Optimizing mRNA Sequence Design
Just as codon optimization evolved in the early 2000s, RNA sequence design is emerging as a path to transform the economics and methodologies of RNA as a drug modality
Claes Gustafsson | | 4 min read | Opinion
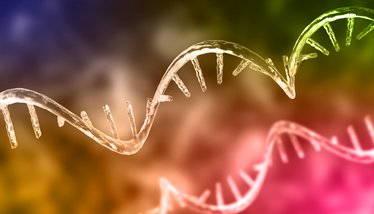
Credit: AdobeStock.com
The COVID-19 pandemic swiftly propelled us into the era of mRNA-based therapeutics. In less than a year, the scientific community went from not knowing the identity of the viral pathogen to manufacturing millions of vaccine doses with an exceptional 95 percent efficacy. This remarkable achievement under extraordinarily challenging circumstances highlights the transformative potential of mRNA as a drug modality.
Messenger RNA therapeutics have numerous advantages over protein therapeutics:
- mRNA can encode any protein (or therapeutic RNA).
- mRNA circumvents typical protein manufacturing challenges such as folding, multi-subunit assembly, aggregation, and post-translational modifications of the functional protein since the drug manufacturing is performed directly by the transfected cell.
- The design-to-manufacturing timeline is very rapid for mRNA therapies, making quick updates to existing therapeutics feasible, for example, vaccines where a novel viral mutant necessitates rapid updates of the vaccine antigen.
- The scalability of RNA manufacturing is also relatively straightforward compared to proteins, as evidenced by the distribution of over 12 billion COVID-19 vaccinations. We often talk about scale-up in drug manufacturing, but I’m particularly excited about the scale-down of drug delivery with mRNA where, for example, gene replacement drugs can be applied to rare disease populations in a transient manner, mitigating the risk of unforeseen side effects. Cost of small-scale RNA synthesis with equal quality and regulatory rigidity to large commercial scale is eminently doable.
Beyond the continuous development of mRNA vaccines for infectious diseases – such as new COVID-19 variants, influenza virus, respiratory syncytial virus, and Zika virus – there are ongoing efforts to explore RNA for personalized cancer vaccines, mRNA-enhanced cell therapy, therapeutic genome editing (by encoding Cas9 and gRNA), transient gene replacement, therapeutic antibodies, and more.
mRNA drugs offer a vast design space because of the redundancy of the genetic code. With, on average, three codons coding for each amino acid, a tiny 20-amino acid peptide can be encoded by over three billion different mRNA sequences (320). This figure does not even account for the additional diversity derived from modified nucleotides, alternative capping, and lipid nanoparticle formulations. Different mRNA sequences can profoundly affect manufacturability, in vivo stability, translational efficiency, RNA secondary and tertiary structure, tissue-specific expression, and more while still encoding the exact same protein. Designing the 'optimal' mRNA sequence remains challenging and likely to yield multiple independent answers because of the almost infinite sequence space and the many different functional goals.
The Medicine Maker Presents:
Enjoying yourself? There's plenty more where that came from! Our weekly Newsletter brings you the most popular stories as they unfold, chosen by our fantastic Editorial team!
Controlling mRNA stability and expression levels through sequence design is crucial, but it’s also possible to create sequences that are easier to purify and faster to manufacture, reducing costs and improving production efficiency. Optimal mRNA design can mean many different things to different groups, depending on their specific needs and goals. Some current mRNA design strategies rely on identifying and cataloging sequence motifs to avoid or include based on perceived functionality. While these approaches are quick to develop, they often lack the extensive experimental data needed to ensure biological relevance and tend not to address the epistatic nature of biology.
The current mRNA design challenge mirrors the codon optimization problem faced by the industry in the early 2000s. Initially, using the most common codons for a given amino acid was believed to maximize protein expression. The prevailing assumption was that the most abundant codon for an amino acid in the host genome would correspond to the most abundant tRNA isoacceptor, leading to higher protein expression. This assumption proved overly simplistic, neglecting the dynamic regulation of tRNAs, tRNA maturating enzymes, and other translational components. Despite these complexities, synthetic gene sequence design platforms proliferated, varying in effectiveness. Today, almost all commercial recombinant protein production relies on optimized, non-natural nucleotide sequences, including proteins expressed in their endogenous hosts.
Successful gene codon optimization algorithms use pattern matching based on empirical data, creating and testing multiple gene sequences to identify patterns enhancing protein expression. For example, a set of, e.g., 96 mRNA sequences, each encoding the exact same amino acid sequence, and each of the nucleotide sequences designed to be approximately 70 percent identical to one another (2 out of 3 nucleotides in each codon), can be defined using Design of Experiment (DoE) techniques. This design principle systematically distributes all possible sequence variables (e.g., codon bias, homopolymers, RNase sites, CpG-islands, secondary and tertiary structures, and GC content) among the gene sequences. These sequences are then synthesized and tested for protein expression in an expression system, identifying both poorly and highly expressed variants. Machine learning algorithms can then derive cross-validated sequence patterns that correlate with and causally relate to protein expression yield. The resulting algorithm is subsequently used to design new gene sequences tailored to maximize recombinant protein expression in the tested host context.
This data-driven approach can similarly be applied to mRNA design, leveraging machine learning to optimize sequences. Researchers can map out the mRNA design space by applying the same logic and processes used in codon optimization for recombinant protein expression. Experiments involving multiple open reading frame sequences and standardized 5’ and 3’ untranslated regions in various cell lines will provide valuable insights into host cell effects and interactions between genetic elements. These ongoing experiments offer a wealth of sequence-function information that can be mined using ML strategies similar to the ones successfully employed for codon optimization, driving future innovations in mRNA sequence design.
In conclusion, exploring RNA sequence features to improve therapeutic mRNA design is just beginning. By leveraging lessons learned from codon optimization, RNA sequences will be able to drive future innovation, complementing and potentially overtaking protein therapeutics for certain applications.
Co-Founder & Chief Commercial Officer at ATUM