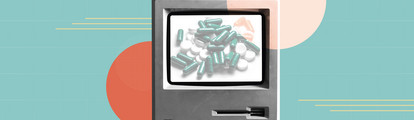
Taking a Byte out of Formulation Development
With so much technology at our disposal, it’s time to get smart in formulation by adopting in silico approaches.
Ronak Savla, Julien Meissonnier, Jan Neelissen | | Longer Read
There are a number of technological advances that have helped drive improvements in small-molecule drug design – the sequencing of the human genome, increases in computing power, and high-throughput screening, to name just a few. Receptors and proteins that were once seen as undruggable at the cellular and sub-cellular levels have suddenly become more targetable. But drugs that are ligands for these newer targets are larger and more lipophilic (poorly soluble) than those in the past, introducing more biopharmaceutical and pharmacokinetic (PK) hurdles to optimal oral absorption and bioavailability.
Besides redesigning the chemical structure of the drug molecule, formulation development can help address these challenges. The primary goal of formulation development is to improve drug efficacy and safety by improving solubility and modifying the PK of the drug molecule, particularly absorption.
However, drug formulation is largely an empirical method that requires considerable time, material, and labor. Increasingly, companies want a fast “go/no-go” decision before spending more time and resources on a project. And ideally you want to get the decision right first time. Harnessing the power of in silico modeling and simulation technologies, along with more physiologically relevant, high-quality in vitro studies, should lead to faster formulation development, less attrition, reduced costs, and – most importantly – better treatments for patients. Modeling approaches can identify and predict oral absorption risks or liability factors, so that formulators can address the most pressing issues.
During the 2018 Controlled Release Society Annual Meeting in New York, we organized a panel discussion titled, “Inflection Point of Drug Formulation: Advanced In Silico Applications for Rational Drug Development.” The focus of the session was how in silico technologies – solubility prediction and physiologically based pharmacokinetic (PBPK) modeling, and molecular dynamics simulations – influence formulation studies. In an ideal world, most (if not all) formulation development studies would be performed using computational tools with minimal reliance on in vitro and in vivo experiments. Unfortunately, despite advances in technology and computing power, most in silico approaches have yet to achieve the level of robustness to minimize or eliminate the empirical nature of formulation. Of these technologies, solubility prediction is the most widespread, PBPK modeling the most advanced, and molecular dynamics the newest – representing the next generation of computational drug delivery tools.
Here, we focus on solubility prediction and PBPK modeling tools by providing a summary of the current state and insights on our experiences working with them. We look at these tools, in particular, because of their ubiquitous nature in drug development and importance in making drug formulation decisions.
A Common Problem
Solubility is one of the primary factors of oral absorption and exposure, according to the Biopharmaceutics Classification System (1), Developability Classification System (DCS) (2) and Biopharmaceutical Drug Disposition Classification System (BDDCS) (3). Solubility is perhaps the most commonly predicted molecule characteristic. Many pharmaceutical companies use solubility cutoffs, based on aqueous solubility, when selecting which compounds to advance for further development. But there are multiple formulation technologies including amorphous solid dispersions and lipid-based drug delivery systems that can improve solubility. We have three recommendations for integrating solubility predictions into drug formulation:
- Solubility predictions should be taken with a grain of salt. Predictions provide guidance, but should be carefully understood before making formulation decisions. Compared with the original “General Solubility Equation,” (4) whose inputs were melting and LogP, newer solubility prediction models, such as genetic algorithms and artificial neural networks, strive to establish quantitative structure property relationships (for example, molecular weight, polar surface area, aromaticity, rotating bonds), but are not significantly better at predicting solubility (5, 6, 7). It is quite common for different software to make different solubility predictions. One major reason for this variability lies in the complex nature of solubility. A drug’s solubility is dependent on its molecular descriptors, composition of the solvent system, and molecular form parameters. These variables present a challenge to create uniform model training datasets for prediction.
