The Quest for the Golden Batch
Knowledge (or data) is power when it comes to batch-quality prediction and obtaining the coveted perfect profile
Eoghan Moloney | | 4 min read | Practical
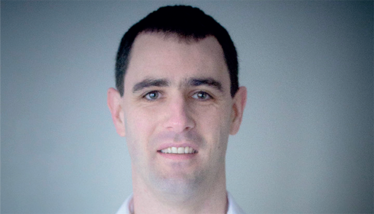
Batch quality is a critical metric – not only because safeguarding patient health is paramount, but also because poor quality affects bottom lines and profitability. The cost of a single batch deviation can be anywhere from $20,000 to $1 million per batch, depending on the nature of the product.
Until recently, the only way to analyze historical and time-series data to explore and understand batch deviations was for subject matter experts (SMEs) to spend considerable time manually reviewing spreadsheets. The SMEs would extract production data by hand, populate a spreadsheet, and create graphs. These graphs would then be used to create process parameter profiles to serve as guides for reducing process variability and increasing yield for all future batch development. In other words, creating the “golden batch profile.”
However, this manual approach is increasingly unfit for purpose and unable to help SMEs accurately identify relationships between data points. The current method presents two key issues:
- Golden batch profiles require many hours to be spent manually sifting through years of data or delayed lab results, which makes it hard to optimize process inputs to manage batch yield.
- Out-of-tolerance events will still occur, regardless of applying diligence in controlling critical process parameters (CPPs) of a recipe, as measured by a group of critical quality attributes (CQAs).
The number of variables and the cause-and-effect relationships connecting these two aspects are more complex than originally assumed. Pharmaceutical manufacturers already have the data they need to optimize their operations. What is needed is a method to analyze it all efficiently.
With this in mind, it is no surprise that a growing number of pharma companies are transitioning to advanced analytics to simplify the process of identifying their golden batch profile. New live connectivity solutions can eliminate the need for manual input into spreadsheets and facilitate data cleansing, contextualization, aggregation, and near-real-time process data analysis. A live connection between all relevant sources of data allows SMEs to minimize the time they spend collating data and aligning time stamps by hand.
Advanced analytics platforms for process manufacturing can also be integrated across every area of an organization’s operations, running in a browser with live connections to all process historians to quickly extract data to be analyzed.
Analytics in action
To understand where advanced analytics can have real-world benefits, it is worth considering a specific use case, such as examining a production process with six CPPs connected to a single unit procedure. Historical data from ideal batches with acceptable specifications on all CQAs can be easily used to graph the six variables from all the previous unit procedures. Curves representing performance from historical CPPs can then be superimposed on top of each other using identical scales to uncover new insights that can improve future performance.
Taking this approach, it is immediately clear if the curves form a tight group, or if they are spread across the graph, showing variation in values and times. Advanced analytics can easily aggregate these curves without the need for complex formulas or macros to determine the ideal profile for each CPP. Engineers can replicate this procedure to update the reference profile and boundary for each variable. The result is a better understanding of where there are opportunities to optimize processes.
For example, an upstream biopharma manufacturer harnessed the advanced analytics platform, Seeq, to study the cell culture process. With this technology, the manufacturer could create a model for product concentration based on historical batches to find the CPPs that produce the ideal batch. The company can deploy the model on future batches with golden batch profiles for all of its CPPs to track deviations more effectively and prevent them from recurring.
In another example, a manufacturer used the same technology to rapidly identify and analyze root cause analysis of abnormal batches. The team reduced the number of out-of-specification batches by adjusting process parameters during the batch, reducing wasted energy and materials – and saving millions of dollars.
Bristol-Myers Squibb uses advanced analytics alongside other technologies to capture information needed to test the uniformity of its column-packing processes. The company deploys Seeq to rapidly identify data of interest for conductivity testing to calculate asymmetry, summarize data and plot curves for verification by SMEs (1). By calculating a CPP and distributing it across the entire enterprise, all team members can operationalize their analytics, providing rapid and reliable insight as to when a column was packed correctly. This prevents product losses and quality issues – and even complete batch loss.
Whatever the use case, it is my view that advanced analytics represent the future of batch quality optimization for the pharmaceutical industry. Harnessing the latest developments in digital transformation, machine learning and Industry 4.0, advanced analytics can give a company’s engineers the insight they need to make better, evidence-based decisions. As a result, they will be empowered to go even further in optimizing the performance of day-to-day operations.
- ARC Insights, “Bristol-Myers Squibb improves chromatography and batch comparisons using data and analytics” (2018). Available here
Associate Director of Projects, Life Sciences Manufacturing at Cognizant