
AI Reunites Pharma with Mother Nature
Can AI facilitate a grand comeback for medicines sourced from Mother Nature?
Many popular therapeutics have come from nature, but with the low-hanging fruit taken and new discoveries in the field becoming increasingly challenging, big pharma began to look to other sources of new drugs, effectively severing the original branch of medical discovery for small molecules. But not all that is abandoned need be forgotten – as proven by work undertaken in Carnegie Mellon University’s Computational Biology Department, which could accelerate a “return to the future” of novel natural product research.
We sat down with two of the involved researchers – Bahar Behsaz and Hosein Mohimani – to hear how they used an AI platform to identify molecules that could open up new avenues in small molecule discovery.
Meet the Researchers: Bahar Behsaz and Hosein Mohimani both work at the Computational Biology Department in the School of Computer Science at Carnegie Mellon University, Pittsburgh, Pennsylvania, USA. Bahar is a Project Scientist and Hosein Mohimani is an Assistant Professor.
What are your research interests?
We focus on developing cutting-edge technologies for discovering new drug leads from nature. We focus on a class of molecules called natural products. These molecules were naturally selected across millions of years of evolution to carry a very wide range of bioactivities, which makes them a generous source of drug discovery. We’re specifically interested in developing computational methods that integrate a range of biological Big Data to identify drug-like natural product molecules. In the past, much of our research focused on identifying known molecules – but, today, we have pushed the technology further and are focusing on scalable methods that can discover completely new small molecule compounds with no known counterparts.
What was the motivation behind your research?
Approximately half of all existing clinically approved drugs are inspired by nature. This includes most antibiotics and many widely used antitumor medications. Take penicillin, for example; it is among the most used and well-known natural product drugs, and was discovered – accidentally – by Alexander Fleming when he left a Petri dish uncovered overnight. Most natural products were discovered either by luck or through complex, time- and labor-intensive trial-and-error experiments. It often takes years and millions of dollars to make a single discovery.
By the early 1990s, major pharmaceutical companies had mostly abandoned the search for new natural products. Today, access to high-throughput omics data provides a new roadmap for scalable natural product discovery, but this is only possible if we have computational methods that can use this massive data.
What were your key findings?
We presented a new platform (NRPminer) that combines the power of two different omics technologies – genomics and metabolomics – to discover new natural products at scale (1). The machine learning algorithms we have developed automatically match the signals of a microbe’s metabolites with its genomic data, then identify signals that correspond to a new natural product. This provides researchers with the crucial information they need to isolate the natural products and begin developing them for clinical trials. The method is scalable and can work automatically across thousands of microbial samples.
We further demonstrated that our approach can identify many novel natural products generated in different environments. In particular, we presented four completely novel families of molecules from soil-associated microbes and human skin microbiota. We have demonstrated the antiparasitic activities of two of these non-ribosomal peptide families using direct bioactivity screening, illustrating the strength of our method for discovering novel drug leads.
What’s next for this project?
We are currently applying our integrative methods to even more diverse classes of natural products with different functionalities, and we are creating more methods that are suitable for commercial-scale lead drug discovery.
In your view, does pharma make the most of machine learning for drug discovery?
AI is certainly a staple in today’s pharmaceutical research and development. In the past decade, we have observed more and more companies focusing on the use of AI and machine learning. The most commonly deployed methods focus on prediction of activity, drug design, repurposing, and testing drugs.
Parallel to that, the past decade saw significant advances in high-throughput omics technologies, which opened up a range of new opportunities. In accordance with these experimental advances, scalable computational platforms are emerging. These could enrich all discovery efforts tapping into novel natural products – a completely new space of unexplored small molecules with stunning structural and functional diversity. Scientific progress is now at the stage where – as we demonstrated – it is possible to apply large-scale methods of natural product discovery at the commercial level.
It is not surprising that we are now seeing big pharma shift to a clear focus on natural products for discovering novel drug leads. We are confident that this is just the beginning of the resurgence of natural products, enabled by scalable machine learning and data mining methods.
Through various examples, we have demonstrated the methods that can mine this untapped goldmine. Current AI technologies can provide pharmaceutical researchers with crucial information about new compounds and their potential as valuable drug leads. This would provide an opportunity to tackle currently untreatable diseases.
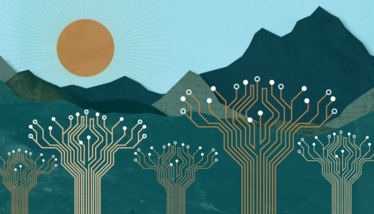
- B Behsaz, H Mohimani et al., “Integrating genomics and metabolomics for scalable non-ribosomal peptide discovery”, Nature (2021). DOI: 10.1038/s41467-021-23502-4
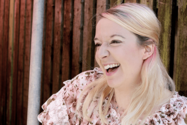
Making great scientific magazines isn’t just about delivering knowledge and high quality content; it’s also about packaging these in the right words to ensure that someone is truly inspired by a topic. My passion is ensuring that our authors’ expertise is presented as a seamless and enjoyable reading experience, whether in print, in digital or on social media. I’ve spent fourteen years writing and editing features for scientific and manufacturing publications, and in making this content engaging and accessible without sacrificing its scientific integrity. There is nothing better than a magazine with great content that feels great to read.
Between studying for my English undergrad and Publishing master's degrees I was out in Shanghai, teaching, learning, and getting extremely lost. Now I'm expanding my mind down a rather different rabbit hole: the pharmaceutical industry. Outside of this job I read mountains of fiction and philosophy, and I must say, it's very hard to tell who's sharper: the literati, or the medicine makers.