processing Pharmaceutical Manufacturing Operation”, Chem. Eng. Sci. 102, 56–66 (2013).
- A. Robles et al., “Advanced control system for optimal filtration in submerged anaerobic MBRs (SAnMBRs)”, J. Membrane Sci. 430, 330–340 (2013).
- R.H. Peiris et al., “Fouling Control And Optimization Of A Drinking Water Membrane Filtration Process With Real-Time Model Parameter Adaptation Using Fluorescence And Permeate Flux Measurements”, J. Process Contr. 23, 70–77 (2013).
- R. Singh, M. Ierapetritou and R. Ramachandran, “An Engineering Study On The Enhanced Control And Operation Of Continuous Manufacturing Of Pharmaceutical Tablets Via Roller Compaction”, Int. J. Pharm., 438, 307–326 (2012).
- R. Singh et al., “Closed-Loop Feedback Control of a Continuous Pharmaceutical Tablet Manufacturing Process via Wet Granulation”, J. Pharm. Innovation 9, 16–37 (2014).