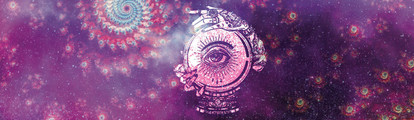
Predicting All Outcomes
A machine learning algorithm aims to take the “what ifs” out of chemical synthesis
Synthetic chemists who want to conduct novel reactions typically turn to the results of previous experiments to help ascertain the optimal conditions for enantioselectivity – and the likelihood of success. But it’s a time-consuming and error-prone process. Matthew Sigman, a chemist at the University of Utah, estimates that there can be anywhere between seven and ten variables in a typical pharmaceutical reaction. “With billions of possibilities, you cannot cover all the variable space with any type of high throughput operation,” he says.
Tapping into the power of AI and machine learning, Sigman and Jolene Reid – another chemist at Utah – have been able to predict the outcome of chemical reactions by analyzing previously published chemical reaction data (1).
“We hope our research will allow chemists to make informed decisions about reaction conditions before beginning experimentation, effectively streamlining the drug discovery process,” says Reid. “Previously, such predictive models were generated for single reactions; however, our research shows that selectivity models can be built and generalized over a range of reactions without affecting the accuracy in prediction.”
Enantioselectivity is sensitive to any reaction component, including temperature and the type of solvent used, and so the team used multivariate linear regression, a machine learning algorithm, to dig into the impact of all reaction parameters by analyzing 367 data entries collected from 17 literature reports on the enantioselectivity of imines. Reid explains that imines are synthetically important molecules with lots of data available on their reactions types – a prerequisite for the team’s analysis. The resulting model was able to predict the outcome of 15 reactions involving one reactant that wasn’t in the original set, and the team went on to predict 13 more reactions, whose reactants and catalyst were not included in the original data.
Now, the team’s goal is to create more general models that would enable the prediction of experiments with reaction conditions that look very different to those in the original set. They also plan to use their tools to better understand the limitations of predictive models, so that chemists will know the reliability of predictions that are used to guide optimal experimental conditions.
- JP Reid and MS Sigman, “Holistic prediction of enantioselectivity in asymmetric catalysis”. Available at https://go.nature.com/2mPWjTf. Last accessed: September 30, 2019.
After finishing my degree, I envisioned a career in science communications. However, life took an unexpected turn and I ended up teaching abroad. Though the experience was amazing and I learned a great deal from it, I jumped at the opportunity to work for Texere. I'm excited to see where this new journey takes me!