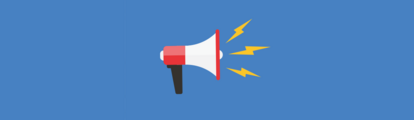
Solutions In Silico
Collaboration seeks to use artificial intelligence to predict how patients will respond to immunotherapy
Protagen AG, the National Center for Tumor Diseases (NCT) and NEC Laboratories Europe GmbH (all based in Germany) have joined forces in an attempt to improve in silico methods to predict how patients will respond to immunotherapy in malignant melanoma. The group want to develop and apply deep machine-learning to explore biomarkers for relevant endpoints – including clinical response and survival.
To find out more about how the collaboration came about and why a predictive approach to immunotherapy is so important, we spoke with the diagnostics biotechnology company, Protagen.
Why is better in silico prediction of immunotherapy responses in needed?
Cancer immunotherapy offers enormous potential for treating – and potentially curing – cancer. But there are some significant hurdles to overcome. Firstly, the approach is currently only effective for a limited number of patients; and secondly, patients receiving such treatments can experience debilitating and sometimes fatal immune-related adverse events (irAEs). Predictive models have the potential to improve the treatment and management of patients in terms of variable clinical response and irAEs.
The advantage of an in silico approach is that more complex models can be developed to help identify the likelihood of irAEs for patients, as well as which clinical feature determines these adverse events.
Why is Protagen excited about the collaboration?
Improving irAEs, clinical response and survival is a priority for cancer patients undergoing immunotherapy, and should be top of the agenda for clinicians, payers and the industry as a whole. We have been focused on biomarker development for some time and have created immuno-profiling approaches and machine learning strategies to deal with highly dimensional patient data. I really believe that machine learning efforts will increasingly influence medicine and drug discovery.
Our partnership with the NEC and NCT will bring together our collective expertise to develop and apply deep machine-learning to explore and establish biomarker models that should improve the in silico prediction of immunotherapy response in malignant melanoma.
How will establishing biomarker models helps achieve this goal?
Developing robust biomarker models can help to predict the onset of irAEs in patients undergoing immunotherapy for their cancer, which should lead to the development of more effective and safe therapies. In addition, biomarker signatures can be used to predict the overall survival of patients undergoing immunotherapies for their cancer.
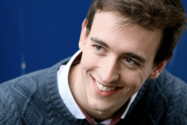
Over the course of my Biomedical Sciences degree it dawned on me that my goal of becoming a scientist didn’t quite mesh with my lack of affinity for lab work. Thinking on my decision to pursue biology rather than English at age 15 – despite an aptitude for the latter – I realized that science writing was a way to combine what I loved with what I was good at.
From there I set out to gather as much freelancing experience as I could, spending 2 years developing scientific content for International Innovation, before completing an MSc in Science Communication. After gaining invaluable experience in supporting the communications efforts of CERN and IN-PART, I joined Texere – where I am focused on producing consistently engaging, cutting-edge and innovative content for our specialist audiences around the world.