Side Effects and Supercomputers
A group of researchers have used supercomputers to link proteins to drug side effects (1). Could this computational model be more predictive than current statistical methods?
Tell us a bit more about the study.
This project was done under the auspices of an internally funded basic R&D effort to use multi-scale modeling. The aim was to combine the results of large-scale, atomic-scale, physics-based, structural modeling of the interactions between proteins and small molecules done by Felice Lightstone’s computational biology group on supercomputers, with publicly available data on side effects in approved drugs. We wanted to see if we could come up with better methods of “red-flagging” candidate drug molecules for potentially lethal adverse reactions. Along the way, we also considered interactions at the level of biological pathways, creating pharmacodynamic models of drug metabolism.
But, the main goal was to see if we could, through computational modeling, develop a cost-effective, reliable way to identify potential side effects as early in the drug development cycle as possible.
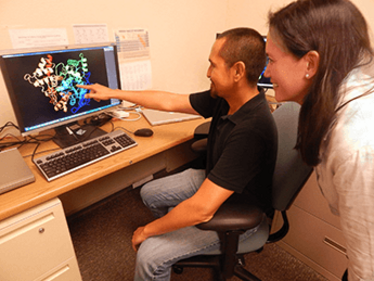
How are the supercomputers used to predict side effects?
A very talented postdoc in Felice’s group, Xiaohua Zhang, did some work on parallelizing the widely used AutoDock Vina molecular docking software package. The Lawrence Livermore National Laboratory version is named VinaLC (2). MPI and multi-threading was used so it could be parallelized on petabyte-scale high performance computing machines, in other words a single docking calculation is one process and thousands run simultaneously. The performance scales up so that one million flexible compound docking calculations can be done in 1.4 hours on 15k CPUs. VinaLC is downloadable at http://bbs.llnl.gov/tools.html.
We applied this software to perform docking calculations on 960 small-molecule drug compounds to 409 human proteins. The docking calculations scored how well a given drug would bind to protein. In a separate calculation, these docking scores were combined with side effect data on a subset of 560 of the drugs – using the publicly available post-market package insert database SIDER. Finally, we applied machine learning algorithms and performed other statistical analysis to establish correlations between particular adverse drug reactions (ADRs) and proteins.
It should be noted that the linkages we identified were largely off-target protein effects –only two percent of the 409 proteins we considered were on-target proteins for the 560 drugs. For a new drug compound, docking the new drug to the protein panel could identify ADRs. For example, if that drug binds to a protein strongly associated with a particular side effect, then that should flag that compound as having a non-zero risk of having that side effect.
How does it compare with other prediction models?
The primary goal for this study was to establish a proof-of-concept prototype to show that combining drug/off-target protein binding data derived from HPC binding calculations with population-level epidemiological data on drug-side effect linkages can provide additional prognostic information relative to just using publicly available, known target data of the type found in the DrugBank database. To accomplish this, we built two distinct sets of ten models where each model predicted for a different ADR group, such as ‘cardiac disorders’ and ‘renal disorders’.
The first set was a ‘control’ group of models trained only with SIDER side effect data and DrugBank target data on the 560 drugs. The second set of models was trained on the SIDER data, but used the largely off-target Vina LC docking scores computed on HPC instead. We used the area-under-the-receiver-operator character curve (AUC) statistic to evaluate the models.
For the DrugBank control group, we obtained a range of AUCs of 0.61-0.74 across the ten ADR groups, and for the VinaLC-trained, “off-target” group we obtained a range of AUCs of 0.60-0.69. Clearly, the DrugBank models and the VinaLC-trained models are very comparable. For two of the ADR groups, ‘vascular disorders’ and ‘neoplasms’, we obtained a range of AUCs that were higher for the VinaLC models than for the DrugBank models, showing that for these two side effects, the off-target data was more predictive of those two ADRs.
I think we are really the first group to have done this direct, apples-to-apples comparison. The AUCs are not all that great, but this was a fairly sub-optimal set of proteins and drugs for two reasons: (i) the drugs are post-market, so by selection bias exhibit little in the way of major side effects, and (ii) the proteins were not selected for their relevance to side effects per se, but rather they were proteins we could get good 3D structures from the Protein Data Bank, which are required by our molecular docking calculations. There is a lot of room for improvement.
What are the next steps?
We have published a proof-of-concept that shows our method is viable. Now, we are ready to do it for real. An initial draft of the human proteome has just been published where the sequences for the approximately20 thousand protein-coding genes are now known. The relevant (druggable) small molecular chemical space is estimated to be 1060 molecules. We would ideally like to fill in as much of the corresponding 1060-row, 20k-column drug-protein docking score matrix as we can. This is going to require basic research to develop better ways to construct and validate protein homology models, as well as 3D structures solved by non-conventional experimental methods since many of the receptors that are critical for drug-binding are membrane-bound and not amenable to conventional X-ray crystallography.
We would also like to partner with healthcare organizations that could provide access to anonymized, patient-level data, so we can incorporate demographics, risk factors and other biomarkers into our analysis. This is tremendously important since there are undoubtedly very interesting correlations between specific drugs, disease states, and other patient-factors that will help us make better models.
Last, but maybe most important, would be partnering with pharmaceutical companies that are willing to share data on failed clinical trials. We could perhaps obtain stronger association signals between particular side effects and protein-binding with compounds that cause non-rare ADR events.
What is it about this work that you found most exciting?
ADRs cause 100,000 fatalities each year and have an associated cost of $177 billion. The idea that, if we are successful, our methods might help mitigate that is very exciting. From a scientific perspective, this project is multi-scale in the truest sense of the word. I mean, we range from atomistic physics-based models to statistical analyses of population-level data! That’s a huge range in scale…
- M. X. LaBute et al., “Adverse Drug Reaction Prediction Using Scores Produced by Large-Scale Drug-Protein Target Docking on High-Performance Computing Machines”, PLoS ONE 9(9), e106298 (2014).
- X. Zhang, S. E. Wong, and F. C. Lightstone, “Message Passing Interface and Multithreading Hybrid for Parallel Molecular Docking of Large Databases on Petascale High Performance Computing Machines”, J. Comput. Chem., 34, 915–927 (2013).
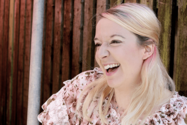
Making great scientific magazines isn’t just about delivering knowledge and high quality content; it’s also about packaging these in the right words to ensure that someone is truly inspired by a topic. My passion is ensuring that our authors’ expertise is presented as a seamless and enjoyable reading experience, whether in print, in digital or on social media. I’ve spent fourteen years writing and editing features for scientific and manufacturing publications, and in making this content engaging and accessible without sacrificing its scientific integrity. There is nothing better than a magazine with great content that feels great to read.