Putting the “Go” in Oligonucleotide Manufacturing
Intellegens CEO Ben Pellegrini explains how machine learning is set to change the oligonucleotide manufacturing landscape
Ben Pellegrini | | 3 min read | Discussion
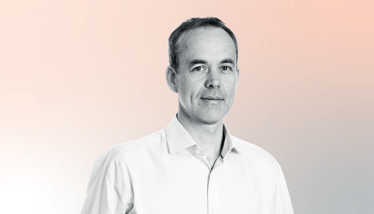
With the aim to overcome key barriers to applying machine learning (ML) to real experiments and processes – for example, the fact that ML typically struggles with ‘sparse’ data (data with gaps) – our latest project, in partnership with the Centre for Process Innovation (CPI) and with funding from Innovate UK, focuses on the potential for ML to act as a catalyst for manufacturing oligonucleotide therapeutics. We are improving predictive modelling tools, experimental program design, optimal process parameter discovery, and target output identification.
Oligonucleotides are difficult to manufacture – particularly at scale. They are large, complex molecules that require a multi-stage synthetic process, interleaved with significant purification and analysis stages. The presence of impurities or small variations in reaction conditions and process steps can make significant differences to the structure, yield, and quality of the end product. Synthesis is expensive, meaning that experimental data is often sparse, and research teams would prefer to extract as much value as they can from the data that exists. Alongside these common industry problems, oligonucleotide manufacturing also has significant sustainability challenges; namely, large amounts of waste produced, poor atom economy, and low use of renewable feedstocks.
For these reasons, oligonucleotide manufacturing is an ideal target for ML, which can help detect subtle, non-linear relationships in multi-parameter data that might otherwise be missed. I expect ML to help research teams better understand the key factors driving oligonucleotide manufacturing processes, leading to improved design and control of these processes.
The importance of oligonucleotide therapies cannot be understated. Despite significant advances in medicine, there is still a large gap between the number of diseases and disorders that are druggable with approved therapies. Oligonucleotide therapies represent a relatively new and innovative approach with the potential to treat a wide range of diseases, including rare genetic disorders, certain types of cancer, and neurodegenerative conditions. The high specificity of oligonucleotides – and their ability to target gene mutations or protein expression – means that they are a form of personalized medicine, with fewer off-target effects and, potentially, fewer side effects than small molecules. Given the promise, many companies within the pharma industry are either investing heavily in platform R&D to progress oligonucleotide pipelines or forming partnerships and collaborations to advance these to commercialization.
Going back to ML adoption in this space, oligonucleotides will likely suffer the same challenges seen in other modalities and sectors. Traditional ML methods and algorithms require large, high-quality datasets for training. And as noted, In oligonucleotide manufacturing it is challenging to obtaining sufficient data – especially for highly complex and nonlinear proprietary processes. Over-simplified models may not provide meaningful insights. Building models that can generalize across different data formats and processes for different pharma companies will also be challenging. As will the integration of ML solutions into existing manufacturing systems, where it is important to work seamlessly with automation and control systems. The final barriers to adoption are simply inertia or a lack of knowledge and understanding of ML technologies.
Certainly, a small number of specialist companies have made progress in addressing the manufacturing challenges of oligonucleotides, but their insights and models are often proprietary (and pharma is an industry where knowledge is not widely shared). As with many challenges, collaboration is likely key; pre-competitive projects could combine expertise, with ML models acting as a vehicle for capturing and sharing knowledge among the collaborating organizations. This way, what is learnt can be shared to accelerate progress and drive innovation.
And in my view, it’s absolutely worth it! A somewhat consistent rule of thumb for ML technology when applied to the DoE is a reduction of around 50–80 percent in the number of experiments required to achieve a given objective. Furthermore, it could generate new insights and guide informed decision making. Yes, it’s speculative – but the effective use of ML could drive two- to five-fold reductions in the problematic process development phase of bringing new oligonucleotides to market.
CEO and co-founder of Intellegens, a machine learning (ML) software company, focused on research-intensive industries such as chemicals, life sciences, and materials. Originally a spinout from the University of Cambridge, Intellegens worked alongside automotive giant Rolls Royce in a research project to extract more value from rare experimental and process data to optimize the design of a new superalloy.