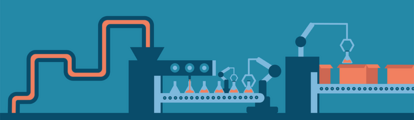
Making the Most of Big Data
Data is of massive value to all pharma processes and operations, but it is also of crucial importance to patient acquisition. But given the vast volume of data companies must trawl through, what is the best approach for finding the information that matters?
Devesh Verma, Brian Gibbs | | 4 min read | Opinion

Pharma companies are in the business of bringing life-saving medications to the right patients at the right time. But if there are no patients, there is no pharmaceutical industry! Therefore, from a broad perspective, the patient challenge for the industry can be classified into two categories. The first challenge is to “acquire” the right patient on the brand, and the next challenge is to retain the patient so they can get optimal benefit from a given therapy.
Pharma medicines are produced with more specialized and novel agents than ever before, which, in turn, results in higher costs and poses reimbursement challenges from the payer community. However, payers are willing to pay for the medications that work effectively for patients, so the industry has to identify the right patient population for a therapy, and the point at which they become eligible.
What does this have to do with AI? Data is the foundation of building an ideal patient acquisition and retention strategy for any pharma company. As the world gathers greater amounts of data from connected devices (for example, health monitors, EHR, lab testing, and so on), an eligible patient for the right therapy can be identified much earlier in their journey. Data can also identify the profiles of patients who may be susceptible to poor adherence to therapy.
However, the industry’s biggest struggle is connecting data across multiple sources and making it available to business stakeholders in real-time. Although connecting data sounds straightforward, when bringing together multiple systems, companies require a primary identifier, which is not readily available at the patient level due to privacy concerns. So, the question becomes, what can the industry do to learn and act on data in a compliant manner?
Machine learning may offer a solution. It is an application of AI that provides systems the ability to automatically learn and improve from experience with little or no explicit programming. Best-in-class organizations will have robust libraries of dynamic AI and machine learning algorithms that inform or directly drive action and provide endless opportunities to address complex questions more precisely and dynamically. Issues related to healthcare physician communication, promotional engagement, and patient behavior can all be predicted and acted upon using such systems.
But as with any algorithm, their ability to adaptively learn using streaming data and accelerated turnaround times is a significant risk. This particular challenge is referred to as “learning bias” and involves systems being trained on biased data. Though easily detectable by examining algorithm performance across learning iterations and closely profiling predictive errors, these steps need to be explicitly built into the AI/machine learning system (and usually require human intervention to diagnose and correct).
Therefore, algorithms should be developed with attention to bias in ways that may drive suboptimal or unethical actions. In many cases, algorithms may need to be simplified to provide explanation and understanding to inform stakeholders of the interventions that can help them avoid this type of predicted outcome.
Though this issue may seem daunting, the potential for AI and machine learning growth within the pharmaceutical and healthcare sector is substantial. So much so that ample funds are being made available for expanding and investing in these technologies. The general perception is that there will be a strong return on investment upon their implementation.
Although a vast number of pharma business problems are amenable to machine learning-based solutions, there are also a significant number that are not and will continue to require knowledge, expertise, and judgment.
In our view, there are three necessary conditions for an AI or machine learning solution to generate high value for pharma organizations:
- Domain expertise. Teams developing the algorithms must understand the nuances and complexities of the buying process in pharma in general, the differences across ecosystems, and specify their algorithms to capture these complexities.
- Data expertise. Teams developing the algorithms must understand the vast data challenges in pharma, differences across regions or geographies, and have solid initial starting protocols for dealing with those challenges.
- AI and machine learning expertise. Teams developing the algorithms must understand best practices for algorithm development, be able to scale the analytic process for numerous countries and dynamically evaluate results for precision and bias, and evaluate the tradeoffs between algorithm explanatory power and parsimony before having them operationalized to inform – or directly drive – action.
If companies can keep these factors in mind as they embark on their journeys, they should be able to reap the massive benefits that these digital solutions offer. Without them, they may find themselves inundated and overwhelmed with the highly valuable, yet unrefined data they own.
Devesh Verma, Decision Science Principal at Axtria Inc.
Devesh Verma, Ph.D., is a Principal at Axtria, where he leads the marketing solutions practice. He has 20+ years of experience in brand marketing and planning, sales leadership, and management consulting. Before joining Axtria, Devesh led US brand marketing for a global pharmaceutical company’s key franchises. Devesh led a customer-centric transformation of the franchises by integrating traditional sales force channels with evolving digital channels. The customer-centric approach helped the franchises achieve the highest annual sales on record of over $3.3B.
Devesh’s experience also includes working at MarketRx, where he led many large engagements in marketing mix optimization, sales force size and structure, and call planning. Earlier, he has also worked at a major US airline and a leading technology firm.
Devesh received his Doctorate in Business Administration from the University of Minnesota. He received his Master’s in Aerospace Engineering from the University of Minnesota and Bachelor’s in Aerospace Engineering from the Indian Institute of Technology, Kharagpur.
Brian Gibbs, Ph.D. Principal – Decision Science, Axtria, Inc.
Brian is a Principal in Axtria’s Decision Sciences Practice and has overseen analytics-based consulting projects for life sciences clients involving targeting, segmentation, marketing mix and optimization, and AI and adaptive machine learning for a wide range of commercial applications.
Prior to joining Axtria, Brian was Vice President in the Consulting Solutions practice at Symphony Health Solutions, a Senior Principal in the Promotion Response practice at IMS Consulting, a Principal in the Management Consulting Practice at Price Waterhouse Coopers, Vice President and Director of Advanced Analytics at GfK V2, and an Associate Partner at Rosetta Marketing.
In addition, he is a frequent presenter at top pharmaceutical industry conferences (e.g., PMSA, PMRG). His academic experience includes seven years in applied social science research and teaching at the University of Michigan and Princeton University and periodic lecturing at the Wharton School.
Brian holds a B.A. in Math/French from Rutgers College (New Brunswick), D.E.A in Political Economy from Institut d’Etudes Politiques (Paris), and a PhD. and M.S. in International Political Economics from the University of Michigan (Ann Arbor).