
Intelligence by Design
Maryam Mahdi | | 13 min read | Interview
AI is finding its place in the pharma industry and companies of all sizes and across therapeutic areas are open to the possibilities its use might bring. Here, we speak to Marcelo Bigal, President and Chief Executive Officer at Ventus Therapeutics, Jo Viney, founder, President and Chief Executive Officer at Seismic Therapeutic, and Tommaso Biancalani, Senior Principal Scientist and Director, AI/ML at Genentech, about their AI journeys and why these systems are so important to the future of R&D.
Why are you personally so fascinated by AI?

Biancalani: I’m excited by the recent progress made in the field of “generative models.” These are models that are capable of starting from a certain data sample and transforming it in a specific way. The classical examples are the filters on our phones that start with a photo of us and end by making us look older or younger. Can we do the same thing with medicine? For example, can we start from a known drug and ask the AI to transform it into a new one with fewer side effects? This would truly be game-changing in the healthcare space.
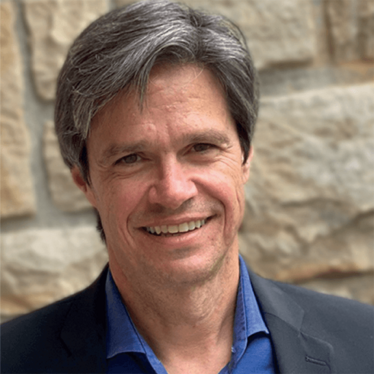
Bigal: In my view, small molecule drug discovery is an incredibly challenging pursuit that typically requires years of time, and the design and testing of thousands of molecules. Despite all the rigorous work, we frequently find that empirically identified “optimal” molecules fail in the clinic due to unoptimized potency, selectivity, or pharmacokinetic properties. The challenge of designing molecules that are fully optimized on potency, selectivity, and pharmacological properties is to get the physics right.
If we had the ability to model and determine the precise atomistic quantum physics parameters of protein-small molecule interaction, we could, in principle, design fully optimized molecules de novo with minimal chemical exploration. While this is not possible yet, I am fascinated with the opportunity to harness the power of machine learning and AI to make the dream of fully optimized physics-based drug discovery within our grasp. In theory, a physics-based AI approach could reduce the number of molecules under scrutiny from thousands to hundreds, and speed up the drug discovery process.
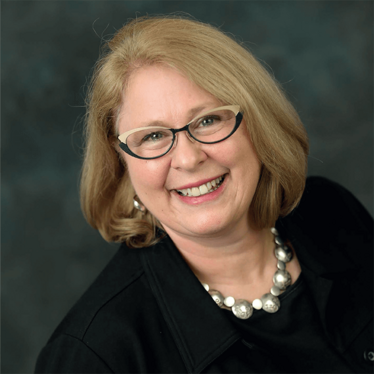
Viney: I’ve spent my entire career in autoimmune disease drug discovery – taking 13 drugs to the clinic at biotech companies. I am personally captivated by AI because I know from experience that developing drugs that target the immune system is difficult. When I began to understand the impact of machine learning to design better biologics and their capacity to advance them faster, I knew this was something I wanted to be a part of!
How has the use of AI in pharma changed over the years?
Biancalani: I see two main turning points. First, our ability to generate and store data exponentially increased in the last few decades (just consider that any pocket-portable USB stick can now contain many more books than the largest library in the world!). Second, the invention of the graphical processing unit (GPU) gave AI algorithms the power to manipulate large datasets – so-called deep learning.
Bigal: Machine learning has been part of drug discovery for more than two decades, with initial applications predicting physico-chemical properties of molecules and designing small libraries of chemical matter for a given target. In those days, lack of computational power made it challenging to widely apply these approaches to very large data sets, and also largely prevented scientists from even conceiving of other applications that we now see being developed. As Tommaso says, advancements in processing power, particularly with GPUs (driven by the gaming industry), have increased the scale of computational possibilities within practical time limits by at least 100-fold. Simultaneously, development of the deep-learning network architectures driven by natural language processing and image recognition has produced new machine-learning capabilities.
Of course, hardware and algorithm development were both required for software development, and thus together they represent a turning point in the application of machine learning to drug discovery. These advancements have led to a renaissance in computation-based drug discovery, revealing the potential for faster identification and optimization of potent and selective drug-like molecules for clinical testing.
