Smooth (Transfer) Moves
Technology transfer is full of risks. But risks can be mitigated with the right strategy – and a little help from digital technologies.
Alan Steven | | Opinion
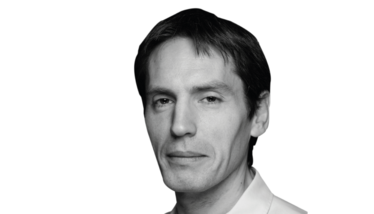
Generally speaking, technology transfer is the culmination of scale-up from pilot plant facilities to a site capable of supplying the necessary volumes for commercial supply. However, technology transfer can also occur because of a need for extra manufacturing capacity to satisfy growing commercial requirements. Thirdly, it takes place when a maturing product is moved from its launch facility to make room for newer products.
Differences in product from manufacturing campaign to campaign within the same site are inevitable. Where such a change is deemed to be statistically significant, however, a pause in manufacturing is likely, threatening the supply of a medicine to patients. The risk of such a change in quality is even greater with a change of manufacturing site, and may be attributed to scale effects, new sources of raw materials and reagents, or differences in manufacturing equipment. In my view, communication, risk management, and digital technologies all have an essential role to play in identifying and addressing the impact of these changes, and hence ensuring the success of technology transfer.
Technology transfer involves the exchange of technical knowledge, data, or procedures, and, therefore, can be considered a subset of knowledge transfer. Being inherently “sticky,” knowledge must successfully flow during the technology transfer process – it cannot be simply thrown over an organizational wall between the donating and receiving groups!
My advice when planning a successful technology transfer is to work backwards from the envisaged end goals – putting together a knowledge transfer plan that outlines the scope, roles, responsibilities, and success criteria for knowledge transfer. As well as the procedures, samples and methods, the receiving site needs to understand the rationale behind the design of the process of manufacture and associated testing.
Having clarity over what is known and what is not known about a manufacturing process makes it easier to decide what can be changed as part of the transfer. Ideally, there should be a mechanistic or statistical understanding (or a hybrid of the two) around how variation in a process input attribute (whether process parameter, material, or facility) affects the overall process or product. The most important gaps in this knowledge can then be addressed using targeted labwork or simulations, ahead of selecting a receiving site for the transfer and agreeing the changes to the process that are in scope.
As part of this assessment of knowledge gaps, it is important to capture the intricacies of the equipment needs and wants of the process, so these can be weighed versus the equipment availability at any potential receiving site. This approach forces decisions around whether to invest in the kit at the original site or to modify the receiving site’s protocols to suit existing equipment.
Technology transfer is being increasingly enabled by new technology, which can help reduce risk. For example, with mixed reality (where 3D content becomes an interactive part of the real world) scientists familiarizing themselves with the process being transferred can be granted an “I-see-what-you-see” perspective when they interact with scientists at the donor site. These experiences allow contextualized data to be shared in real time, and mediate the transfer of “tribal” knowledge that is not easily written down.
Models are another key technology; by modeling how inputs affect product quality and process performance, the behavior of the process in the modified operating environment of the receiving site can be digitally tested from the comfort of an office workstation. If necessary, thousands of simulations can be run. Taking individual models further, digital informational constructs of physical systems can be used to mirror the behavior and dynamics of physical assets involved in a process. Such digital twins can also be used as a training platform for operators and engineers, as they provide feedback in real time.
As an example of the power of modeling, I was recently involved in the technology transfer of an anticonvulsant drug used as an adjunctive treatment for partial onset seizures. Whilst preparing for the transfer, digital simulations based on a kinetic model highlighted the risk of a change in the reaction profile, on transfer, due to the impaired ability of hydrogen to move from the reactor headspace to the catalyst surface in the reactor available at the receiving site. The issue was addressed by stipulating the need to operate under a high pressure of hydrogen gas.
The experimental verification of this hydrogenation model also initially showed unexpected behavior that was traced to more sodium salts being present in an alternatively-sourced input. And that led us to refine the model, which was later successfully verified. I believe this example beautifully illustrates the need for mechanisms that continually build on the corpus of knowledge about what affects product quality and process manufacturability over the entire lifecycle of a product.
Since 2011, the FDA has openly advocated using a lifecycle approach to process validation. It’s fair to say there have been different interpretations of what this should mean, but many in the industry are aligning around the need to use data-driven and risk-based approaches to build, rationalize, and maintain the manufacturing control strategy that articulates how a process should be implemented.
In my opinion, we must continually address gaps in knowledge around what delivers a product with the right attributes, and use this as a basis for controlling or accepting risks. When this approach is taken in the context of a technology transfer preceding a process performance qualification activity, there are likely to be fewer failed batches. In turn, this means the time and cost of achieving successful registration and manufacturing commercial product will be reduced.