- Multiple solubility values should be predicted and/or measured in a variety of media and biorelevant buffers to more accurately and completely represent in vivo conditions. As an example, food effect is a major concern for poorly soluble drugs, such as protein kinase inhibitors. Comparing drug solubility in both fasting state and fed state simulated intestinal fluids can offer some insights as to whether the drug will experience significant food effect.
- Solubility should be analyzed in relationship to the dose, as performed in the DCS. The DCS offers an equation to calculate the solubility-limited absorbable dose (SLAD) – the dose above which solubility is the limiting factor for absorption. Low solubility is not as big of a factor for drugs whose SLAD is greater than the dose compared with those drugs whose SLAD is less than the dose. For drugs whose SLAD is greater than the dose, solubility enhancing formulation technologies to improve absorption may make minimal improvement, whereas these technologies may be critical for drugs whose SLAD is less than the dose.
Pharmacokinetics at work
The gold standard of PK studies are in vivo animal and human studies, but these studies require considerable time and effort. Better understanding of physiology and introduction of in silico PBPK models, however, has drastically reduced PK-related attrition (8). PBPK modeling tools are based on mechanistic models that divide the gastrointestinal tract into compartments described by differential equations. The integration of these differential equations with drug characteristics (for example, pKa, LogP, particle size), formulation factors (for example, solubility and dissolution profile), available PK data, and gastrointestinal physiology (for example, gastric emptying, transit time) give the drug developer some level of predictive capability. And they can help companies choose the molecule with the best properties most likely to attain sufficient exposure, or choose a molecule whose PK challenges are addressable by formulation technologies. Some PBPK modeling tools also provide parameter sensitivity analysis (PSA), which can identify how certain formulation and drug properties affect PK properties.
The use of fundamental physicochemical properties of drug molecules and physiological values (for example, transit time, gut pH and volume) is called a bottom up approach. The fidelity of this approach relies on a selected set of measured or predicted properties of drug molecules and physiological values of the gastrointestinal tract. A bottom up approach is often done early in drug development using off-the-shelf inputs, and prior to the completion of any in vivo studies. The goal is to gain insights into any challenges that the drug is likely to face, such as poor exposure and rapid or extensive clearance. As an example, lack of efficacy may be the result of poor target site exposure because the molecule has low bioavailability, the molecule does not distribute to the target site, and/or is rapidly cleared. In addition to PBPK modeling, the BDDCS uses solubility and permeability to model drug disposition, route of elimination, and effects of efflux and active transporters. According to the BDDCS, the majority of poorly soluble drugs are subject to hepatic clearance, so scientists should pay extra attention to understanding if solubility (the driving force for fraction absorbed) is the only hurdle to adequate exposure, or if clearance (gut wall metabolism and hepatic metabolism) are also potential issues. We’ve seen many instances (31 percent of poorly soluble molecule candidates, according to Catalent’s latest analysis) wherein drug developers spend time and resources fixing one aspect, such as poor solubility, when the real issue turns out to be extensive first-pass metabolism or rapid clearance, for which oral formulation technologies are of minimal help. Therefore, PBPK models can help guide formulation development to address the correct issues.
Once some in vivo studies have been completed, PBPK models can also be built “top down” using PK values from animal studies to build more accurate human predictions. The data from in vivo studies in conjunction with PBPK models can also help with deciding on the optimal formulation pathway. PBPK models help set the target for formulation development studies and before beginning any formulation activity. We recommend that formulators understand whether complete or partial resolution of the solubility issue will result in achieving target PK parameters, as well as whether improvements in peak plasma concentration help if efficacy is driven by exposure. Such insights will enable formulation scientists to make the right trade-off between improvement in PK parameters, target dose, and drug load in formulation.
Finally, PBPK models help construct the oft-missing bridge between in vivo PK studies and in vitro dissolution models, and allow development of in vitro-in vivo correlation (IVIVC). IVIVC can then be used for quality assurance for different batches, or when formulation modifications are made to ensure the new drug formulation will have the same in vivo behavior.