I believe that machine learning will have its biggest impact when combined with and used to enhance novel physics-based methodologies for drug discovery and, as an industry, we are aggressively pursuing novel methodologies and algorithms, with the goal of ultimately identifying drug-like molecules completely in silico.
Viney: With all of the many steps and complexities in the drug development process, it has taken some time for drug developers to figure out how to integrate AI and machine learning within the process. AI is an amazingly powerful technology, but it does not stand alone from other analyses, experiments and insights from expert scientists that are key ingredients to discover and develop new medicines.
What are the biggest misconceptions about AI?
Viney: Related to my last point, perhaps one big misconception is that AI can operate as a standalone approach for drug discovery, design and optimization! Creating a new drug is a complex effort that requires many steps and inputs. In my view, AI can serve as a powerful technology so long as it is integrated with human insights that are essential to the process of creating new medicines.
Bigal: I agree with Jo. The biggest misconception about AI is that it can be a panacea applicable to all aspects of drug discovery – solving all challenges by itself. Like any tool or platform, it is well suited to some areas and not others. One fundamental challenge of AI is the need for sufficiently large data sets to train the algorithms. It is necessary that these training sets have far more data points than the query set. If this is not the case, then AI will have little to no impact on the problem. This is particularly acute in drug discovery, where the query set (chemical space) is over 1063, and the data points are many orders of magnitude less than that.
Another misconception is that AI and machine learning are the same thing. Machine learning is a very specific sub-field of AI. In fact, almost everyone doing work in this field is using machine learning (image processing, language processing, text processing, clustering, property prediction, and so on).
AI is not a black box that magically solves any problem it is applied to. It is a diverse set of powerful technologies that requires ingenuity, deep understanding of the problem, and large amounts of quality training data to develop a successful application.
Biancalani: AI cannot replace human scientists in target and drug discovery – but it can definitely help them. Today’s datasets are so massive that humans cannot possibly “look” at all this data; we need computational methods to extract relevant patterns and information in an automatic fashion. AI offers the tools for doing just that.
Another common misconception comes from the name “artificial intelligence,” which suggests that these algorithms possess (or can eventually exhibit) intelligentia in the same way a human does. This is not correct. These algorithms can perform tasks that were typically performed by humans, but that doesn’t make the software “intelligent.” For example, a software that allows a vehicle to self-drive is very cool, but doesn’t imply that the software “learns” or “thinks” in the same way a human does.
What is the hype versus the reality of AI?
Biancalani: AI is an essential tool or “lever” for R&D and the creation of patient-centric solutions, but it is just one part of the puzzle. There are other critical levers for R&D, including a deeper characterization of human biology, the ability to conduct experiments at exceptionally high resolution and massive scale, and the exploration and application of diverse therapeutic modalities. It’s the interplay among these levers that has the potential to deliver a variety of benefits for patients.
AI is a great tool for navigating large datasets and finding insights that can inform the development of better medicines for patients. But we aren’t at the stage where AI can create a medicine on its own. We still need scientists to validate these insights in the lab and to test the safety and efficacy of potential medicines in clinical trials.
Viney: The excitement surrounding AI in drug development is palpable, as it has emerged as a powerful new technology that can help us to design better drugs and advance them faster to patients. The reality is that there are a multitude of ways that AI can be integrated in the drug discovery process, and our industry is in the early stages of building new approaches to use AI and machine learning. There is so much potential in the future; AI can make major contributions to how we can improve and accelerate the process of creating new medicines.
Bigal: Many companies and scientists have set out to apply AI and machine learning to the entire drug discovery spectrum, from target identification, small molecule discovery, and optimization, to biomarker discovery and clinical trial design and execution. There has been a huge amount of hype from some of these companies, stating that they will solve all the challenges of drug discovery. However, in reality, many of the areas they claim they can impact do not have large enough training sets for this to be possible. In particular, the application of AI to identify new chemical starting points and optimize affinity of chemical matter using purely image-based approaches is an area where the hype outweighs the reality.
