Neural Networks Vs Bruteforce Docking
How Finnish researchers carried out one of the world's largest virtual drug screens using AI
Ina Pöhner | | 4 min read | Opinion
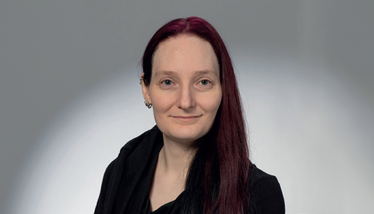
Credit: Author supplied
Many researchers rely on rapid, computer-aided screenings of large compound libraries to identify agents that can block a drug target. In recent years, the size of these collections has surged considerably – and we’re now at a crossroads. Libraries are growing faster than the processing capabilities of computers. Screening a billion-scale compound library against a solitary drug target is a time-consuming endeavor, even when using state-of-the-art computers. Faster approaches are desperately needed.
While myself and the team were getting up-to-speed with the field, we noticed a gap. Previous research in the field had mostly been performed on million-scale datasets, despite being intended for billion-scale applications.
We believe that our research represents the first rigorously benchmarked machine learning (ML)-boosted and AI-driven virtual screening approach (1). We conducted brute-force docking of 1.56 billion compounds to two targets from ongoing drug discovery efforts. Research on a similar scale remains scarce in most contexts, so when we started the project, we identified just a single publicly accessible giga-scale docking dataset.
Before delving further, it is important to understand the fundamental aspects of docking. Molecular docking is a computational process of predicting how a small molecule ligand binds to its target receptor. This involves two main steps: fitting the small molecule into the target’s binding region and then calculating a “docking score” to quantify the complementarity between the ligand and the receptor. These scores are then used to model a compound’s binding affinity (albeit imperfectly).
Traditionally, docking was used to narrow down potential hit candidates from extensive screening databases, offering a higher throughput than experimental methods. But, once our available libraries of compounds grew beyond the billion scale, even the high throughput of docking and related screening methods became insufficient in a reasonable project timeframe. In fact, many of the challenges that researchers face in in silico screening have existed for a long time. For example, the scoring functions used in docking are well-recognized as imperfect predictors of binding affinity, meaning that the reliable identification of true actives based on docking alone is impossible.
Even the fastest methods for brute-force molecular docking can only process tens of molecules per minute (per CPU). In a regular, early-stage drug discovery project timeframe of no more than a few days, it was possible to dock entire compound libraries on the million scale to support the selection of best hit candidates. But because conventional docking processes every compound one by one, this is not feasible with giga-scale libraries.
As evidenced in our study, performing brute-force docking on billions of compounds for a single target can now extend to several months – even with the assistance of supercomputing resources. In addition, the risk of drowning out true actives in a lake of false positives – a general problem of docking studies – has been observed to worsen with growing library sizes.
The need to modernize conventional screening methods is clear. And I am happy to say that we may have a solution. Meet HASTEN (our shorthand for machine learning boosted docking) – a technology that leverages deep neural networks, in combination with conventional docking, to accelerate docking-based virtual screening and enable the timely processing of ultra-large compound libraries. Neural networks – when presented with enough examples – can learn the features of high-scoring molecules. Small subsets of the huge compound libraries are docked by conventional brute-force docking, and the obtained docking scores are used to train the neural network. HASTEN, thereafter, acts as a surrogate for docking, predicting docking scores for the remainder of the library much faster than brute-force docking could. The time required to screen 1.56 billion compounds in our study was reduced from four months to about ten days.
HASTEN enabled us to complete the giga-scale screen in under two weeks, since we only had to dock one percent of the whole compound library. We also observed a robust recall of more than 90 percent of the very top-scoring virtual hits from the brute-force docking. In a fraction of the time, HASTEN was able to produce equivalent, and, in some cases, better results.
Building on the success of the current study, we hope to push the boundaries of library and dataset sizes even further. Given the known target dependence of docking tools and their scoring functions, we’re looking into different method combinations to enable the best possible choice for different drug targets. We believe this approach will also help us address some of the shortcomings of the current tested methods, such as in modeling target flexibility.
- T Sivula, “Machine Learning-Boosted Docking Enables the Efficient Structure-Based Virtual Screening of Giga-Scale Enumerated Chemical Libraries,” Journal of Chemical Information and Modeling, 63, 18 (2023): DOI: 10.1021/acs.jcim.3c01239
Researcher at the School of Pharmacy, University of Eastern Finland