Bridging the 'Omics Gap
A new personalized test aims to predict side effects
Trying to assess a patient’s potential for side effects is currently more guesswork than science; several different patients can be given the same drug but exhibit a different set of side effects because of variations at the ‘omics level.
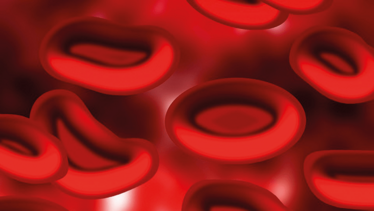
A team of scientists led by Neema Jamshidi from the Palsson’s Systems Biology Research Group at the University of California, have developed a model that could predict which set of side effects a patient will experience (1). The method combines genomics, metabolomics and proteomics to predict how variations in different people's genes impact drug metabolism. For example, the model was used to try to understand why some people experience side effects to ribavirin, a drug used to treat hepatitis C (8 to 10 percent of patients receiving ribavirin experience anemia). We spoke with Jamshidi to find out more.
How does your predictive model work?
We used a variety of individual-specific data, including plasma and red cell metabolomic measurements, single nucleotide polymorphism genotyping, supported by proteomic data (2). Since metabolomic data will not uniquely define a model for each individual, we generated nearly 4,000 functional cellular models for 24 individuals. The models simulate the metabolic behavior and capabilities of cells. We were particularly interested in red blood cells because they are biologically tractable and readily available. This means that the potential side effects we can detect must be manifested through disruption of the metabolic function of cells – anemia is a good example.
How do you expect it to be used by the pharma industry?
It could be very useful during the drug development stage because it could be used to conduct predictive screenings. I don’t think the industry currently uses cell-scale mechanistic models of metabolism so it should be very interesting. However, a realistic application of these models is probably not to expect a “perfect” model, but rather to use it as a filtering tool to identify exactly which individuals are potentially susceptible to particular side effects.
What were the most surprising moments during this project?
Initially we did not expect to identify individuals with potential susceptibility to side effects from therapeutic drugs. The goal was to determine if we could differentiate between individual people based on metabolic differences and link these to underlying genetics. There are of course many methods in machine learning that can differentiate individuals. However, the added benefit of using models is that there is biological coherence in the models and as a result we can interpret the observed behavior and predictions in the context of the metabolic network. The results of this study suggest that this methodology may be applied to other cells – and could be used to achieve individual-specific diagnoses and other precision medicine applications.
What are the next steps for this work?
There are numerous areas that we would like to pursue; the most obvious next steps are to further test the predictions made about the individuals identified in the analysis and to expand the model to include other cell types, including platelets and hepatocytes.
- A. Bordar, et al., “Personalized whole-cell kinetic models of metabolism for discovery in genomics and pharmacodynamics,” Cell Syst., 1(4), 283-292 (2015).
- A. Bordbar, N. Jamshidi and B.O. Palsson, “A proteomically derived knowledge-base of erythrocyte metabolism that can be used to simulate its physiological and patho-physiological states,” BMC Syst. Biol., (2011) doi:10.1186/1752-0509-5-110.