Given some of the limitations of current in silico approaches, the best PBPK models should incorporate in silico, in vitro and in vivo data inputs, such as drug molecule physicochemical characteristics (molecular weight, logP, pKa, acid/base), solubility across the pH range, solubility in simulated fluids, and various PK characteristics, including permeability, plasma protein binding, and intrinsic clearance from in vitro microsomal and/or hepatocyte studies. A good model will allow for the determination of many PK parameters with a high degree of precision. Be aware, however, that the quality of the data inputs play a significant role in determining the precision of the predictions. Typically, in vitro and in vivo data are preferred over inputs from in silico predications, but it is not always feasible in early product development to conduct in vivo studies.
Time and effort!
Drug formulation is an important aspect of pharmaceutical development. And though this statement may seem obvious, many companies forego critical formulation activities and advance drugs with unresolved solubility and/or PK challenges resulting in drugs reaching the market with suboptimal and sometimes detrimental impact on the patients (9). In other cases, innovators focus their efforts and resources on a non-developable molecule because of inaccurate modeling and/or incomplete understanding of the real hurdles to exposure and activity. In either case, the time and cost of pharmaceutical development are driven higher with little-to-no patient benefit.
There are several formulation technologies that can help improve solubility and absorption – and it’s crucial that manufacturers understand these given the increasing number of poorly soluble drugs in the pipeline. Solubility prediction and PBPK modeling are useful tools to understand the potential challenges that each molecule may present, and can accelerate and guide candidate selection and formulation development. However, formulators must understand the caveats and nuances of these tools and not blindly follow outputs.
Good formulation development demands considerable time and effort – but the rewards justify the means.
- GL Amidon et al., ”A theoretical basis for a biopharmaceutic drug classification: the correlation of in vitro drug product dissolution and in vivo bioavailability”, Pharm Res. 314-420 (1995). PMID: 7617530.
- JM Butler, JB Dressman, “The developability classification system: application of biopharmaceutics concepts to formulation development”, J Pharm Sci, 99, 4940-4954, 2010. PMID: 20821390.
- CY Wu, LZ Benet, “Predicting drug disposition via application of BCS: transport/absorption/elimination interplay and development of a biopharmaceutics drug disposition classification system”, Pharm Res, 22, 11–23, 2005. PMID: 15771225.
- Y Ran, SH Yalkowsky, “Prediction of Drug Solubility by the General Solubility Equation (GSE)”, J Chem Inf Comput Sci, 41, 354-357 (2001). PMID: 11277722.
- F Chevillard et al., "In Silico Prediction of Aqueous Solubility: A Multimodel Protocol Based on Chemical Similarity", Mol Pharm, 9, 3127-3135 (2012). PMID: 23072744.
- T Sanghvi et al., "Playing Russian roulette with tyrosine kinase inhibitors", QSAR Comb Sci, 22, 258-262 (2003). PMID: 23340477.
- J Huuskonen, “Estimation of Aqueous Solubility for a Diverse Set of Organic Compounds Based on Molecular Topology”, Chem Inf Comput Sci, 40, 773-777 (2000). PMID: 10850781.
- S Boyer, C Brearley, AM Davis, “Attrition in Drug Discovery and Development”, Attrition in the Pharmaceutical Industry: Reasons, Implications, and Pathways Forward, 1st edition, John Wiley & Sons (2016).
- RZ Szmulexitz and MJ Ratin, "Playing Russian roulette with tyrosine kinase inhibitors", Clin Pharmacol Ther, 93, 242-244 (2013). PMID: 23340477.
“How many people can truly say that they are working on what they dreamed of as kids? I guess I’m one of the lucky ones.” After learning about the field of nanotechnology as a high school student, Ronak saw the opportunity of combining his interest in science with his passion to help others. Ronak started working in a nanotechnology drug delivery lab during the summer after his freshman year at Rutgers University. He continued developing innovative nanoparticle drug delivery solutions during pharmacy school and graduate school. This has launched him to his current position as the Applied Drug Delivery Fellows at Catalent Pharma Solutions as part of the Rutgers Pharmaceutical Industry Fellowship Program.