As I mentioned, the theoretical query set of small molecules is thought to be about 1063, yet the training set in the best of circumstances is likely less than a million compounds (106), if we combine all known small molecules with enough information to train on. Thus, applying AI and machine learning to this challenge is not feasible. One simply has to look at the molecules produced by some of these companies purported to use image-based machine learning approaches to realize that this is largely hype. In reality, they are simply making small modifications to pre-existing chemical matter, which a good medicinal chemist could do easily without any computational help.
The challenge of small molecule drug design is a function of physics. The laws of physics are universal and thus applying machine learning approaches to enhance and optimize physics-based parameters requires a far smaller data set to train on. I believe companies pursuing these types of approaches and platforms are the ones that should be able to realize the value of machine learning to this problem.
What advice would you give to others interested in using AI for pharmaceutical R&D?
Bigal: My advice would be to make sure that you understand the problem from the first principles and not just assume that AI can solve it. Find those areas to which AI approaches are best suited, where sufficient training sets exist, and focus on those areas. Drug discovery is an incredibly complex and difficult undertaking; don’t assume there is one way to solve the challenges and instead think beyond AI to look for solutions. Importantly, never underestimate the need for experienced drug discovery scientists in your organization. Nothing can replace years of experience and a track record of success in developing drugs that address diseases of high unmet need. AI is only a tool to support those scientists and cannot replace the years of experience and know-how that will remain a critical component for success.
Biancalani: Smaller enterprises, such as startup companies, often have sophisticated AI methods. However, to be successful in leveraging these methods to solve complex problems and accelerate R&D, you need both sophisticated AI and large datasets. Large datasets can be very difficult for a small company to access, but they may be able to access them through collaborations or partnerships with larger companies like Roche and Genentech.
What will pharma’s future relationship with AI look like?
Viney: We have seen a lot of interest throughout the drug industry in AI and machine learning, and pharma companies are exploring different ways that AI can be used for specific drug modalities and in specific therapeutic areas. Like Tommasso says, it is important that companies maintain open dialogue with others who might be potential collaborators, while keenly focusing on advancing their own drug programs and developing drug product candidates to help patients.
The first AI-designed drugs are just beginning to enter the clinic, meaning we really are at the cusp of being able to see the power of the AI approach for accelerating and augmenting drug discovery and development. A decade from now, I hope we will be able to look back and see that this was an exciting time and a major turning point for medicine.
Biancalani: I believe AI can remodel every field where large datasets are present. This is an incredibly exciting time in the healthcare field. We are at an inflection point in drug R&D; science and technology are converging, and computational methods, such as machine learning, will be as essential as biology and chemistry to the future of medicine.
Thanks to this convergence, and the transformative scientific and technological advances in recent years, we have an opportunity to bring multiplicative, rather than incremental, benefits to drug discovery and development – and, most importantly, to patients.
Bigal: I think AI will continue to be a growing part of the pharma industry as we move beyond the hype and identify areas where AI can truly make a difference. With the continuing advancement of algorithms and hardware, we will continue to see innovative scientists think of new ways to apply this toolset.
It is important to remember that the concept of applying AI and machine learning to drug discovery is not new. Many of the concepts have been considered before only to fall by the wayside due to ineffective application, lack of computational power, weakness of algorithms, or the absence of enough data to train on. Though advancements in AI will make application to drug discovery more effective, I do not believe it will completely remodel the industry. At the end of the day, we are developing chemicals that have biological effects in complex organisms. The complexity of the human organism is still far beyond our understanding, and it would be naïve and arrogant to believe we have the knowledge to effectively model this in a computer to the point that it can replace experimental testing and hypothesis-driven science.
AI is an exciting new technology and we are in the hype cycle. Though some of the claims out there are for marketing purposes, there are many areas where the application of AI technology can genuinely increase throughput and efficiency of drug discovery, from target analysis with platforms like AlphaFold 2, to virtual screening of chemical libraries, to processing experimental results with image recognition. As we collect more data on biological interactions in organisms, we can envision AI helping to uncover complex patterns underpinning those interactions that would help us to understand disease mechanisms, select the most effective targets, rapidly identify and develop therapeutic molecules, and quantify effects of genetic variability to personalize treatments.
After finishing my degree, I envisioned a career in science communications. However, life took an unexpected turn and I ended up teaching abroad. Though the experience was amazing and I learned a great deal from it, I jumped at the opportunity to work for Texere. I'm excited to see where this new journey takes me!